The next chapter of search is all about engineering the type of relevant content that can easily show up in every type of search. Businesses need to drive visibility in all channels, including Generative AI systems, Tik Tok, Amazon, and even app stores.
All these various channels have different nuances to take into account, but they have one thing in common: relevance ties it all together.
Engineering relevance treats visibility in modern search and AI systems as an engineering problem, not an optimization exercise. The idea of engineering rather than optimization fits because more often than not you have to build something rather than tweak something. Whether it’s software, content, or relationships across the internet, it’s all a form of engineering.
Nearly all organic discovery systems, from AI Overviews (AIOs) to YouTube’s algorithm, still rely heavily on behavioral signals like watch time, dwell time, and abandonment rates. But what gets shown has shifted. Search engines now fan out queries semantically, tapping into topical clusters. Yet the SEO industry continues to force-fit strategies into outdated frameworks.
Almost all search now uses Generative AI, so this engineering process will work everywhere. For example, when it comes to YouTube, optimizing images in videos or what’s said in the video still heavily relies on user interaction (watch time, abandonment rates, etc.). This is still how organic search works as well, but standard SEO work isn’t enough. SEO keeps trying to shove everything into a framework that doesn’t really work anymore and is not what search engines are currently using.
Standard search looks for certain keywords on a page to decide its rank on a SERP, leading to some irrelevant content getting solid engagement and performing well. AI looks for particular passages and phrases from relevant sources. And it goes deep into a site to find them, too, with 82% of Google AI Overviews citations coming from deep pages.
What does this mean? We should no longer simply optimize content. We need to engineer it.
What is Relevance Engineering?
Relevance engineering is the art and science of improving visibility for any search surface. It’s the intersection of information retrieval, user experience, artificial intelligence, content strategy, and digital PR to give visibility in any form of search
In other words, it’s the culmination of all your hard work over the years to build your company’s brand, create helpful content for customers and prospects, and design a site that’s straightforward, accessible, and keeps searchers coming back for more.
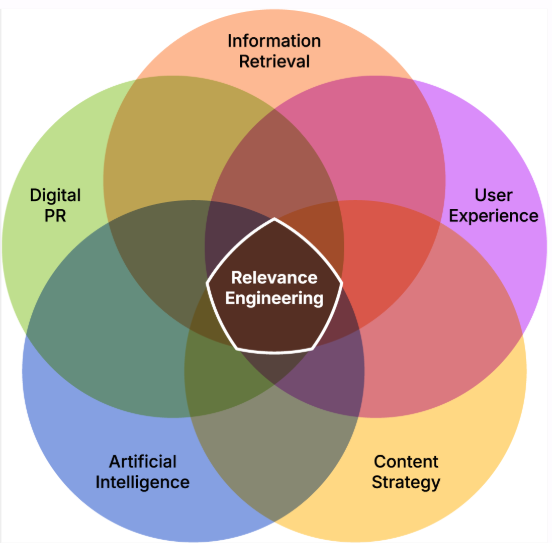
You’ve been doing that all along, right?
With the advent of Generative AI search systems (AI Overviews, Perplexity, Gemini, etc.), it’s clear that the future of search is retrieving relevant content that’s wrapped up in a neat, no-click package and served directly to the searcher (essentially featured snippets on steroids).
What impacts will this have on the day-to-day work and life of SEOs? The typical thinking needs to change. Factors like using keywords in the page title and H1 and constantly building more links don’t matter in a Gen AI environment. You need a more technical understanding to verify that what you’re doing will actually move the needle.
Relevance engineering turns relevance into something quantitative and measurable. SEO is often about vagaries, or someone’s best guess of how Google works, while this is a more science-driven method of approaching search. But relevance isn’t subjective, so we need relevance scores in our tools.
Conversational search is largely about relevance and can be scored mathematically. Like traditional search, documents and queries are plotted in multidimensional vector space. The closer a document vector is to a query vector, the more relevant it is.
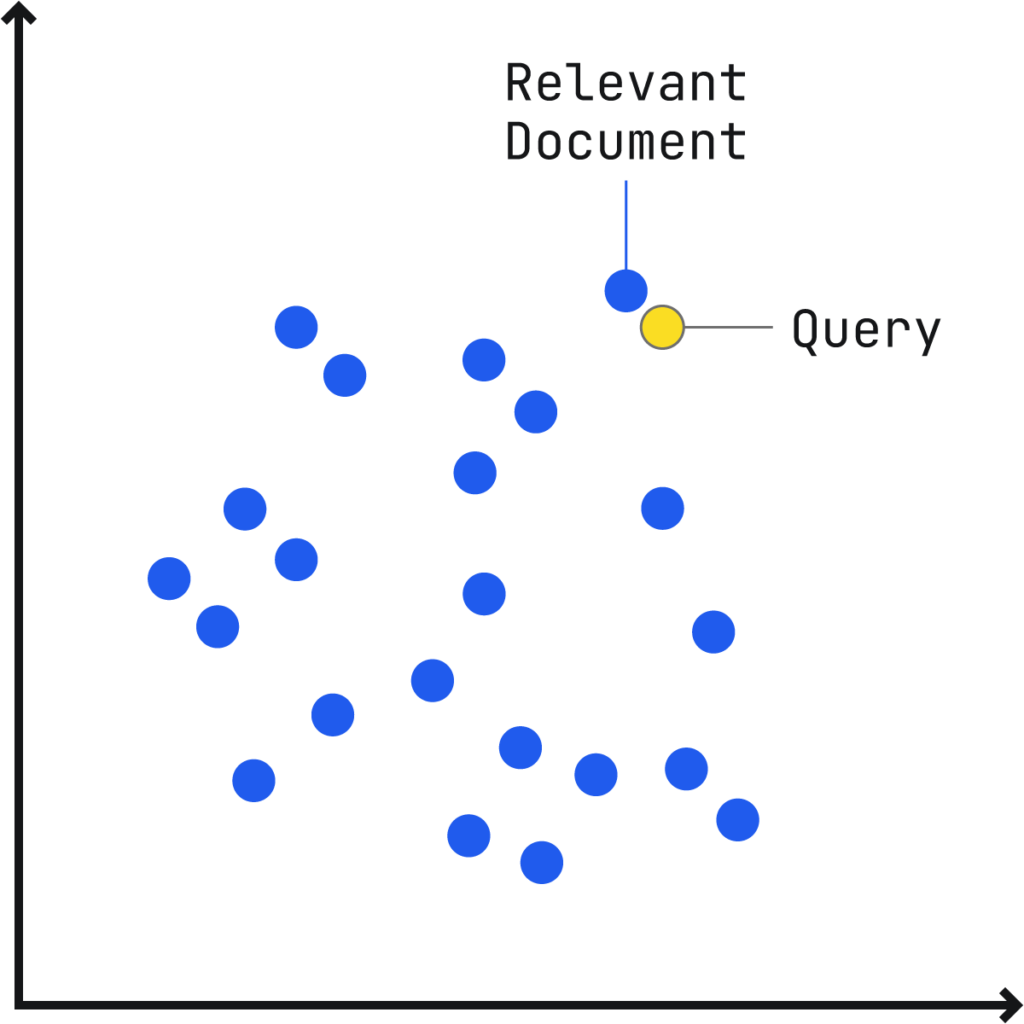
When HubSpot coined the phrase “inbound marketing” back in 2005, they created a framework for why channels often considered to work in isolation should always work together. Similarly, we are framing a new practice and requiring people to level up across a variety of disciplines.
SEO professionals are familiar with best practices and are effectively just power users of search engines. But as it stands, SEO is poorly defined. Everything new that Google says is suddenly under its purview, so relevance engineering is a new framework with elements of all disciplines.
Relevance engineering builds quality into its core methodology, treating content as part of a scientific system rather than a marketing tactic. What many call “content strategy” in SEO circles often amounts to elaborate plans that frequently remain theoretical rather than operational.
Relevance engineering, by contrast, incorporates workflows where everything serves a clear purpose. It brings engineering discipline and scientific rigor to what has often been an improvised process:
- Information is structured to make intuitive sense to users.
- Content decisions are made based on measurable objectives.
- Results are analyzed systematically, not anecdotally.
- Success patterns can be replicated and scaled.
Relevance Engineering in the Age of EEAT
Relevance engineering provides a structured, AI-driven approach to ensuring content visibility. As mentioned before, this methodology blends user experience, AI, content strategy, digital PR, SEO, and sometimes audience research to align content with both user expectations and search engine evaluation criteria.
While audience research isn’t always necessary for every optimization effort, it’s a crucial component that many overlook in SEO. Understanding user intent remains fundamental to achieving relevance.
With Google’s focus on experience, expertise, authoritativeness, and trustworthiness (EEAT), relevance engineering moves beyond traditional keyword matching. Google can measure these factors at the author, site, and entity levels using vector embeddings, evaluating how closely a page aligns with a user’s expectations.
For example, a site focused on sneakers that suddenly starts publishing content about bananas would likely dilute its site focus score, signaling irrelevance. Similarly, an over-reliance on AI-generated content without strategic alignment can trigger negative detection signals.
EEAT is not about author bios and it never was. Google is looking to model authors around the web, creating vector representations of them and then comparing them to other authors. Site level vectors will vectorize the pages and then average these vectors to represent the entire site. It’s the same with authors; Google looks for all the content you write and creates an average vector to represent your work.
Don’t get me wrong, author pages aren’t bad. They’re helpful if you need to disambiguate yourself from other people. But the real value comes from writing a lot of content about a specific topic. If you write 100 pages of content and 60 of them are about SEO, you can be mathematically labeled as an expert on SEO.
EEAT is abstract and represents everything Google is doing, but it’s this oversimplification that causes the understanding to get lost in SEO. Over the years, it started with webmasters and web developers that knew and understood the underlying tech, but then overspecialized content SEOs, technical SEOs, link builders, etc. came in who didn’t know enough of the big picture about how things work. This doesn’t happen with relevance engineering.
Relevance engineering leverages AI-driven analysis to predict whether content strategies will succeed before they are deployed. This is akin to a publicly available, practical application of what Google might use internally to assess relevance.
You wouldn’t publish an article that scores poorly in cosine similarity to the target topic or pursue backlinks from a site that lacks topical alignment. A data-backed approach minimizes the guesswork that has traditionally defined SEO, allowing marketers to optimize with precision rather than the usual trial and error.
Is Google Search Getting Worse?
German researchers did a year-long longitudinal study of Google Search that showed SEO content that’s primarily driven by affiliate marketing has made search worse.
SEO-driven affiliate content prioritizes monetization over user value. The study found that much of today’s search results are dominated by commercially optimized content designed more for rankings than for genuine usefulness. Google needs to distinguish between high-quality, relevant content and pages engineered purely to capture traffic.
While Google has stated that AI-generated content is acceptable as long as it’s created for people, the challenge lies in differentiating good from bad content at scale. Traditional ranking signals still play a role, but user engagement metrics, like whether a user’s search session is successful, are becoming more important indicators of quality.
Despite Google’s efforts to detect AI-generated content, detection remains unreliable. OpenAI abandoned its AI text detector due to its mere 25% accuracy rate, and similar tools frequently produce false positives and false negatives.
Because of this, teachers and professors are running student assignments through these AI detectors and they’re being incorrectly flagged as machine-generated. AI-generated content detection continues to be a problem, and as models evolve, previous detection mechanisms quickly become obsolete.
To address these challenges, Google must continuously adapt its evaluation methods, leveraging more robust, multi-layered signals rather than relying solely on direct AI detection. Search quality depends on filtering out low-value content and accurately assessing what resonates with users.
Google has updated its Search Quality Rater Guidelines to expand its definition of spam, address filler content, and target misleading or exaggerated claims to help identify low-value content online.
The Importance of Structured Data
As search engines become more reliant on AI and machine learning, the role of structured data remains critical. Structured data helps search engines understand, categorize, and connect information, making content more accessible and relevant to users. By organizing data in a machine-readable format, businesses can improve their visibility in search results, voice search, and AI-driven experiences.
Recent advancements show that structured data is evolving alongside AI, particularly through three emerging models that integrate knowledge graphs (KGs) with large language models (LLMs):
- Knowledge Graph-enhanced LLMs – These models use structured knowledge from KGs during pre-training and inference, ensuring that LLMs generate more factually grounded responses.
- LLM-augmented Knowledge Graphs – In this approach, LLMs enhance and complete KG data, filling in missing connections or inferring relationships that might not be explicitly stated.
- Synergized LLMs and Knowledge Graphs – This multilayered system combines the strengths of both approaches, allowing LLMs and KGs to work together dynamically, improving both knowledge retrieval and reasoning.
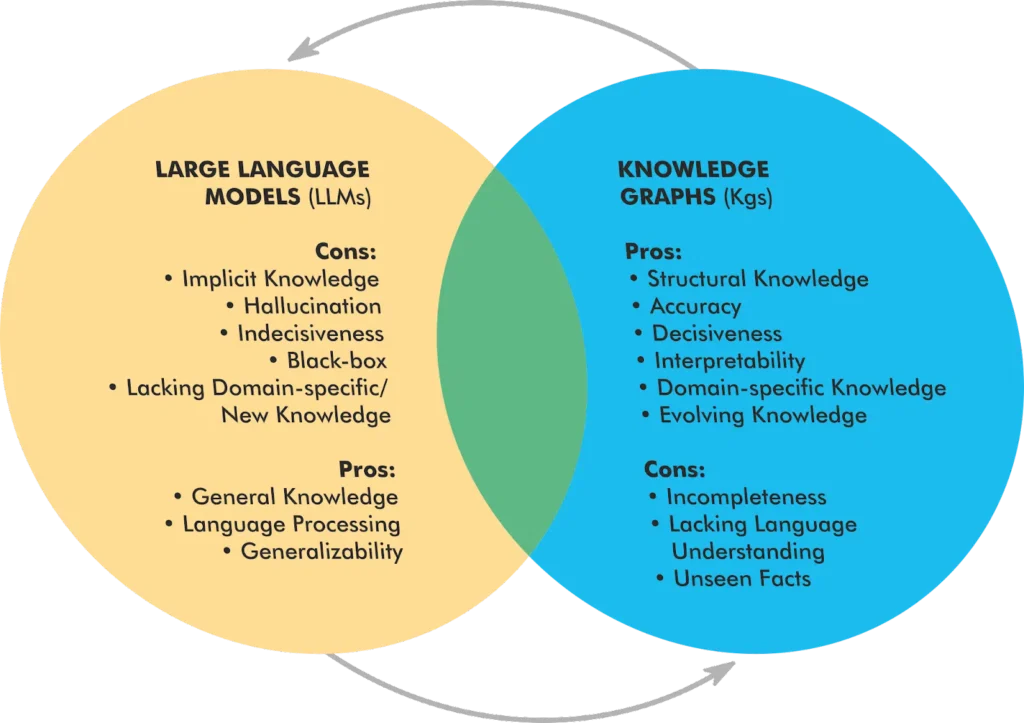
By embracing structured data, businesses not only enhance their search visibility but also future-proof their content for AI-driven search. As Google’s search algorithms continue to integrate LLMs, structured data will play an even greater role in ensuring that content is accurately interpreted and contextually relevant.
Engineering for Relevance
There are a number of other ways to ensure your brand’s content is more visible and is working with AI rather than against it.
Google shifted from lexical to semantic search a decade ago with its Hummingbird update and most SEO software hasn’t caught up since. The lexical model counts the presence and distribution of words, while the semantic model captures meaning. This was introduced through an innovation called Word2Vec.
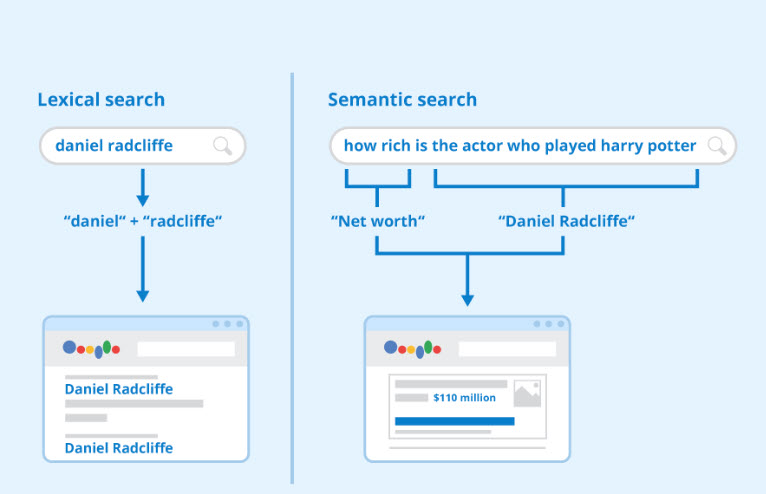
The Importance of Fraggles
When it comes to showing up in AI Overviews, it’s all about fraggles, a term that describes the fragmented passages that populate AI Overviews like in the example below:
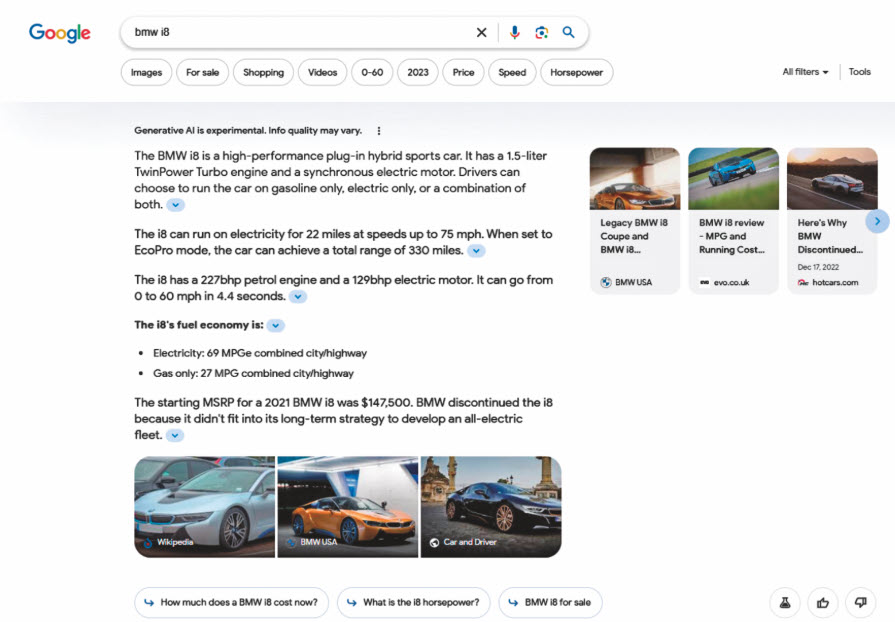
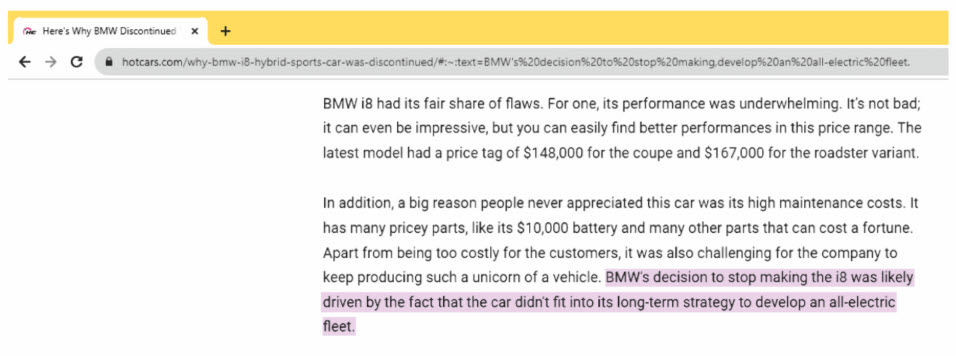
If the content on your site is arranged in easy-to-find simple phrases that answer specific questions, you have a better chance of having fraggles show up in AI Overviews.
Semantic Suggestions for Content Relevance
Here are some tips on how to structure your content for a better chance of appearing in AI search results:
- Clearly Structure Your Content into Semantic Units
Break down your content into concise paragraphs or sections that cover clearly defined topics. Long paragraphs blend multiple ideas, making embeddings less specific. Shorter paragraphs (50 to 150 words) typically capture distinct semantic concepts or ideas more cleanly, resulting in clearer vector embeddings. Also, use headings and subheadings to separate sections.
- Use Explicit Semantic Triples or Clear Subject-Predicate-Object Patterns
Embedding models capture semantic relationships best when content explicitly outlines relationships. Semantic triples (subject-predicate-object) significantly boost retrieval accuracy and content relevance.
- Incorporate Rich Contextual Keywords and Entities
Explicitly mention closely related keywords, synonyms, or entities to enhance semantic understanding. This increases the chance of being retrieved and accurately cited.
- Provide Unique, Highly Specific, or Exclusive Insights
Unique content or proprietary data increases the likelihood that your page is retrieved and cited as authoritative in RAG pipelines.
- Avoid Ambiguity
Clearly defined, straightforward sentences reduce embedding noise and retrieval errors.
The Future of SEO is Relevance Engineering
As search evolves, relevance engineering has become essential for ensuring content aligns with both user intent and search engine expectations. The growth of EEAT, AI-driven content, and structured data underscores the need for a scientific, data-backed approach to SEO that goes beyond guesswork to prioritize measurable relevance.
While concerns about declining search quality persist, driven by SEO-first affiliate content, incorrect information (AI hallucinations), and unreliable AI detection, Google will continue to refine its ranking mechanisms, relying more on user engagement signals and structured knowledge to assess content value.
How we operate as an agency has also changed as a result. Scoring is already part of our regular content audits for clients, but we understand the need to bring more to the surface of our work. We now provide relevance engineering insights, or Relevance Audits, to help clients work with AI and remain prominent in search results. Sound like something you need? Reach out and let’s talk.
- An Introduction to Relevance Engineering: The Future of Search - May 8, 2025
- How Retrieval-Augmented Generation is Redefining SEO - March 27, 2025