Rank tracking is conceptually broken and we have to have a conversation about it. My goal is to open up that conversation in hopes that we can collectively come up with a better way to do things. Below is, of course, my thinking on the subject. I welcome yours as well.
Google has continued to flood the SERPs with more features. The placement of those features is continuing to determine what can go where in the rankings. Yet, as an industry, we’re still basically counting rankings in the same manner that we always have. Our rankings model made sense for 10 blue links, but for the insights that we want from this data, it is now functionally obsolete.
I touched on this idea four years ago when I wrote the Technical SEO Renaissance; it’s worth revisiting now because the issue has become more pronounced.
For instance, if a Featured Snippet now eliminates the ability of a result to be elsewhere on page one, it is no longer position zero, it’s position one! Some tools are considering them as such. Many others are not. This inconsistency in rank tracking methodologies can be problematic for marketers as information is compared across tools.
While rankings are certainly not the measure that I recommend living and dying by, they are the lever that we directly adjust through SEO efforts. It’s in our best interest to position (no pun) rankings as a valuable part of the Organic Search feedback loop.
No matter how high-minded we attempt to be as an industry, our clients often look at rankings for us to prove we did something more than the market conditions themselves.
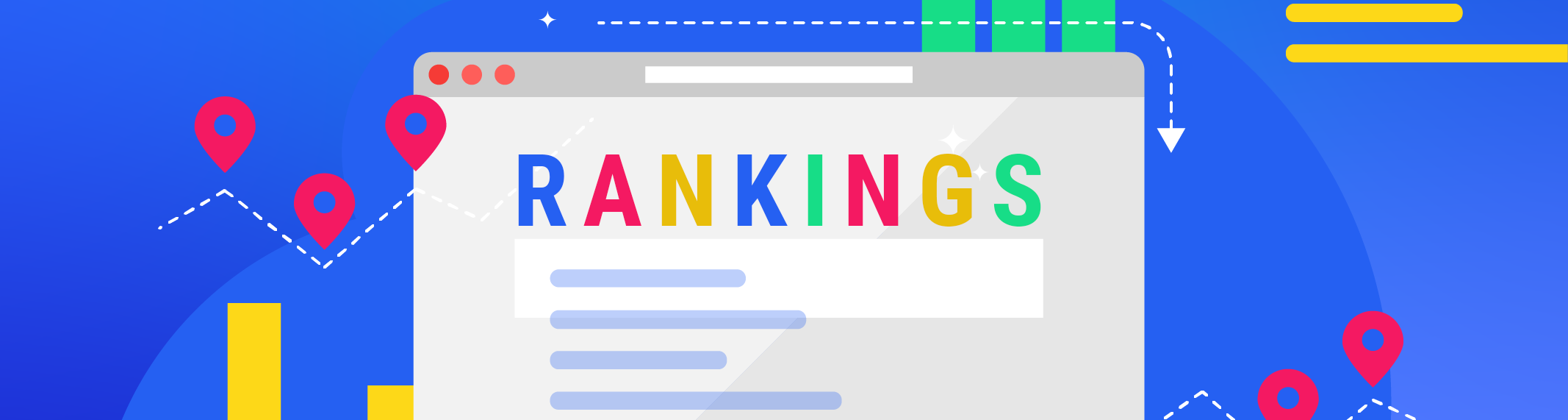
How rankings are collected doesn’t reflect an actual user context
The way the data is collected by rank tracking tools does not necessarily reflect the experience of a real user.
Over the past fourteen years, I’ve built a lot of ad hoc rank tracking tools; some more sophisticated than others. I’ve also had the privilege of seeing and/or discussing the backends of some of our industry’s most popular rank tracking tools with some incredibly bright product people. There are very smart engineers in the SEO space that have mastered the challenge of keeping up with Google’s swashbuckling hijinx in the SERPs, but I wonder if our solution, as an industry, only covers part of the problem.
For the most part, rank tracking is done as follows:
- Spin up a crawler – A stateless instance of a crawler or headless browser is instantiated behind a proxy of some kind. Effectively, this client is “clean.” In other words, it’s meant to represent a user that has never done anything on the web before so as to counteract the effects of personalization. The proxy acts as a mechanism to trick search engines because they tend to be pretty aggressive with throttling bots.
- Perform a Search – The instance performs a search for a specific query with some series of parameters set based on the ranking report’s configuration. Specifically, rank tracking tools adjust for location, device, and language. Some tools will review pages one through ten of results, while others will set the options to return one page with 100 results. Note: this distinction is important because a search engine may show different results depending on the pagination method that the tool chooses.
- Save the SERP – The SERP is retrieved from Google. Savvy teams do verification to ensure that Google isn’t faking the rank tracking tool out. It’s not uncommon for Google to return garbage data, blank pages or a captcha. The verified SERP is saved to a data store.
- Extract Features – Features are extracted from the stored SERP. What is extracted is dependent upon the level of detail provided by the rank tracking tool.
- Recycle the Crawler – The client is either killed or cycled through enough times in attempts to confuse the search engine into believing that it is a different user from a different place performing a different search.
- Repeat – The process is repeated until SERPs for all keywords have been collected.
This process represents a user that is booting their new device for the first time and having their first action on that machine be performing their first ever search on Google.
Effectively, we’re reporting on someone who has no context with Google to inform how we perform with those that do.
As a result, all rankings are the equivalent of an experiment performed in a sterile environment. Not to mention that all this scraping is potentially inflating search volume.The conclusions that come from such an experiment are often unlikely to be reflective of what may happen in the real world.
If we want actual precision and accuracy that aligns with what users see, perhaps what we really need is a series of clients with persistent user contexts for capturing rankings. Since Google does highly personalize results, if we have a persona that we are targeting, perhaps we should be performing rank tracking through a series of profiles that build the same context with Google. In that model, clients aren’t clean. Rather they are continually logged in to Google, visiting sites and clicking results to establish affinities across the ecosystem. It’s the same way you might create a Facebook profile that matches all of the features of the audience you target to verify that your ad is running.
Obviously, that doesn’t scale, but I posit that modern rank tracking isn’t about horizontal scalability; it’s about vertical focus. Nevertheless, all measurement is inherently broken,so I suspect marketers may not find that level of accuracy additionally valuable.
To that end, Google Search Console’s Position value actually makes a bit more sense than rank tracking done by SEO software because it’s giving you an average of your visibility across all the different user contexts that Google displays.
How rankings are counted is obsolete
Dr. Pete recently went into great detail about the #1 position is getting lower than ever. He highlights an example for the keyword [lollipop] where the first Organic Search result shows up at 2,938px down the page. Dr. Pete’s eye-opening research indicated that, on average, the #1 Organic (web) result is 64% lower on the page than 2013.
Naturally, that is an inherently massive problem for visibility. It’s also a large problem when using our historical rankings model as a key performance indicator. In an extreme case, for illustrative purposes, you might be #1 for a keyword since 2013 and, if you’re only using rankings to determine success, you wouldn’t understand why you’ve lost traffiRank tracking tools have done a great job of surfacing the fact that there are different features in the SERP. However, there is more to be done to contextualize that information in terms of reporting largely because those features can appear in a variety of positions.

Consider this example [car insurance]. Liberty Mutual ranks number one. As a marketer reviewing these results, you’d believe you’re doing great. The tool indicates that there are some features in the SERP and some Ads on the top, but that’s fine, right?
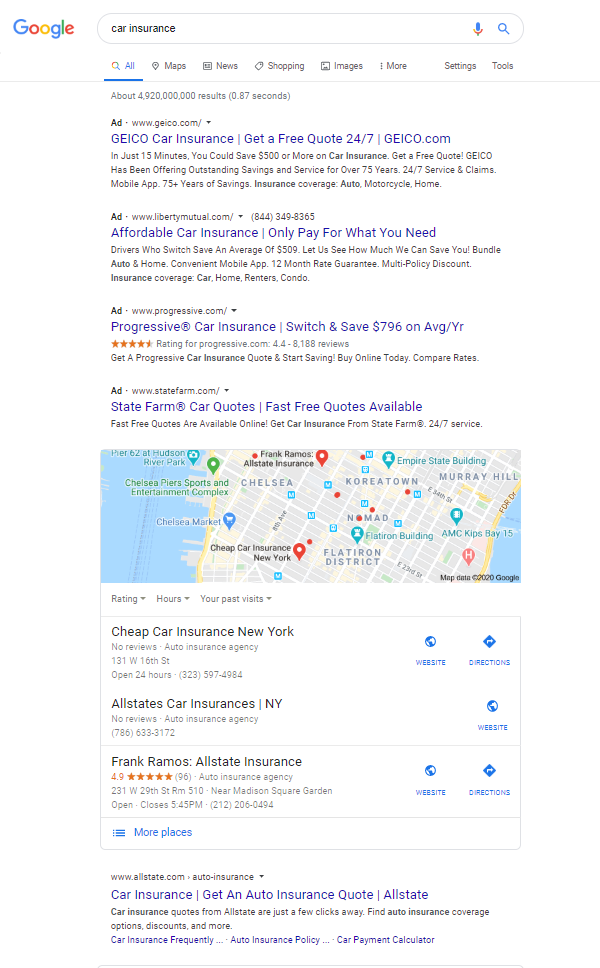
Wrong. The first Organic Search result for this query is actually the 9th option on the page. Without viewing the SERP you may not understand just how far down that is.
How about [mortgage]?
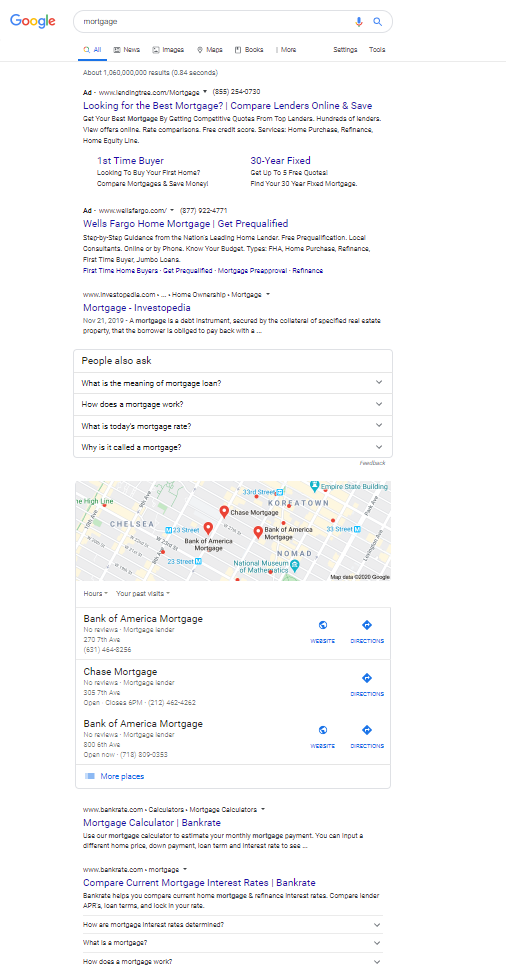
If we don’t count the sitelinks in the first ad as an option, the first organic result is the twelfth option in this SERP.
What about [nyc mortgage rates]?
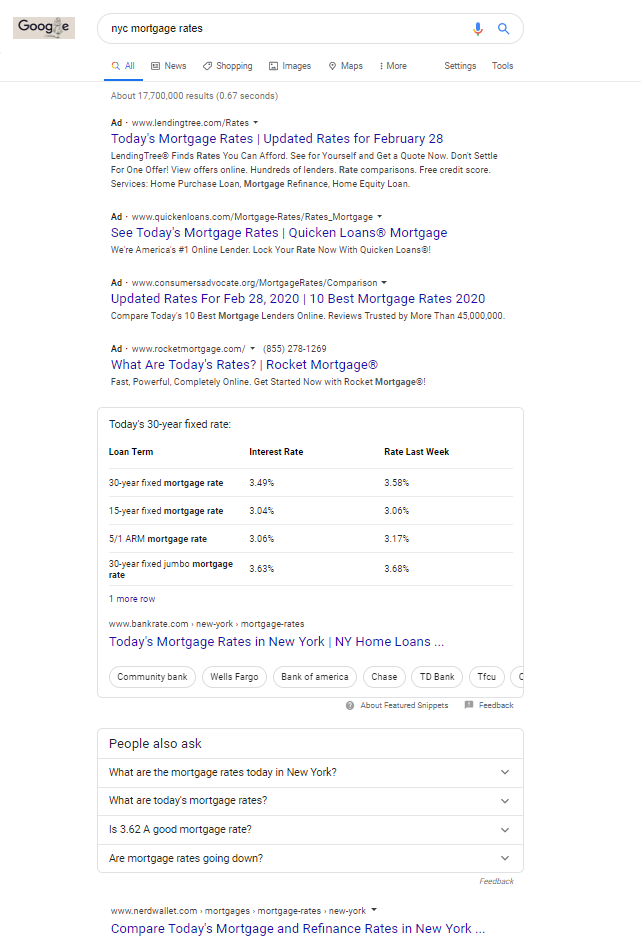
4 ads. Featured Snippet. People Also Ask. Then, finally the first organic (web) result.
This is a clear indication that Organic Search rankings need to be presented with context of the visual position, not just its numerical position in terms of other Organic Search results.
I certainly don’t have an account on every rank tracking tool out there, so some tools may have completely solved the need for context this, but here are some screenshots of some of the ones I do have access to.
One tool highlights the position and the SERP features, but there is no indication of their contextual impact upon the position of your result.
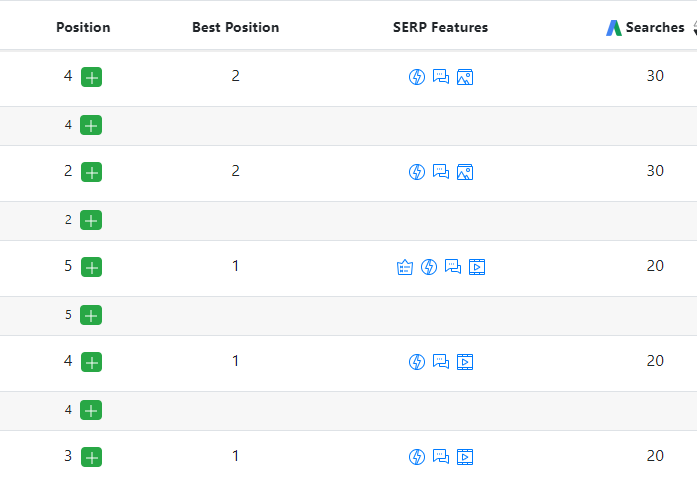
Another tool gives “Base Rank,” or a ranking without universal search features and then an indication of the SERP features. The standard ranking they provide is computed using all organic features. I believe this to be an improvement over what many tools provide.
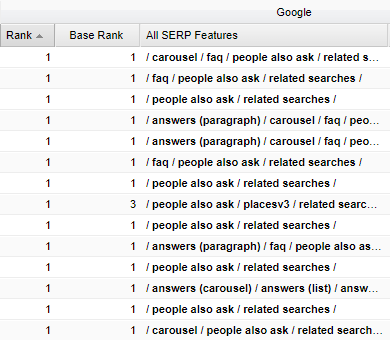
Another more “enterprise” tool uses a “blended rank” which accounts for universal, local and instant answers. I’m not aware of this measured all the same elements as the tool above.
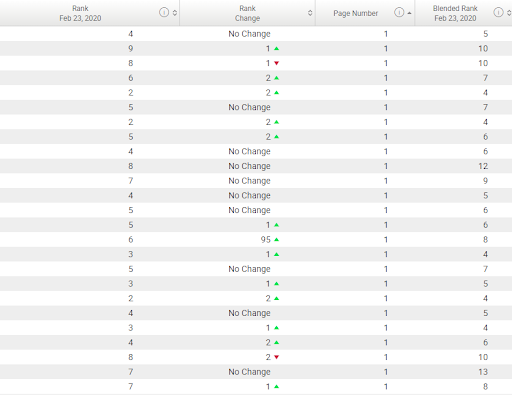
Also, with there being so many elements to choose from on the first page, this tool’s indication of which page the element appears is valuable as well. Historically, if we’re counting from 1-100, but there are actually 20 elements on page one, we may assume that element 15 is on page two. Having a clear indication of that will be incredibly valuable.
Conceptually, that raises an interesting point. We measure the first 10 blue links, then the next and then the next. Measuring 10 when there can be so many options does not reflect a user’s experience of the page.
Number One Ain’t Number One Anymore…Or Is It?
The Nielsen Norman Group recently released some compelling eye-tracking research on how people engage with modern SERPs. In the example below, their data indicates that the eye is drawn to 52 points on the page for the query [best refrigerator to buy].
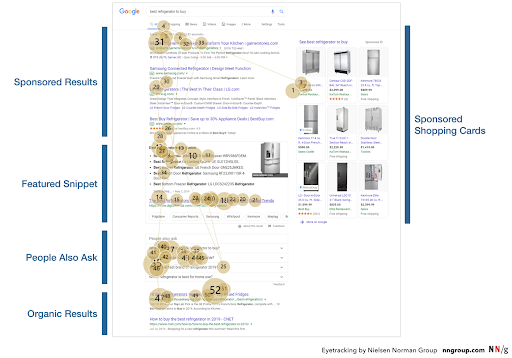
Historical eye-tracking studies from Moz, dating back to when the SERP was a bit more simplistic, suggest that the viewing pattern was a bit more vertically linear.
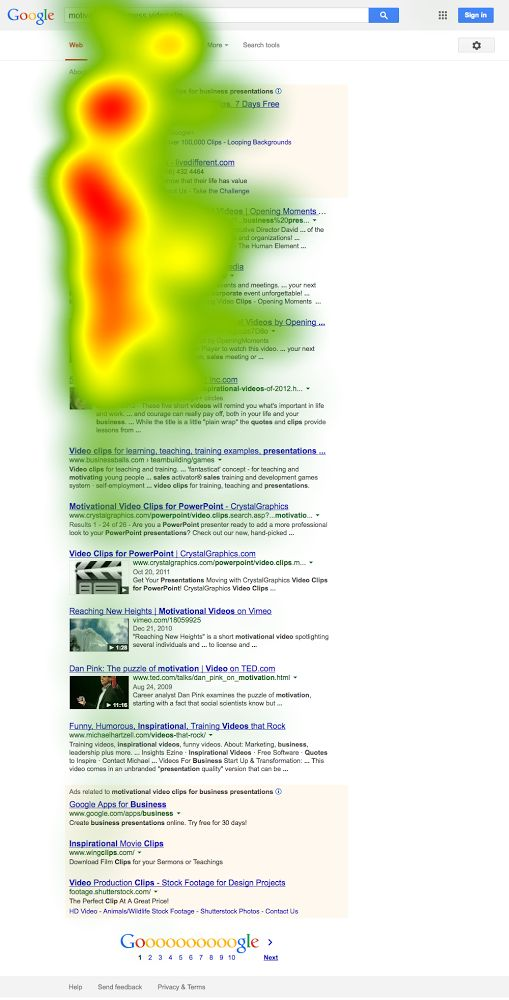
Nielsen Norman Group calls this new behavior a “pinball pattern” and it’s a reflection of how different any given SERP may be as compared to the next.
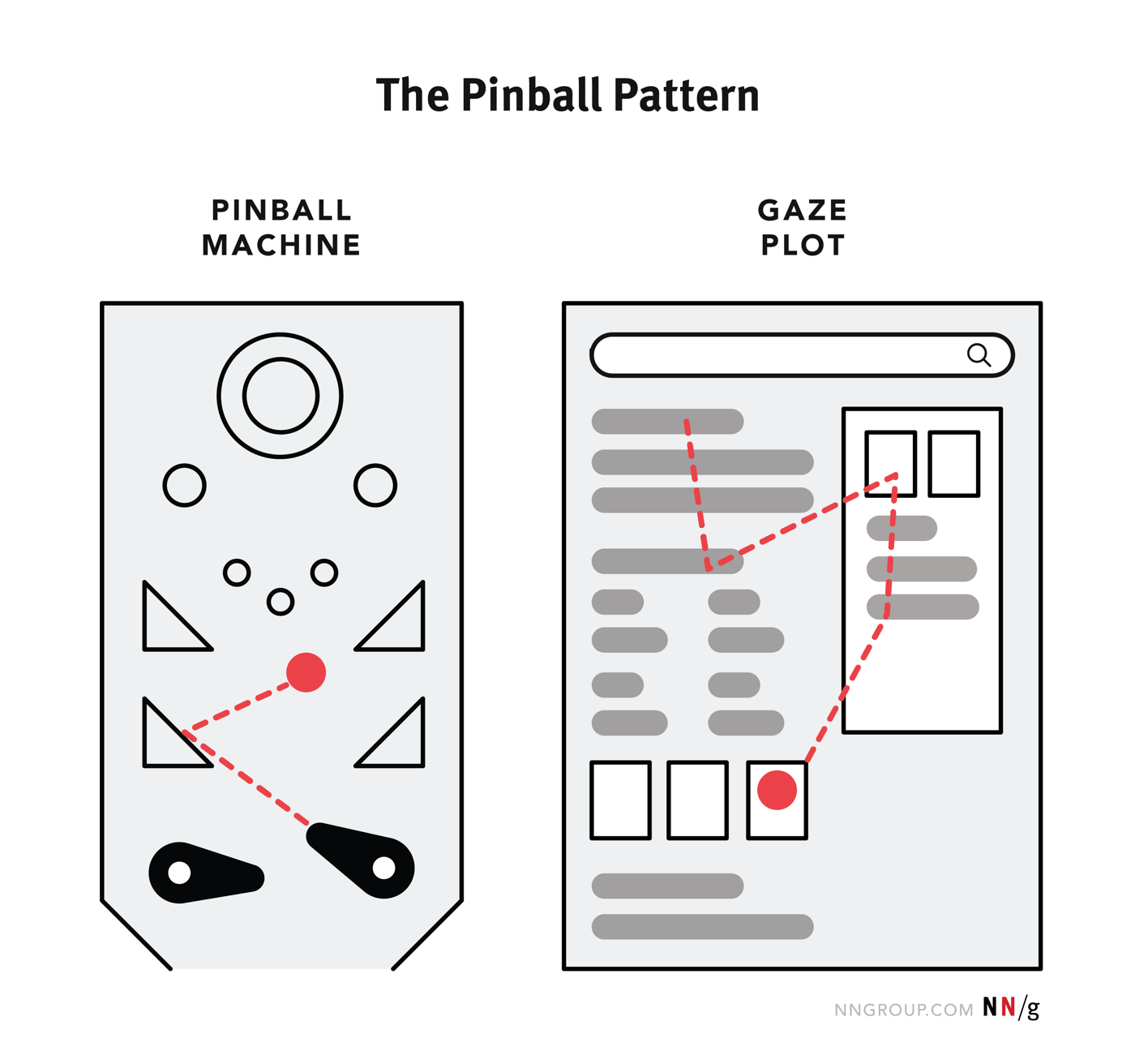
“Because search-results pages are now so inconsistent from query to query, users are often forced to assess the page before digging in and making a selection. That means that layout of a SERP can determine which links get visibility and clicks.
The inconsistency in SERP layout means that users have to work more to process it than they used to. It may be that search engines try to encourage people to explore more than just the first result. Yet, people are fairly fast in choosing a search result— we found that users spent an average of 5.7 seconds considering results before they made their first selection (with a 95% confidence interval from 4.9 to 6.5 seconds).”
Nielsen Norman Group
This is why it’s important to understand what SERP features are taking away attention from what we know as organic rankings. It’s another reason why how we measure rankings doesn’t necessarily align with the current patterns of user behavior. People skip around and make decisions based on elements that we don’t have a good way to measure click through behavior on.
Separately, this is also why models that attempt to reverse engineer (not provided) don’t make much sense either. In examples I’ve seen, the cross-validation data set used is from pre-2011 results. It would be erroneous to use Google Search Console’s Search Analytics data as the cross-validation data set, because its positions do not directly reflect our rankings model. This makes the conclusions inherently invalid because the presence of new SERP features dramatically changes the user behavior.
Well, what does Google think?
Google, or at least Danny Sullivan, agrees that the ten blue link focused rankings is a bit outmoded. He expresses as much in response to Dr. Pete’s post.
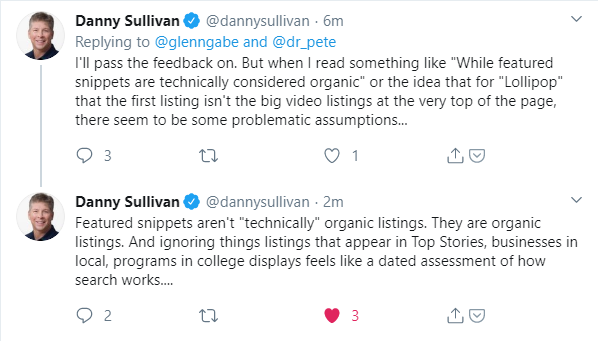
Again, Google’s model for Position actually makes a lot more sense than what we’re doing because it takes into account all Organic visual elements rather than just the ten blue links. From the documentation on how Position is measured:
“Most of search result elements have a numeric position value describing their position on the page.The following desktop diagram shows a search results page with four blue link sections (1, 3, 4, 5), an AMP carousel (2), and a Knowledge Panel card (6). Position for each is calculated from top to bottom on the primary side of the page, then top to bottom on the secondary side of the page (in right-to-left languages, the right side is the primary side), as shown here:
Google
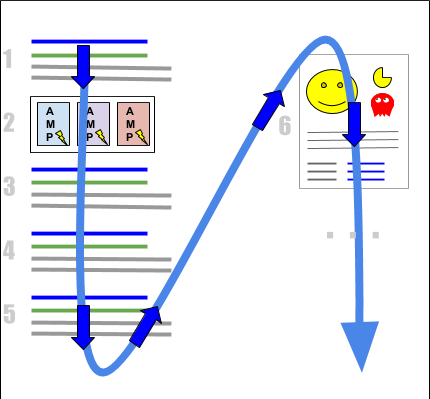
The location of the result elements on the page can vary depending on the device type, search features, and the screen size, but the general rule is the same: position is calculated top to bottom, left to right (or right to left for RTL languages). Note that this method of calculation might change in the future.
All links within a single element have the same position. For example, in the previous diagram, all items in the AMP carousel have position 2; all links in the “blue link” block at position 5 have position 5; all links in the knowledge card have position 6, and so on.
Google
When considering a SERP such as [life insurance], we have four ads, an organic result for Nerdwallet that is visually lost between the ads and People also ask rankings. All of that is next to the visually overpowering Knowledge box on the right.
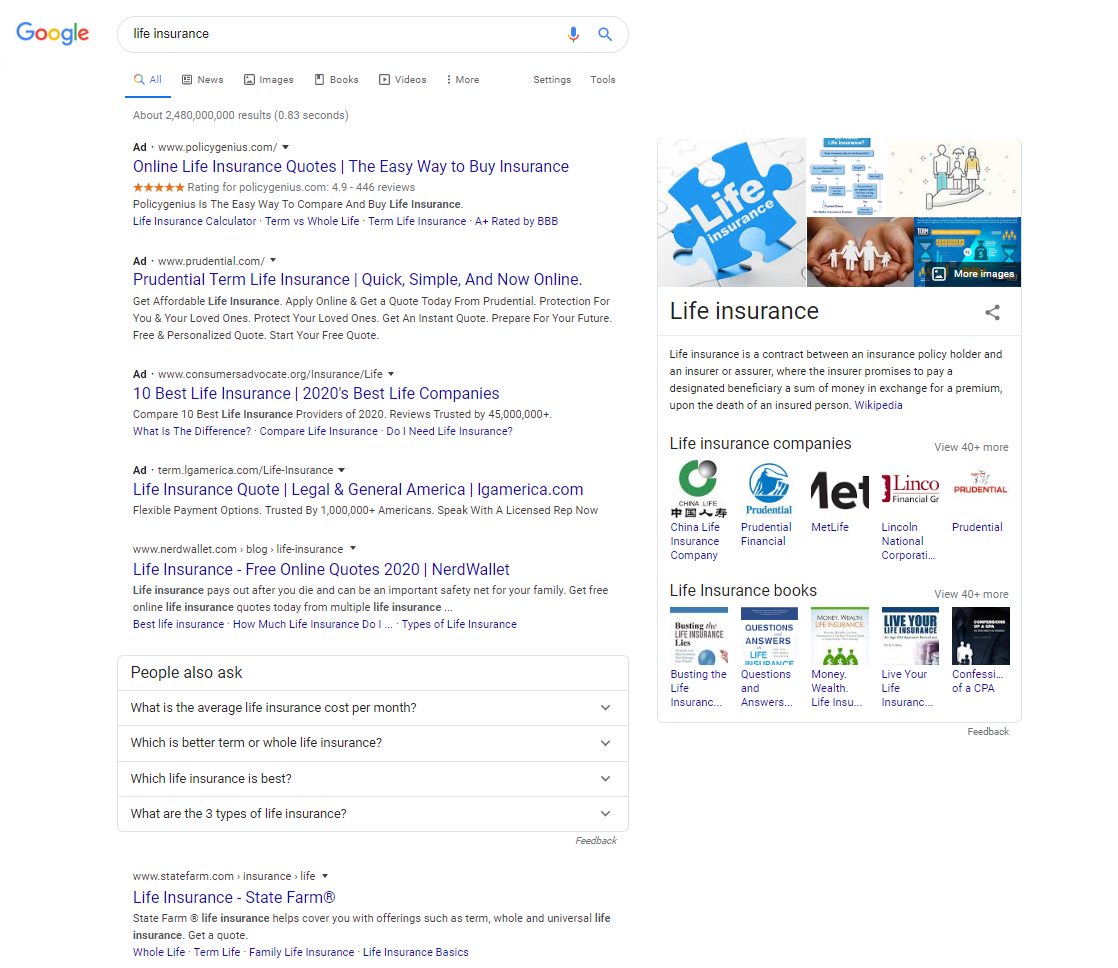
Following Google’s Position methodology, it would make more sense to consider the Nerdwallet result number 1, the People Also Ask number 2, the State Farm result number 3 and the Knowledge box number 4. These are the things the user would actually see organically.
However, I don’t think Google’s approach with Position is perfect for the SEO’s use case, but I think it is more reflective of the reality of the SERP and how users interact with it.
How does this all impact click-through rate?
With data sources like Jumpshot and Hitwise meeting their untimely demise, we no longer have visibility into the clickstream activity on Google.
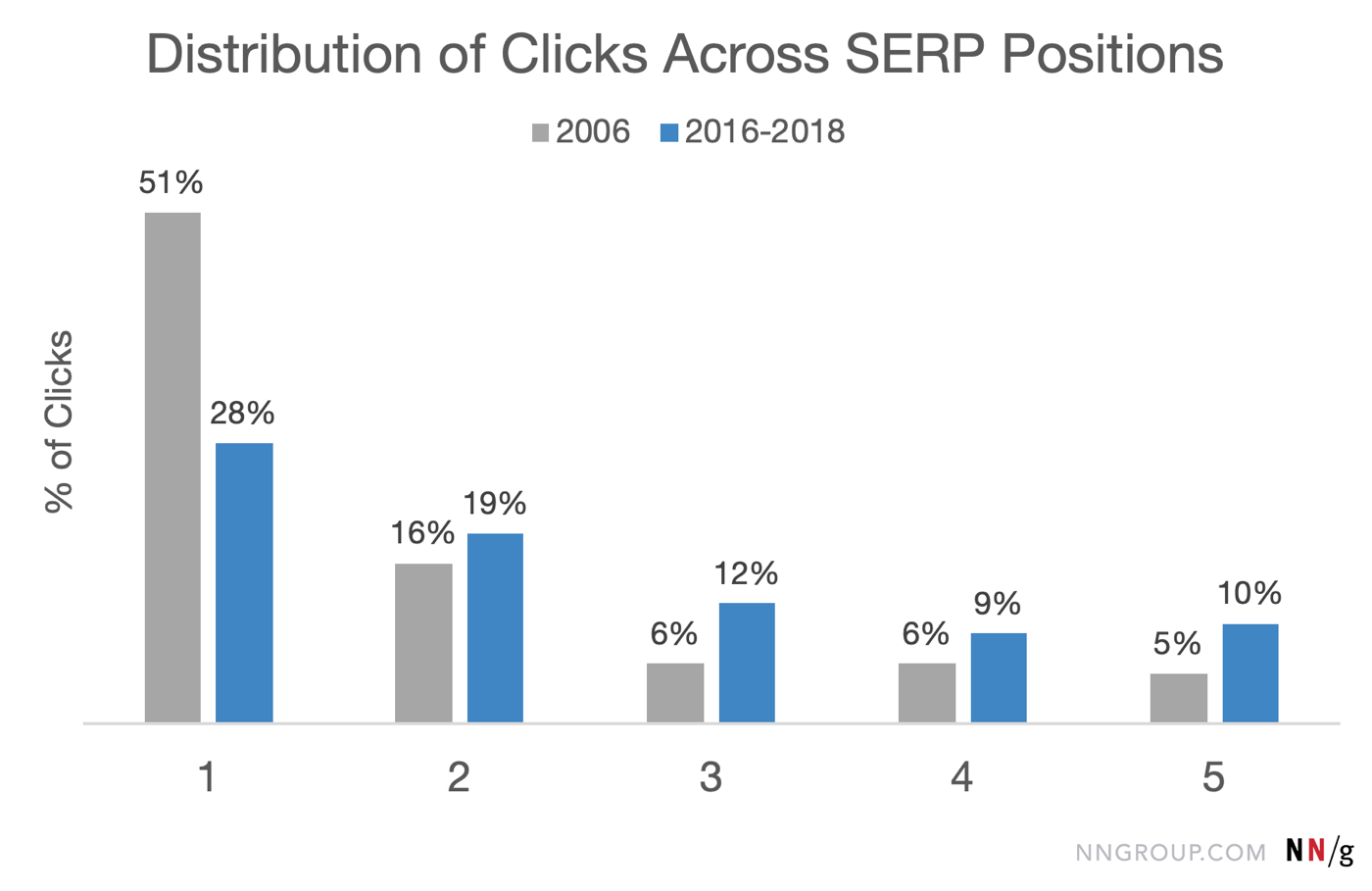
However, NNG also took a look at the distribution of clicks and how they’ve changed over time. What they found is what we’ve all been seeing as far as there being a redistribution of clicks across the top three. Historically, we know that the number one position in the ten blue links dramatically outperformed anything else.
While their examination was based on users in their eye-tracking study, the click through rate models we use in SEO are blind to what else is happening on the SERP. That is to say, what we may have thought of as 0-click may have been a click on a Featured Snippet or a link in a People Also Ask.
Since the Position model doesn’t align with our rankings model in that it counts other features in determining position, we effectively don’t have a dataset to compare against. In other words, we don’t truly know how click-through rate is impacted and we won’t until we bring some alignment between Google’s model and SEO’s model.
A Proposal for Rank Tracking v2.0
Naturally, the next question is what do we do next? How do we, as SEOs, move forward?
Well, a metric of any kind is not valuable without context. As such I’m proposing that we open up ranking from (up to) two values to six:
- Legacy Rankings – All platforms should continue to compute rankings the way they previously have to allow for reporting continuity. Introducing something different from the way that rankings are currently considered is difficult because making the switch will potentially tank all of your reporting. Legacy ranking models should still be computed and added to reports. The new rankings model could also be applied for those tools that maintain SERP archives to give marketers a good sense of what these metrics illustrate over time.
- Web Rankings – This is the metric that we’ve been using. However, we’d count the presence of a featured snippet as part of this metric. This would be the equivalent of STAT’s base rankings
- Absolute Rankings – A position complete with all features on the SERP including ads.
- Feature Rankings – Organic Rankings that include all Organic SERP features. Presumably, this could be used to better compare GSC data with a ranking.
- Offset – The pixel position of the ranking from the top of the page. This would be measured by the distance that the a tag has from the body tag.
- Page – The page of the SERP that the ranking was seen on.
Counting would follow the same top left to bottom right model that Google has illustrated. Page two and beyond will follow the same model.
This model for rankings brings more context, standardization across tools and makes ranking values far more actionable without the need to visually review every SERP.
Proof of Concept
To show how this could potentially work, I’m going to use SerpApi. They do a great job of abstracting the rank tracking process and extracting all the features that you need into JSON. You won’t have to worry about all the work that goes into managing proxies, DOM traversal and Google’s various attempts to thwart your scraping.
For this, we can get everything we need for the proof of concept from the demo on the homepage.
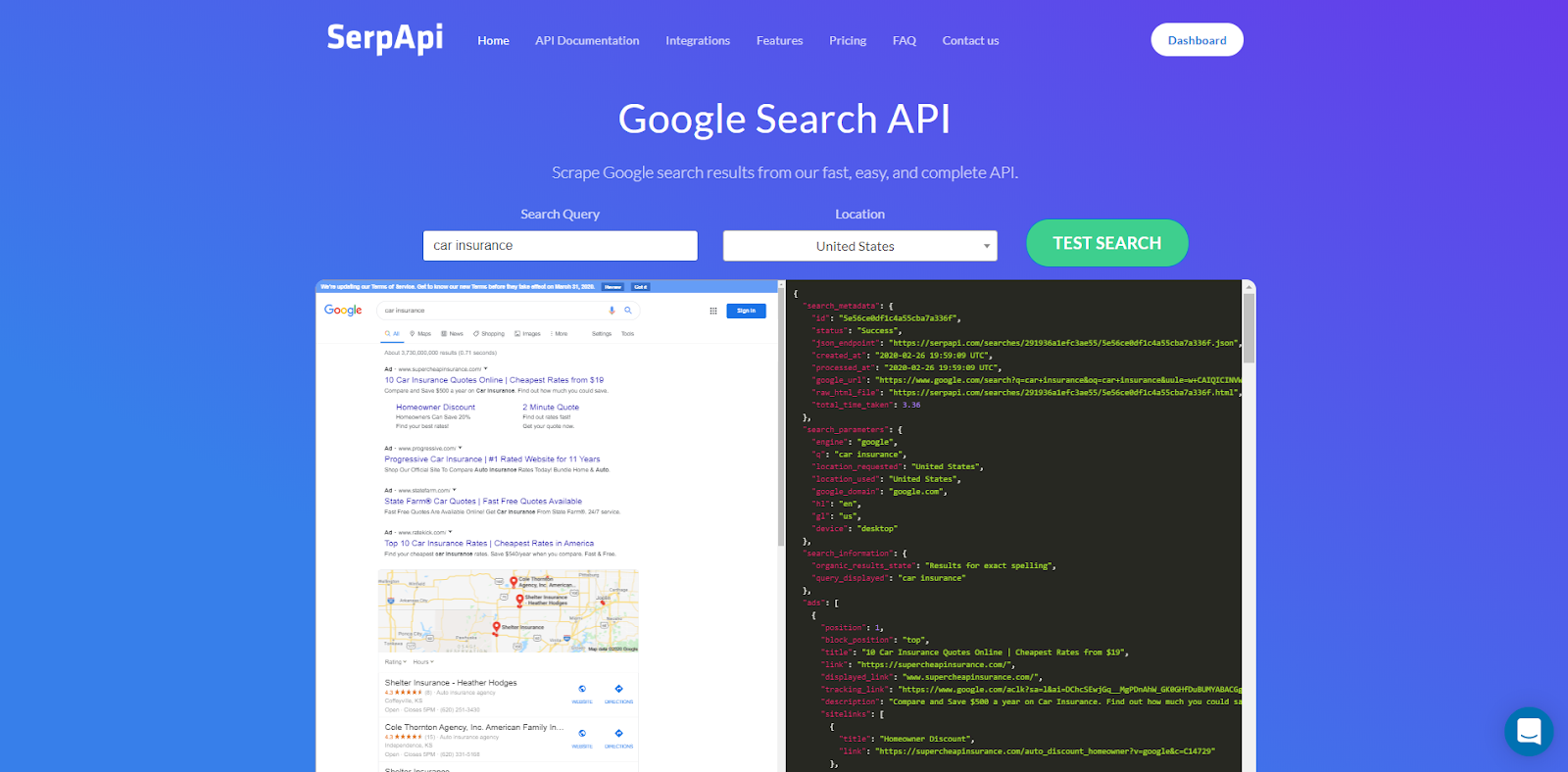
Effectively, for any given query, you can use their HTML source to compute your visual offset and the JSON response to compute your own rankings counts.
In this case I’ve collected the JSON for both the [car insurance], [mortgage] and [nyc mortgage rates] queries. Using the JSON object, you can also pull the HTML source from the search_metadata.raw_html_file member.
I’ve written some NodeJS code (with some support through callback hell from John Murch) to illustrate how this can be done. You can find it here and play with it at your leisure. Pull Requests are open.
In the example below, we are imagining that we are NerdWallet looking to identify where we rank under this new model. We loop through the JSON object and count positions of element types that are relevant to the true visual user experience.
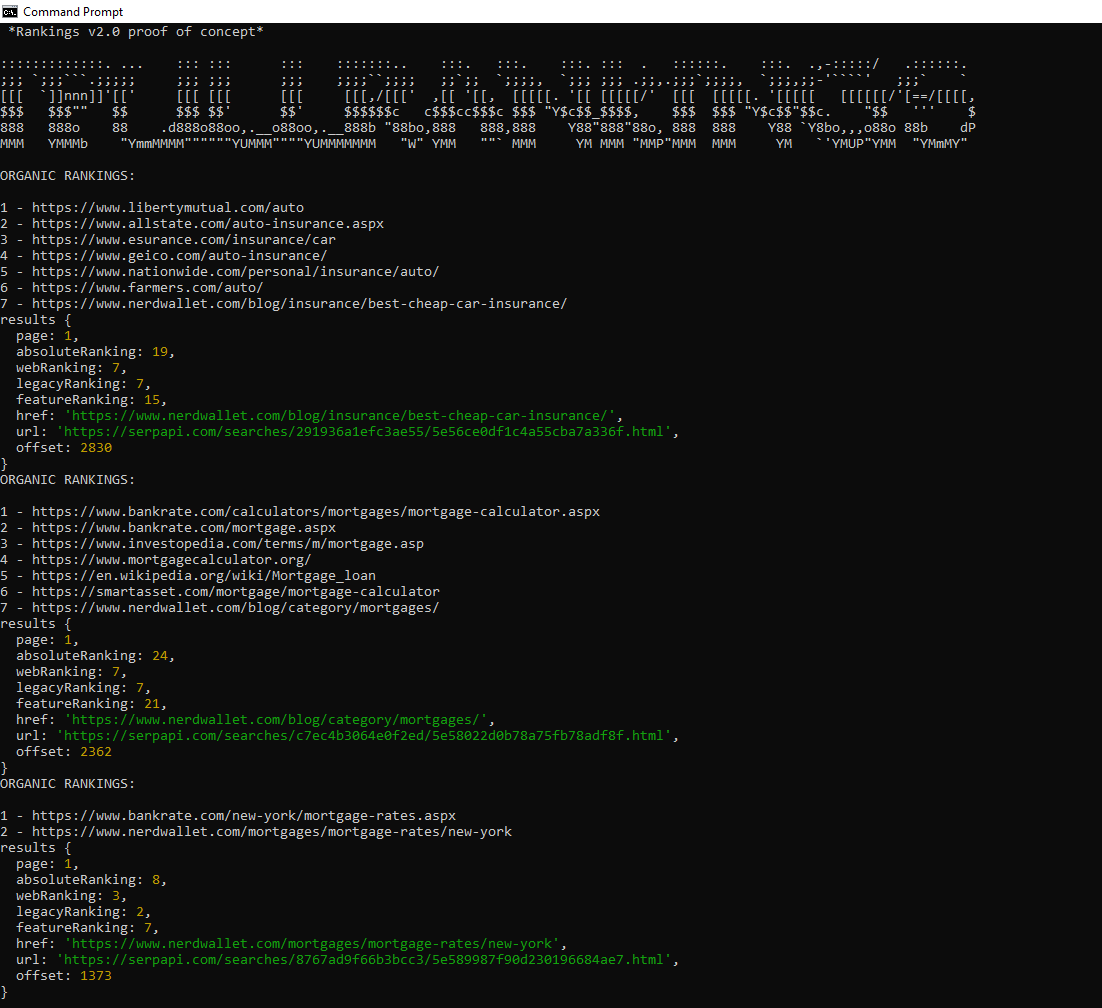
For the keyword [car insurance] that yields:
- Web Ranking – 7
- Legacy Ranking – 7
- Absolute Rankings – 19
- Feature Rankings – 15
- Offset – 2830px
- Page – 1
For the keyword [mortgage], the code would return:
- Web Ranking – 7
- Legacy Ranking – 7
- Absolute Rankings – 24
- Feature Rankings – 21
- Offset – 2362px
- Page – 1
For the keyword [nyc mortgage rates], the code would return:
- Web Ranking – 3
- Legacy Ranking – 2
- Absolute Rankings – 8
- Feature Rankings – 7
- Offset – 1373px
- Page – 1
For [nyc mortgage rates], notice that the Base Ranking and Organic Ranking are different due to the presence of the Featured Snippet in this SERP.
Also, without the need to look at the SERP, you now can now clearly see that there is less competition for attention for a keyword such as [nyc mortgage rates] than the other two.
Again, my goal here is to start a conversation about improving measurement of Organic Search. This is a presented as a proof of concept that indicates the approach can be done. Much of the heavy lifting is abstracted by SerpApi and the code is not sophisticated. It’s simply just a mechanism to explain that what I’m suggesting is possible.
The SERP is New, Our Rankings Should be Too
Search Engine Optimization is certainly not a field where we can do things the way we have always done them — simply because we have always done them that way. Search engines will continue to dramatically change in response to the needs and behaviors of users. Our tools and our metrics need to keep pace and in some cases, help drive that innovation.
Rank Tracking 2.0 will offer us the opportunity to evolve with Google and to deliver more actionable reporting. The same way that you see a keyword is dominated by Amazon and Wikipedia you know not to waste your time, you’ll know if #1 is 2000px down, it’s not worth your time.
Now over to you. How have current ranking methodologies not been enough for your use case? How have you overcome them?
Leave a Comment