I attended the first day of Google I/O 2025 and left feeling a mix of excitement and anxiety. On one hand, as a user and developer, I’m excited for the new products and features. Google is truly a marvel of modern technology and that was on full display with products like Flow, AndroidXR, and Search. On the other hand, I’m terrified at what it means for the SEO community because the skillset and technology we use to support driving visibility is not prepared for where things are headed. To top it off, the ongoing conversation is keeping people complacent which is dangerous for the advancement of the field.
There’s been much chatter within the SEO community lately about how the generative AI driven features of Google make no difference; “it’s just SEO.” In fact, Google’s latest attempts at guidance reflect that. Much of the argument is rooted in the overlapping mechanics between generative information retrieval and classic information retrieval for the web.
Yes, you still need to make content accessible, indexable, and understood, but the difference is that in classic IR, your content comes out the same way it goes in. In generative IR, your content is manipulated and you don’t know how or if it will appear on the other side even if you did all your SEO best practices right and it informed the response. Therein lies the disconnect and the layer where SEO as it currently exists is not enough.
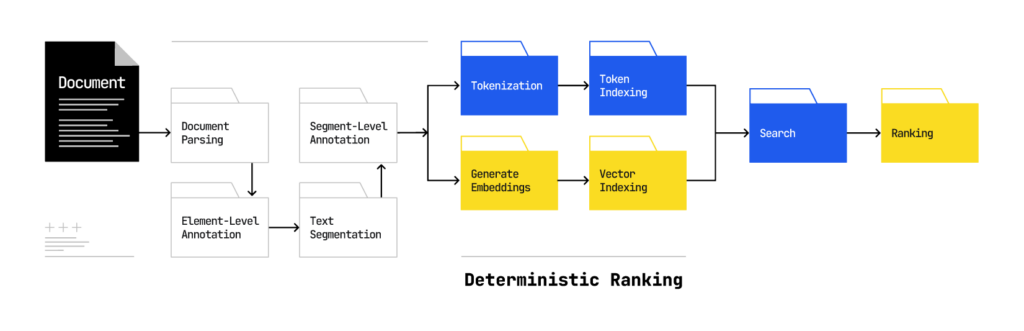
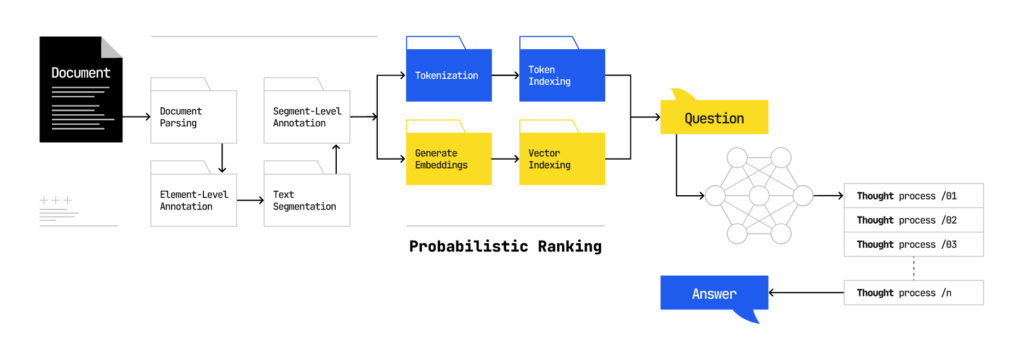
The Overlaps with Classic Organic Search will be Short-Lived
Last month at SEO Week, in my Brave New World of SEO talk, I doubled down by saying that, sure, there is high overlap between the organic SERPs and AI Overviews right now, but we’re not ready for what happens when memory, personalization, MCP, and the requisite agentic capabilities are mixed in. What happens when Google is pulling data from every application on the web?
With the announcement of enhancements to AI Mode, literally everything I said is either now live in your Google Search experience or on the way this year. Google has also been warning us since the launch of their AIO and AI Mode explainer doc that the best of AI Mode will ultimately make its way to the core search experience. The more I’ve researched how these features work, the more adamant I’ve become that our space needs to think bigger.
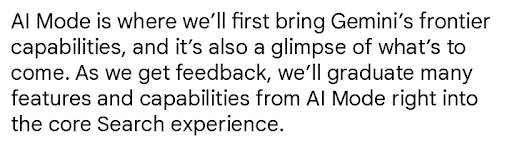
So, let’s talk about why we’re not ready and what we need to do to get ready.
It’s Not Just SEO, but what is SEO Anyway?
The argument that AI Mode and AI Overviews are “just SEO” is short-sighted at best and dangerously misinformed at worst.
What this position gets wrong isn’t just technical nuance; it’s the complete misunderstanding of how these generative surfaces fundamentally differ from the retrieval paradigm that SEO was built on. The underlying assumption is that everything you’d do to show up in AI Mode is already covered by SEO best practices. But if that were true, the industry would already be embedding content at the passage level, running semantic similarity calculations against query vectors, and optimizing for citation likelihood across latent synthetic queries. The shocking lack of mainstream SEO tools that do any of that is a direct reflection of the fact that most of the SEO space is not doing what is required. Instead, our space is doing what it has always done, and sometimes it’s working.
SEO is a Discipline Without Boundaries
Part of the confusion stems from the fact that SEO has no fixed perimeter. It has absorbed, borrowed, and repurposed concepts from disciplines like performance engineering, information architecture, UX, analytics, and content strategy, often at Google’s prompting.

Structured data? Now SEO. Site speed? SEO. Entity modeling? SEO. And the list goes on.
In truth, if every team accounted for Google’s requirements in their own practice areas, SEO as a standalone discipline would not exist.
So what we call SEO today is more of a reactive scaffolding. It’s a temporary organizational response to Google’s structural influence on the web. And that scaffolding is now cracking under the weight of a fundamentally different paradigm: generative, reasoning-driven retrieval and the competition that has arisen on the back of it.
SEO is Not Optimizing for AI Mode
There is a profound disconnect between what’s technically required to succeed in generative IR and what the SEO industry currently does. Most SEO software still operates on sparse retrieval models (TF-IDF, BM25) rather than dense retrieval models (vector embeddings). We don’t have tools that parse and embed content passages. Our industry doesn’t widely analyze or cluster candidate documents in vector space. We don’t measure our content’s relevance across the synthetic query set that’s never visible to us. We don’t know how often we’re cited in these generative surfaces, how prominently, or what intent class triggered the citation. The major tools have recently begun sharing rankings data for AIOs, but miss out on the bulk of them because they track based on logged-out states.
The only part that is “just SEO” is the fact that whatever is being done is being done incorrectly.
AI Mode introduces:
- Reasoning models that generate answers from multiple semantically-related documents.
- Fan-out queries that rewrite the search experience as a latent multi-query event.
- Passage-level retrieval instead of page-level indexing.
- Personalization through user embeddings, meaning every user sees something different, even for the same query in the same location.
- Zero-click behavior, where being cited matters more than being clicked.
These are not edge cases. This is the system.
So no, this is not just SEO. It’s what comes after SEO.
If we keep pretending the old tools and old mindsets are sufficient, we won’t just be invisible in AI Mode, we’ll be irrelevant.
That said, SEO has always struggled with the distinction between strategy and tactics, so it doesn’t surprise me that this is the reaction from so many folks. It’s also the type of reaction that suggests a certain level of cognitive dissonance is at play. Knowing how the technology works, I find it difficult to understand that position because the undeniable reality is that aspects of search are fundamentally different and much more difficult to manipulate.
Google’s Solving for Delphic Costs, Not Driving Traffic
We are no longer aligned with what Google is trying to accomplish. We want visibility and traffic. Google wants to help people meet their information needs and they look at traffic as a “necessary evil.”
Watch the search section of the Google I/O 2025 keynote or read Liz Reid’s blog post on the same. It’s clear that they want to do the Googling for you.
On another panel, Liz explained how, historically, for a multi-part query, the user would have to search for each component query and stitch the information together themselves. This speaks to the same concepts that Andrei Broder highlights in his Delphic Costs paper on how the cognitive load for search is too high. Now, Google can pull from results from many queries and stitch together a robust and intelligent response for you.
Yes, the base level of the SEO work involved is still about being crawled, rendered, processed, indexed, ranked, and re-ranked. However, that’s just where things start for a surface like AI Mode. What’s different is that we don’t have much control over how we show up on the other side of the result.
Google’s AI Mode incorporates reasoning, personal context, and later may incorporate aspects of DeepSearch. These are all mechanisms that we don’t and likely won’t have visibility into that make search probabilistic. The SEO community currently does not have data to indicate performance, nor tooling to support our understanding of what to do. So, while we can build sites that are technically sound, create content, and build all the links, this is just one set of many inputs that go into a bigger mix and come out unrecognizable on the other side.
SEO currently does not have enough control to encourage rankings in a reasoning-driven environment. Reasoning means that Gemini is making a series of inferences based on the historical conversational context (memory) with the user. Then there’s the layer of personal context wherein Google will be pulling in data across the Google ecosystems, starting with Gmail, MCP, and A2A man this is a platform shift and much more external context will be considered. DeepSearch is effectively an expansion of the DeepResearch paradigm brought to the SERP, where hundreds of queries may be triggered and thousands of documents reviewed.
The Multimodal Future of Search
Another fundamental change is that AI Mode is also natively multimodal, which means that it can pull in video, audio, and their transcripts or imagery. There’s also the aspects of Multitask Unified Model (MUM) that underpin this, which can allow content in one language to be translated into another and used as part of the response. In other words, every response is a highly opaque matrixed event rather than the examination of a few hundred text documents based on deterministic factors.
Historically, your competitive analysis compared text-to-text in the same language or video-to-video. Now you’re dealing with a highly dynamic set of inputs, and you may not have the ability to compete.
Google’s guidance is encouraging people to invest in more varied content formats at the same time that they are cutting people’s clicks by 34.5%. It will certainly be an uphill battle convincing organizations to commit these resources, especially when “non-commodity” content won’t have a long life span either. Google is bringing custom data visualization to the SERP based on your data. I can’t imagine remixing your content on the fly with Veo and Imagen are far behind. That alone changes the complexion of what we’re able to strategically accomplish in the context of an organization.
The Current Model of SEO Does Not Support Where Things are Going
I went to sleep the first night of I/O thinking about how futile it will be to log in to much of the SEO software we subscribe to for AI Mode work. It’s pretty clear that, at some point, Google will make AI Mode the default, and much of the SEO community won’t know what to do.
We are in a space where rankings have been highly personalized for twenty years, and still, the best we can do is rank tracking based on a hypothetical user who joined the web for the first time, and their first act is to search for your query. We’re operating on a system that has been semantic for at least ten years and hybrid for at least five, but the best we can do is lexical-based content optimization tools?
Siiiiigh…..there is a lot of work to be done.
Maybe James Cadwallader was Right After All
At SEO Week, James Cadwallader, co-founder and CEO of conversational search analytics platform Profound casually declared that “SEO will become an antiquated function.” He quickly couched that by saying that Agent Experience (AX) is something that SEOs are uniquely positioned to transition to.
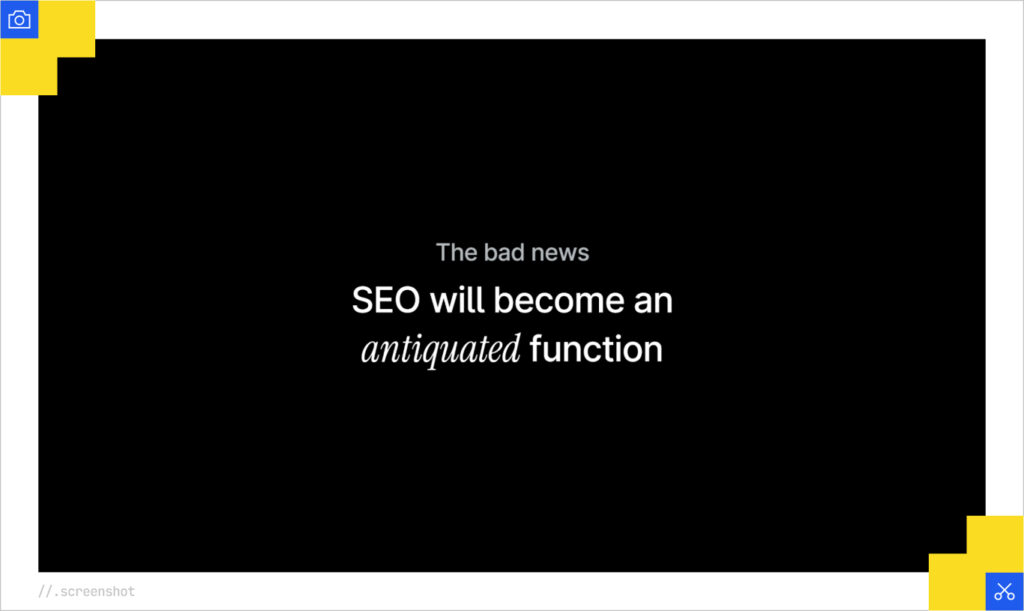
Before he got there, he thoughtfully made his case, explaining that the original paradigm of the web was a two-sided marketplace and the advent of the agentic web upends the user-website interaction model. Poignantly, James concluded that the user doesn’t care where content comes from as long as they get viable answers.
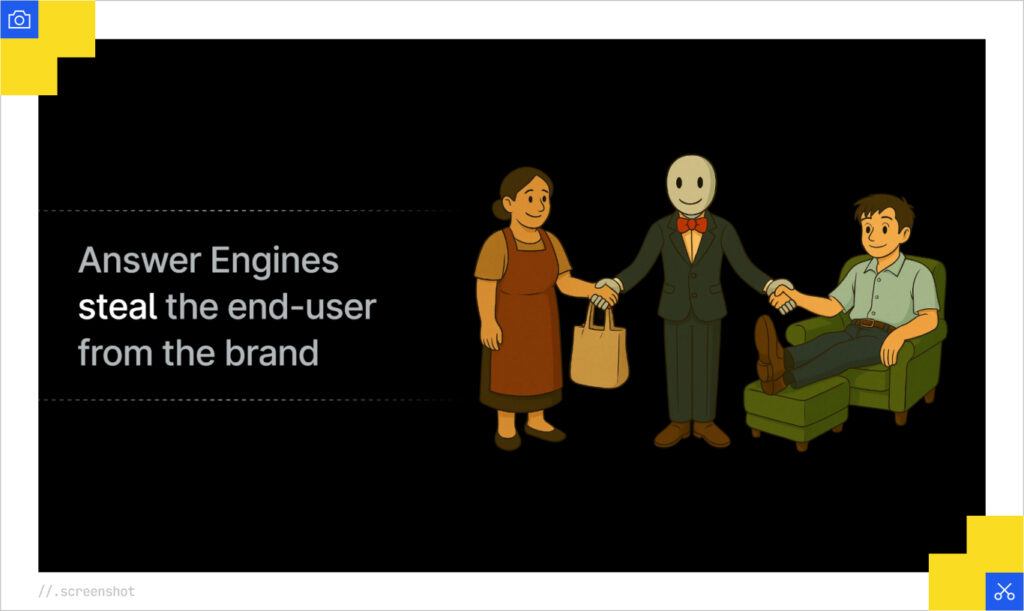
So, while Google has historically warned us against marketing to bots, the new environment basically requires that we consider bots as a primary consumer because the bots are the interpreters of information for the end user. In other words, his thesis suggests that very soon users won’t see your website at all. Agents will tailor the information based on their understanding of the user and their reasoning against your message.
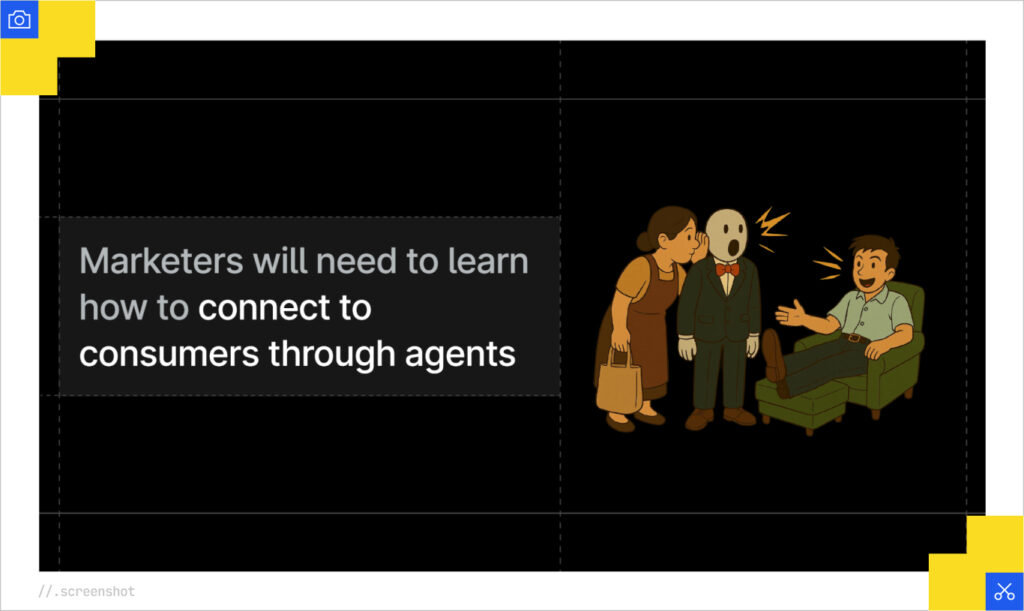
On the technical end, James talked through his team’s hypothesis on how long-term memory works. It sounds as though there’s a representation of all conversations that is constantly updated and added to the system prompt. Presumably, this is some sort of aggregated embedding or another version of the long-term memory store that further informs downstream conversations. As we’ll discuss a few hundred words from here, this aligns with the approach described in Google’s patents.
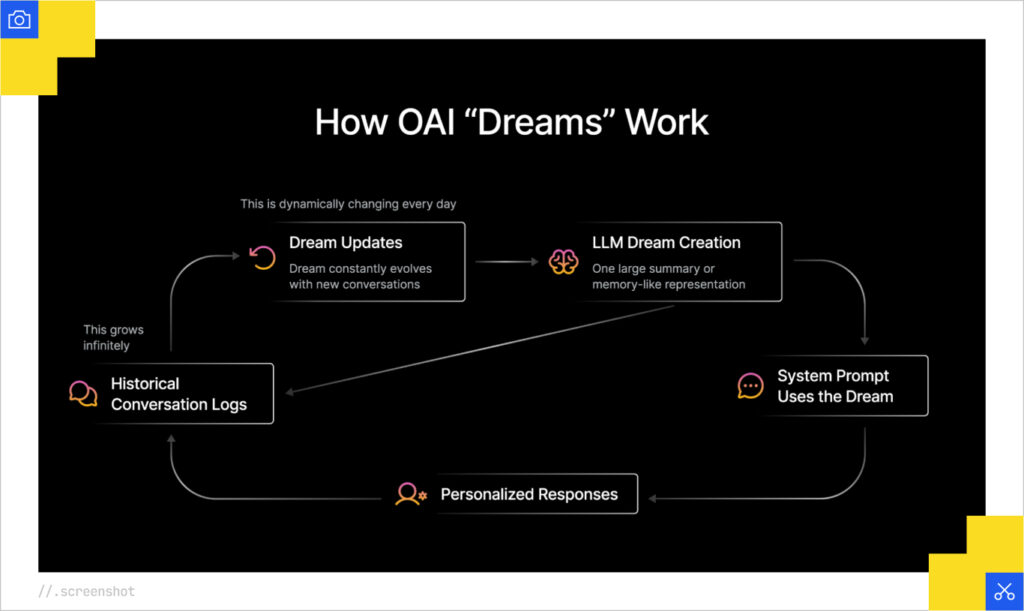
Initially, I thought his conclusions were a bit alarmist, albeit great positioning for their software. Nevertheless, one of the things that I love about Profound is that they are technologists and not beholden to the baggage of the SEO industry. They didn’t live through Florida, Panda, Penguin, or the industry uproar against Featured Snippets. They are clear-eyed consumers of what is and what will be. They operate in the way best-in-class tech companies do, so they are focused on the state of the art and shipping product quickly. Since the I/O keynote, I’ve come to recognize James is right, unless we do something!
James’s talk is more biased towards OpenAI’s offerings, but as we’ve seen, Google is going in an overlapping direction, so I definitely recommend checking it out to give context.
No Data and No Real Direction from Google
At I/O, we had some discussions with Google engineers, and part of the conversation revolved around recognition that the relationship between them and our community is symbiotic, although simultaneously and paradoxically one-sided. After all, the web would not have adopted the secure protocol, structured data, or Core Web Vitals as fast or as completely as it did if our community did not do the legwork to make it happen. I hope whoever had those social engineering OKRs got promoted.
We also discussed how sites are losing clicks due to AIOs, and how we don’t have any data or any air cover from Google to prove to enterprises that the landscape has changed.
I’d suggested that it would have been helpful to have insights from internal usability studies or some results from the Google Labs tests of AIOs to know search behavior is changing. The engineers seemed surprised to hear how universal the click losses have been. Ultimately, we were told, again, that things are moving so fast and are so volatile that it would have been difficult to provide any data or warnings up front to have helped our community through this process. However, there were allusions that there will be future releases that may help. Since that conversation, we’ve gotten a couple of articles on Search Central that allude to the improved quality of visits from search and direction to stop measuring clicks.
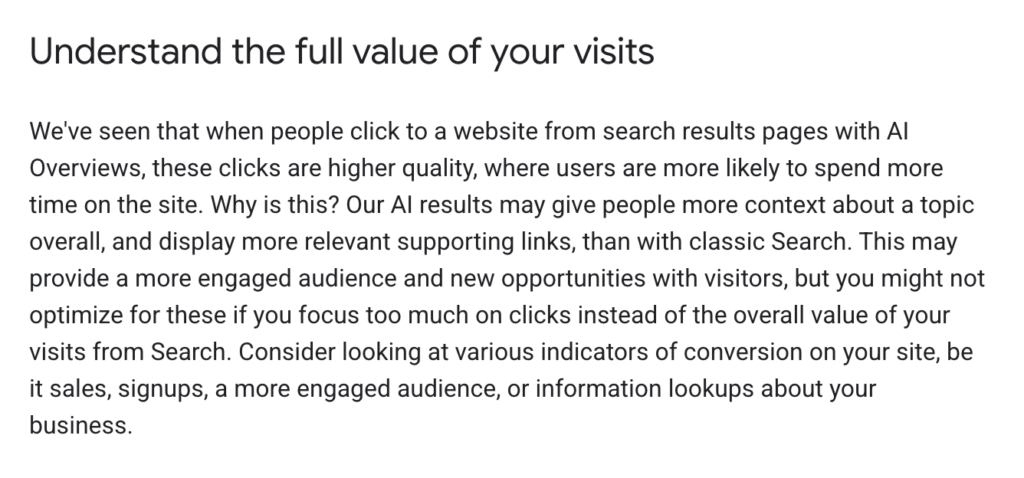
So, I’m not sure whether to say “I’m sorry” or “you’re welcome.” Accept whichever works for you.
However, it’s difficult to hear such things and then learn the next day from the Google Marketing Live event that advertisers will have query-level data about AIOs, but it is what it is.
I also asked what they think our role should be in an agentic environment driven by reasoning, personal context, and DeepResearch. Aside from the standard “create great and unique and non-commodity content,” they said they weren’t sure.
And, that’s fine. We were in a similar position when RankBrain launched, and the party line was that Google didn’t know how their new stuff worked. It’s not like they were going to tell us to start using vector embeddings to understand the relevance of our content. It simply means it’s time to activate our community and get back to experimenting and learning.
Unfortunately, I don’t know that everyone is going to make it through this era. The same way some of the last generation’s SEOs couldn’t survive the paradigm shift post-Panda and Penguin, I suspect some won’t cross the chasm into this next wave of search technology.
Those of us that will, we need to start from an understanding of how the technology works and then work our way back into what can be done strategically and tactically.
No present like the time…I took to figure this out for y’all.
How AI Mode Works
I’m getting tired of watching people rewrite my posts in simpler ways, so we’ll start this with some prose as a simple overview of how AI Mode works. Then we’ll go through it in a more technical form with references to patents.
You can also use this NotebookLM file to get a podcast or ask your own questions to this post.
Prose Version
You open Google and ask it a question. But what happens next doesn’t resemble search as you’ve known it. There are no blue underlines. Just a friendly, context-aware paragraph, already answering the next question before you think to ask it. Welcome to AI Mode.
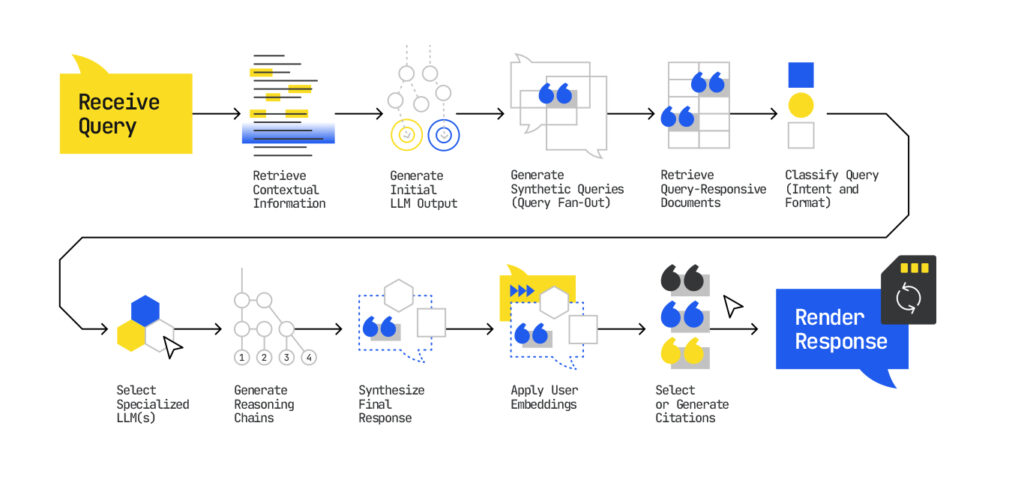
Beneath the surface, what looks like a single reply is actually a matrixed ballet of machine cognition. First, your question is quietly reformulated into a constellation of other questions, some obvious, some implicit, some predictive. Google’s models “fan out” across this hidden web of synthetic queries, scanning not just for facts, but for ideas that can complete a “reasoning chain.”
Behind the scenes, the system isn’t just ranking content, it’s arguing with itself. It selects documents not because they won the SERP, but because they support a point in the machine’s obfuscated logic. Reasoning chains are like those old-school scratch-pad thoughts we all have while solving a problem and are now encoded into how answers are constructed. It’s not “What’s the best electric SUV?” It’s “What does ‘best’ mean to this user, right now, across these priorities?”
And, if that wasn’t enough, the models generating your answer aren’t monolithic. They’re task-specific, tuned and selected based on what kind of answer is needed. A summarizer. A comparer. A validator. It’s an ensemble cast with a rotating spotlight. Each contributes a line; a final model assembles the script.
All of this happens inside an invisible architecture powered by your past. Your clicks, your queries, your location, your Gmail threads are all boiled down into a vectorized version of… “you.”(You read that in Joe Goldberg’s voice, didn’t you?) A personalization layer that doesn’t just color the margins of the result, but warps the very selection of what qualifies as relevant.
And when the answer finally materializes, your webpages might be cited. They might not. Your content might appear not because you were optimized for the keyword, but because a single sentence happened to match a single sub-step in the machine’s invisible logic.
SEO spent the past twenty-five years preparing content to be parsed and presented based on how it ranks for a single query. Now, we’re engineering relevance to penetrate systems of reasoning across an array of queries.
Just mail my Pulitzer to the office.
The Technical Version
Of course, Google has published some high-level documentation on how AI Overviews and AI Mode work. But, you can see from your scrollbar that that is obviously not enough for me. So, in the spirit of the late great Bill Slawski, I’ve done a bit of my own research and uncovered some of Google’s patent applications that align with the functionality that we’re seeing.
The patent application for “Search with Stateful Chat” gives us a foundational understanding of how Google’s AI Mode functions. It marks a departure from classical search into a persistent, conversational model of information retrieval. The system understands you over time, draws from numerous synthetic queries, and stitches answers together using layered reasoning. Additionally, the “Query Response from a Custom Corpus” patent that fills in critical details about how responses are generated. It explains not just what the system knows about you, but how it selects which documents to pull from, how it filters them, and how it decides what to cite.
AI Mode Employs Layered and Contextual Architecture
AI Mode operates as a multi-phase system built on top of Google’s classic index. Instead of treating each query in isolation, it maintains persistent user context by tracking your prior queries, locations, devices, and behavioral signals and turns each interaction into a vector embedding. This stateful context allows Google to reason about intent over time rather than just intent in the moment.
When a new query is entered, AI Mode kicks off a “query fan-out” process (don’t worry the deep-dive on that is coming) and generates dozens (or hundreds) of related, implied, and recent queries to uncover semantically relevant documents the user didn’t explicitly request. Each of these synthetic queries is used to retrieve documents from the index, which are then scored and ranked based on how well their vector embeddings align with both the explicit and hidden queries.
These documents form what the second patent calls a “custom corpus” or a narrow slice of the index that the system has determined is relevant for your query, at this moment, for you. This corpus is the foundation for the rest of the AI Mode response.
AI Mode Uses Multi-Stage LLM Processing and Synthesis
Once the custom corpus is assembled, AI Mode invokes a set of specialized LLMs, each with a different function depending on the query classification and perceived user need. For example, some models may:
- Summarize comparative product reviews
- Translate or localize information across languages
- Extract and format structured data
- Apply reasoning across multiple documents
The patent lists some explicit assessments that are made about how to respond based on the understanding of the user’s information need:
- needs creative text generation
- needs creative media generation
- can benefit from ambient generative summarization
- can benefit from SRP summarization,
- would benefit from suggested next step query
- needs clarification
- do not interfere
From the patent’s description these align with LLMs, however, this is not a classic Mixture of Experts (MoE) model with a shared routing layer. Instead, it’s a selective orchestration where specific LLMs are triggered based on context and intent. It’s closer in spirit to an intelligent middleware stack than a single monolithic model.
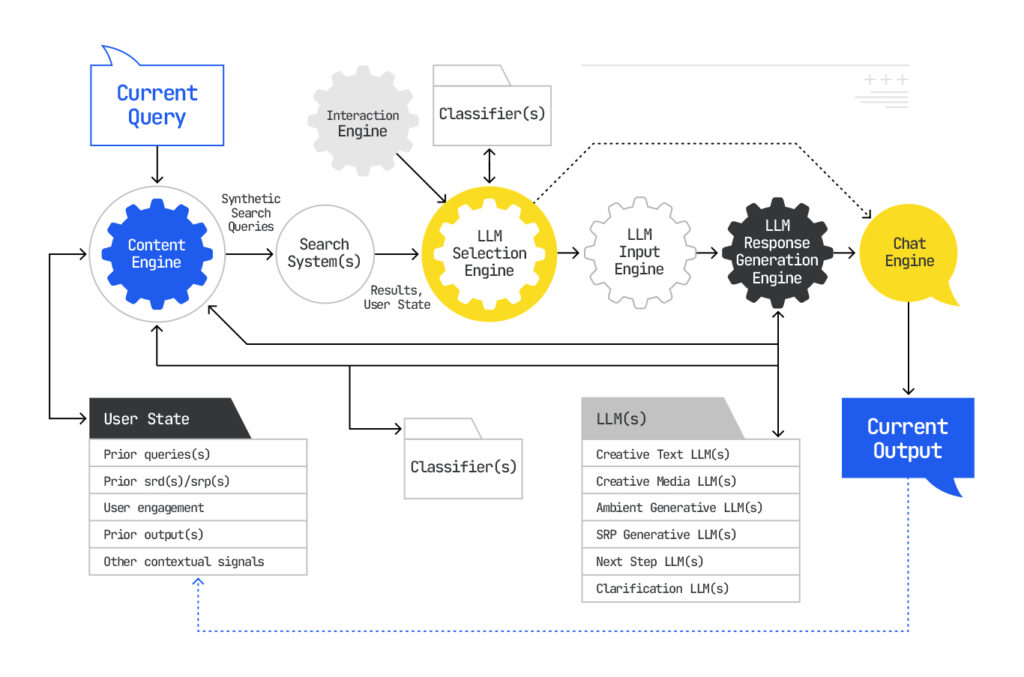
Although there is some discussion of generating hypothetical answers to compare the passages against, the system doesn’t generate responses out of thin air. Instead, as with all RAG pipelines, it extracts chunks from relevant documents, builds structured representations of that information, and synthesizes a coherent answer. Some chunks are cited; many are not. And as “Query response using a custom corpus” patent application describes, citation selection happens independently of document rank, based on how directly a passage supports the generated response.
AI Mode Leverages Dense Retrieval and Passage-Level Semantics
Though we’ve discussed embeddings multiple times, it’s worth saying that this entire pipeline runs on dense retrieval. Every query, subquery, document, and passage is converted into a vector embedding. Google, as Jori Ford reminded me that I’ve repeated ad nauseum for the past few years, calculates similarity between these vectors to determine what gets selected for synthesis. What matters is no longer just “ranking for the query,” but how well your document, or even an individual passage within it, aligns semantically with the hidden constellation of queries.
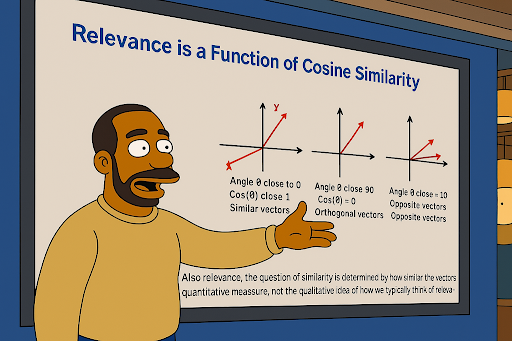
Additionally, Google’s retrieval pipeline no longer operates solely on static scoring functions like TF-IDF or BM25. While hybrid retrieval may still underpin initial candidate selection, the actual ranking and inclusion of content in generative answers increasingly depend on language model reasoning.
According to the “Method for Text Ranking with Pairwise Ranking Prompting” patent application, Google developed a novel system in which an LLM is prompted to compare two passages and determine which is more relevant to a user’s query. This process is repeated across many passage pairs, and the results are aggregated to form a ranked list.
Instead of assigning fixed similarity scores, the system asks: “Given this query, which of these two passages is better?” and lets the model reason it out. This represents a shift from absolute determinative relevance to relative, model-mediated probabilistic relevance. It aligns with AI Mode’s likely behavior, where:
- Dense retrieval surfaces a pool of candidate passages.
- Pairwise LLM prompting selects which passages are most valuable.
- The final synthesis model generates output based on the ranked results.
This has several strategic consequences:
- You’re not competing in isolation, you’re being compared directly to other sources chunk-by-chunk.
- The winner is chosen by a model capable of reasoning, not just counting tokens or (yikes) keyword density.
- Passage clarity, completeness, and semantic tightness become even more critical because your content must survive pairwise scrutiny.
The implication is clear: it’s not enough to rank somewhere for a topic. You must engineer passages that can outperform competing content head-to-head in LLM evaluations, not just semantic similarity.
AI Mode Has Ambient Memory and Adaptive Interfaces
“Stateful chat” means Google accumulates an ambient memory of you over time just like James described for OpenAI. As described in the Search with stateful chat patent, these “memories” are likely aggregated embeddings representing past conversations, topics of interest, and search patterns. The interface itself adapts too, drawing from what we saw demonstrated in the Bespoke UI demo from last year.
It dynamically determines which elements (text, lists, carousels, charts) to display based on the information need and content structure. I highlighted this video in my talk at Semrush’s Spotlight conference last year as an indication of the future of search interfaces. When I first saw it, I knew we were in for something! Now we know that this functionality is powered by one of the downstream LLMs in the AI Mode pipeline.
AI Mode Does Personalization Through User Embeddings
A foundational innovation enabling AI Mode’s contextual awareness is the use of “user embedding” models as described in User Embedding Models for Personalization of Sequence Processing Models patent application. This personalization mechanism allows Google to tailor AI Mode outputs to the individual user without retraining the underlying large language model. Instead, a persistent dense vector representation of the user is injected into the LLM’s inference pipeline to shape how it interprets and responds to each query.
This vector, called a user embedding, is generated from a user’s long-term behavioral signals: prior queries, click patterns, content interests, device interactions, and other usage signals across the Google ecosystem. Once computed, the user embedding acts as a form of latent identity, subtly influencing every stage of AI Mode’s reasoning process.
In practice, this embedding is introduced during:
- Query interpretation: altering how the model classifies intent,
- Synthetic query generation: shifting which fan-out queries are prioritized,
- Passage retrieval: re-ranking results based on individual affinity,
- Response synthesis: generating text or selecting formats (e.g., video, list, carousel) aligned with the user’s past preferences.
Importantly, this system allows for modular personalization: the same base model (e.g., Gemini) can serve billions of users while still producing individualized results in real time. It also introduces cross-surface consistency. The same user embedding could inform personalization across Search, Gemini, YouTube, Shopping, or Gmail-based recommendations. In fact, Tom Critchlow showcased on Twitter that he got the same response in both AI Mode and Gemini.
No pun, but the implication is profound. AI Mode is no longer just intent-aware; it’s memory-aware. Two users asking the same query may see different citations or receive different answers, not because of ambiguity in the query, but because of who they are. That makes inclusion a function of both semantic relevance and profile alignment. That means logged-out rank tracking data is meaningless for AI Mode because responses can be 1:1.
The SEO Takeaway for AI Mode
AI Mode rewrites the rules. You’re no longer optimizing for a specific keyword or even a specific page. You’re optimizing for your content to be semantically relevant across dozens of hidden queries and passage-competitive within a custom corpus. Your ranking is probabilistic, not deterministic, and your presence in the result depends as much on embedding alignment as it does on authoritativeness or topical breadth.
To compete, you need to:
- Influence user search behavior through other branding channels
- Engineer content at the passage level for both semantic similarity and to be LLM-preferred
- Understand and anticipate synthetic query landscapes
- Optimize for semantic similarity and triple clarity
- Track rankings through profiles with curated user behaviors
This isn’t traditional SEO. This is Relevance Engineering (r17g). Visibility is a vector, and content is judged not only on what it says, but how deeply it aligns with what Google thinks the user meant.
How Query Fan-Out Works
The query expansion technique Google refers to as “query fan-out” is fundamental to how AI Mode retrieves and selects content. Rather than issuing a single search, Google extrapolates the original query into a constellation of related subqueries in parallel. Some of these synthetic queries are directly derived, others inferred or synthesized from user context and intent. These queries span various semantic scopes and are used to pull candidate documents from the index. This enables Google to capture intent that the user didn’t, or couldn’t, explicitly express.
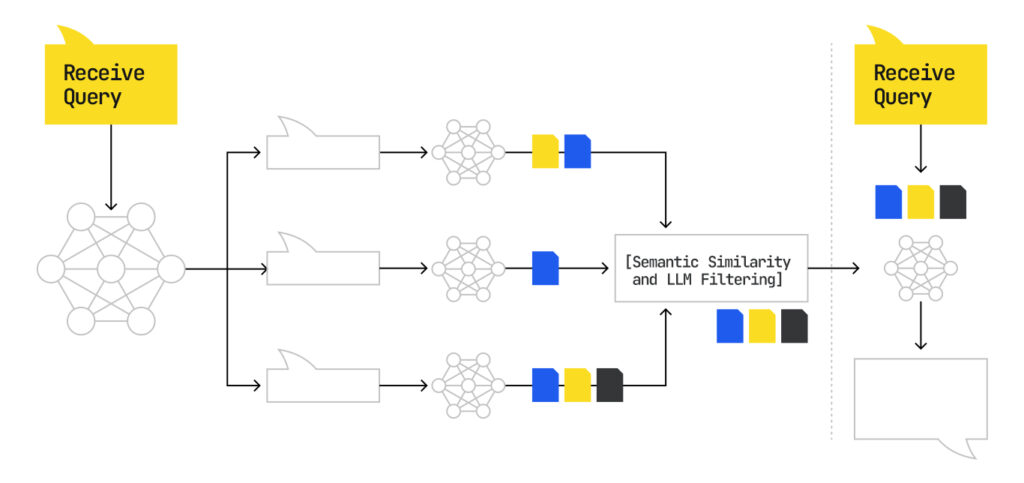
Google proudly discusses the concept from a high-level in the recent public documents, but the patent application Systems and methods for prompt-based query generation for diverse retrieval, offers a detailed blueprint of how query fan-out works. The process begins with a prompted expansion stage, where a LLM is used to generate multiple alternate queries from the original query. The model doesn’t hallucinate queries at random it is instructed with a structured prompt format that emphasizes:
- Intent diversity (e.g. comparative, exploratory, decision-making)
- Lexical variation (e.g. synonyms, paraphrasing)
- Entity-based reformulations (e.g. specific brands, features, topics)
Query Fan Out Synthetic Query Types
The query fan out process considers an array of different approaches to construct synthetic queries. Based on the various patents, the table below outlines the types of synthetic queries used:
Synthetic Query Type | Definition | Trigger Condition | Role in AI Mode | Example (Base Query: “best electric SUV”) | Patent Source(s) |
Related Queries | Queries that are semantically or categorically adjacent to the original query, often linked via entity relationships or taxonomy. | Recognized co-occurrence patterns or topical proximity in the Knowledge Graph. | Expands retrieval scope to cover similar or overlapping domains of interest. | “top rated electric crossovers” “best hybrid SUVs” | WO2024064249A1, US20240362093A1 |
Implicit Queries | Queries inferred from user intent, behavioral signals, or language model reasoning—what the user likely meant but didn’t explicitly say. | LLM inference based on phrasing, ambiguity, and historical user behavior. | Helps the model fulfill the deeper or unstated information need of the user. | “EVs with longest range” “affordable family EVs” | WO2024064249A1, US20240289407A1 |
Comparative Queries | Queries that compare products, entities, or options. Often synthesized when the user is making a choice or decision. | Classifier detects decision-making or ambiguity in original query. | Triggers retrieval of structured or contrastive content for synthesis and re-ranking. | “Rivian R1S vs. Tesla Model X” “EV SUV comparison chart 2025” | WO2024064249A1, US20240362093A1 |
Recent Queries | Queries recently issued by the user, used to inform contextual understanding and query expansion in session-based or memory-informed search. | Prior queries in the session or search history retrieved via contextual layer. | Used to maintain conversational state and personalize fan-out expansion or synthesis. | (Prior queries: “EV rebates in NY” → “best electric SUV”) | US20240289407A1 |
Personalized Queries | Queries aligned to a specific user’s interests, location, or behavioral history (via embeddings). | Retrieved from long-term user memory or injected user profile embeddings. | Refines retrieval to reflect the unique context and past behavior of the individual user. | “EVs with 3rd row seating near me” “EVs eligible for CA rebate” | WO2025102041A1, US20240289407A1 |
Reformulation Queries | Lexical or syntactic rewrites that maintain core intent but use different phrasing or vocabulary. | Generated via prompt-based rewriting using LLMs (e.g., Gemini). | Increases lexical diversity of query fan-out to capture alternate phrasings of the same intent. | “which electric SUV is the best” “top EV SUVs for 2025” | WO2024064249A1, WO2025102041A1 |
Entity-Expanded Queries | Queries that substitute, narrow, or generalize based on entity relationships in the KG. | LLM crosswalks entity references to broader/narrower equivalents. | Broadens or specifies scope using KG anchors—e.g., replacing “SUV” with specific models or brands. | “Model Y reviews” “Volkswagen ID.4 vs Hyundai Ioniq 5” | WO2024064249A1, US20240362093A1 |
Each of these is then routed through Google’s embedding-based retrieval system to locate relevant passages. What’s most important here is that ranking for the original query no longer guarantees visibility, because AI Mode is selecting content based on how well it aligns with one or more of the hidden fan-out queries, which, again, makes ranking in AI Mode a complex matrixed event.
Query Fan Out Filtering and Diversification
The Systems and methods for prompt-based query generation for diverse retrieval patent further outline a filtering mechanism to ensure the selected queries:
- Span multiple query categories (e.g., transactional, informational, hedonic)
- Return diverse content types (e.g., reviews, definitions, tutorials)
- Avoid overfitting to the same semantic zone (e.g., ensuring information diversity)
This helps Google build a more well-rounded and informative synthesis, pulling not just from the best-ranking document but from a custom corpus rich in contextual diversity. In other words, it’s not enough to just say what the competition is saying.
Query Fan-out Prompt-Based Chain of Thought
To improve quality and relevance, the synthetic query generation process may also include chain-of-thought prompting, where the LLM walks through reasoning steps like:
- What the user is likely trying to achieve
- What aspects of the original query are ambiguous or expandable
- How to reframe the query to cover those needs
In other words, the LLM doesn’t just output alternate queries. It explains why each was generated, often using task-specific reasoning or structured intents (e.g., “Help the user compare brands,” “Find alternatives,” “Explore risks and benefits”).
The SEO Implication of Query Fan-Out
As I’ve learned more about query fan-out, I recognize that I wasn’t aware of it as a key aspect of AI Overviews. Early reports of AI Overviews pulling content from deep in the SERPs likely misunderstood what was happening. It’s probably not that Google’s AI was reaching far down the rankings for a single keyword; it was reaching across rankings for a different set of background queries entirely. So while SEOs are tracking position for [best car insurance], Google may be selecting a passage based on how well it ranks for [GEICO vs. Progressive comparison chart for new parents]. Based on ZipTie’s latest data, ranking #1 for the core query only gives you a 25% chance at ranking in the AIO.
To surface in AI Mode, you must ensure:
- Your content ranks for multiple potential subqueries
- Your passages are semantically dense and well-aligned with diversified intents
- You engineer relevance not just for head terms, but for the expanded query space Google is quietly exploring in the background
How Reasoning Works in Google LLMs
One of the defining features of Google’s AI Mode is its ability to reason across a corpus of documents to generate multi-faceted answers. The “Instruction Fine-Tuning Machine-Learned Models Using Intermediate Reasoning Steps” patent describes a system for constructing and using “reasoning chains.” These are structured sequences of intermediate inferences that connect user queries to generated responses in a logically coherent way. While this may not be the exact patent for how reasoning functions in AI Mode, it does give a sense of reasoning approaches that have informed iterations of Google’s models.
Rather than relying on end-to-end generation or selecting standalone answers, this system enables Google to:
- Interpret the user’s intent and implicit needs
- Formulate intermediate reasoning steps (e.g., “the user wants an SUV suitable for long commutes, so prioritize range and comfort”)
- Retrieve or synthesize content for each step
- Validate the final output against the logic of those steps
These reasoning chains may be segmented into the following groups:
- In-band – Steps generated as part of the LLM’s main output stream (e.g., via chain-of-thought prompting)
- Out-of-band – Steps created and refined separately from the final answer, then used to guide or filter that response
- Hybrid – Steps used for query expansion, document filtering, synthesis structuring, and validation at different points in the pipeline.
This is a dramatically different operation from what we are historically used to in SEO. The job now needs to include tactics to remain relevant throughout all these reasoning steps.
How Reasoning Works in the AI Mode Pipeline
Contextualizing everything we’ve learned with the steps in the Search with stateful chat patent application, we can get a sense of how and where reasoning is applied.
Stage | How Reasoning Is Applied |
Query Classification | LLM generates initial reasoning hypotheses: What does the user likely mean? What decision-making path are they on? |
Query Fan-Out | Synthetic queries are generated based on inferred reasoning needs e.g., comparing features, exploring risks, looking for alternatives. |
Corpus Retrieval | Reasoning chains determine which types of content or perspectives are required to fulfill each step, resulting in more targeted document selection. |
LLM Selection and Task Routing | Specific models are chosen for subtasks based on the reasoning structure (e.g., use Model A for extraction, Model B for summarization, Model C for synthesis). |
Final Synthesis | Reasoning chains serve as scaffolds for answer construction with each part of the response aligning with one or more logical steps. |
Citation | Passages that most directly support individual reasoning steps are cited not necessarily the highest-ranking or most comprehensive document. |
In other words, reasoning pretty much touches every stage of the process. And that process is more opaque than anything we’ve ever been up against.
How to Structure Content to Pass Through Reasoning Layers
Content that appears in AI Mode doesn’t just need to be indexable and informative. It’s no longer enough for content to be “generally relevant.” It must be granularly useful, retrievable by step, and semantically aligned with each logical inference. It must be designed to win at multiple reasoning checkpoints. These include passage-level embedding similarity, comparative re-ranking, and natural language generation modeling.
There are four strategic pillars for creating content that succeeds in AI Mode, each of which corresponds to a specific set of content characteristics. They are as follows:
- Fit the Reasoning Target – Content should be semantically complete in isolation, explicitly articulate comparisons or tradeoffs, and be readable without redundancy. These qualities ensure it can be effectively evaluated and selected by reasoning models during pairwise ranking or summarization tasks.
- Be Fan-Out Compatible – To align with the subqueries generated during query expansion, content must include clearly named entities that map to the Knowledge Graph and reflect common user intents such as evaluation, comparison, or constraint-based exploration.
- Be Citation-Worthy – Content needs to present factual, attributable, and verifiable information. This includes using quantitative data, named sources, and semantically clear statements that LLMs can extract with high confidence.
- Be Composition-Friendly – Structure content in scannable, modular formats such as lists, bullet points, and headings (like I’ve done heavily throughout this article). Use answer-first phrasing and include elements like FAQs, TL;DRs, and semantic markup to make the content easily composable during synthesis.
Use this table as your guide for how to implement these characteristics into your content engineering efforts.
Characteristic | Why It Matters | What It Looks Like | Strategic Function |
Semantically Complete in Isolation | LLMs retrieve and reason at the passage level not the whole page. A passage must answer or contextualize a specific subquery on its own. | “The Tesla Model Y offers 330 miles of range, advanced driver assistance, and a spacious interior. Compared to the Ford Mustang Mach-E, it provides more range but less trunk space.” | Fit the Reasoning Target |
Explicit About Comparisons or Tradeoffs | Many generative prompts involve choice-making. Content that articulates pros, cons, and “why X over Y” survives better in LLM pairwise ranking and synthesis. | “The Rivian R1S is ideal for off-road enthusiasts due to its ground clearance and quad-motor system, while the Tesla Model X excels in highway efficiency and autonomous features.” | Fit the Reasoning Target |
Entity-Rich and Knowledge Graph-Aligned | Entity linking helps AI systems disambiguate and retrieve content via fan-out expansions. Specific brand, product, and category names improve visibility. | “The Hyundai Ioniq 5, classified as a compact crossover SUV, is built on Hyundai’s E-GMP platform and supports 800V ultra-fast charging.” | Be Fan-Out Compatible |
Structured in Scannable Chunks | Content needs to be modular and extractable LLMs recombine pieces, not full documents. Clear structure enables chunk selection and formatting in synthesis. | Pros – 300-mile range – Fast charging Cons – Limited rear visibility – No Apple CarPlay support | Be Composition-Friendly |
Contextualized With Intent Language | Generative systems favor passages that reflect user goals (e.g., shopping, comparing, troubleshooting). Intent-aligned phrasing improves alignment. | “If you’re shopping for a reliable EV under $50K with high safety scores and fast charging, the Kia EV6 is a standout option.” | Be Fan-Out Compatible |
Readable and Free of Redundancy | Redundant, bloated, or repetitive language weakens LLM performance and increases likelihood of exclusion in pairwise ranking or synthesis. | ✘ “The Tesla Model Y is great. The Model Y is great because it’s great for families. Families love the Model Y.” ✔ “The Tesla Model Y combines long range with family-friendly design and seating for up to seven.” | Fit the Reasoning Target |
Inherently Answer-Oriented | LLMs are often asked direct questions. Content that reflects clear answers, especially early in a paragraph or section, is more likely to be used in generation. | “Yes, the federal tax credit applies to the 2024 Mustang Mach-E if it meets final assembly and battery sourcing requirements.” | Be Composition-Friendly |
Factual, Attributable, and Verifiable | Citation-worthy content must present facts clearly, avoid speculation, and include attributes like sources or structured claims (semantic triples). | “The 2024 Ioniq 5 has an EPA-estimated range of 303 miles and supports 350kW DC fast charging.” Source: U.S. Department of Energy, March 2024. | Be Citation-Worthy |
Based on how these systems function, these are all things you’ll need to do to be in the candidate documents, but there is no guarantee that you’ll get any visibility from any of this if you are misaligned with the user embeddings.
This indicates that a big part of the job now is building user embeddings that represent the activities of your targets and simulating how your content appears on the other side of the pipelines. We also need to know where we stand in all the queries being considered.
Meet Qforia
When faced with a complex problem like this, I put on my Relevance Engineer hat and think about how I would build something like this. This is how we developed our deep understanding of AIOs prior to their launch back in 2023. However, the complexity of AI Mode renders it difficult to replicate the product quickly with a simple RAG pipeline. I suspect it will be a few more weeks before I’ve built an AI Mode proof of concept.
Instead, I’ve been working on replicating the query fan-out idea in service of our AIO Simulator tool. I started by parsing features from the primary query and its SERP like entities, PAAs, related queries, etc. That approach got to something that worked, but perhaps did not surface enough variance. After learning that Gemini itself is used to build the list, I continued to explore other potential ways to do it.
At I/O, JC Chouinard shared a May 5th, 2023, paper entitled “Query Expansion by Prompting Large Language Models” with me. The paper, from the Google Research team, describes a Chain-of-Thought prompting technique for expanding queries. So I tinkered with a series of prompts until I got something that yielded results that I assume are reasonable. Then I did some digging of my own and found the aforementioned Systems and methods for prompt-based query generation for diverse retrieval patent application that explains in more detail how queries are selected in the query fan-out process.
Using similar methodologies, we can identify several types of the related and implied queries. However, we won’t have any visibility into a user’s recent queries.
Sidebar: I did some digging to see if Google has exposed the query fan-out data publicly. I’m not ready to talk about that, but there is a URL in the network requests when AI Mode is loading that responds with recent queries and a few other parameters.
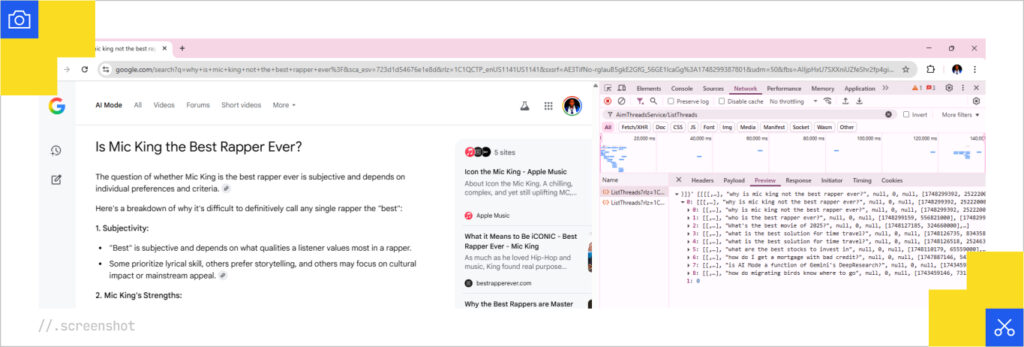
The URL looks like:
https://www.google.com/httpservice/web/AimThreadsService/ListThreads?rlz=XXX&sca_esv=YYY&udm=50&reqpld=[null,null,0]&msc=gwsclient&opi=ZZZZ
Hopefully, one of the clickstream data providers will start to intercept the call and collect that data to add to their offerings.
The result is a simple app I’ve built with the latest Gemini 2.5 Pro called Qforia. Based on the initial query, it generated a series of queries, the type of synthetic query, the user intent, and the reasoning behind why the query was selected.
Since AI Mode is more complex and surfaces more queries, Qforia does the same, but in both situations, it asks the model to determine how many queries are required. When it gives its output, it shares its reasoning behind the number of queries it selected.
How to Use Qforia
Qforia is a simple tool. You put in a query and an API key, and you get a bunch of potential searches back in alignment with the various types of synthetic queries that Google is generating during query fan-out.
Here’s your step-by-step to getting started with it:
- Grab a Gemini key and put it in the API Key input box. This requires a paid key to be set up because it’s using the latest capabilities of the latest model. If you get a free key, it will fail.
- Put in your query in the query text area. The more complex the query, the more results you’re likely to get.
Select AI Overview or AI Mode and click “Run Fan Out.”
Here’s an example of an AI Overview result:
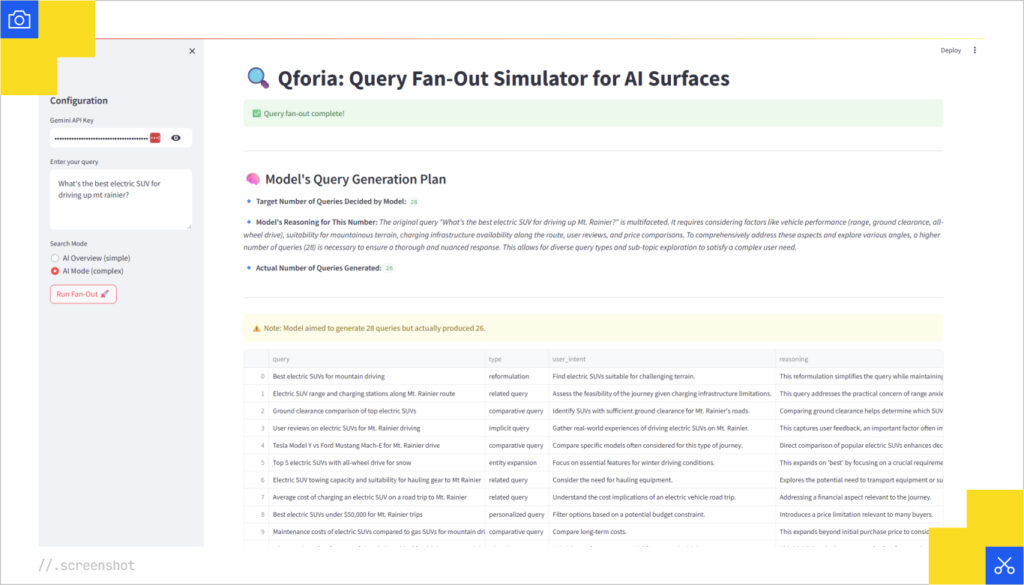
Here’s an example of an AI Mode result for the same query. Note that it attempted 28 queries, but only returned 26.
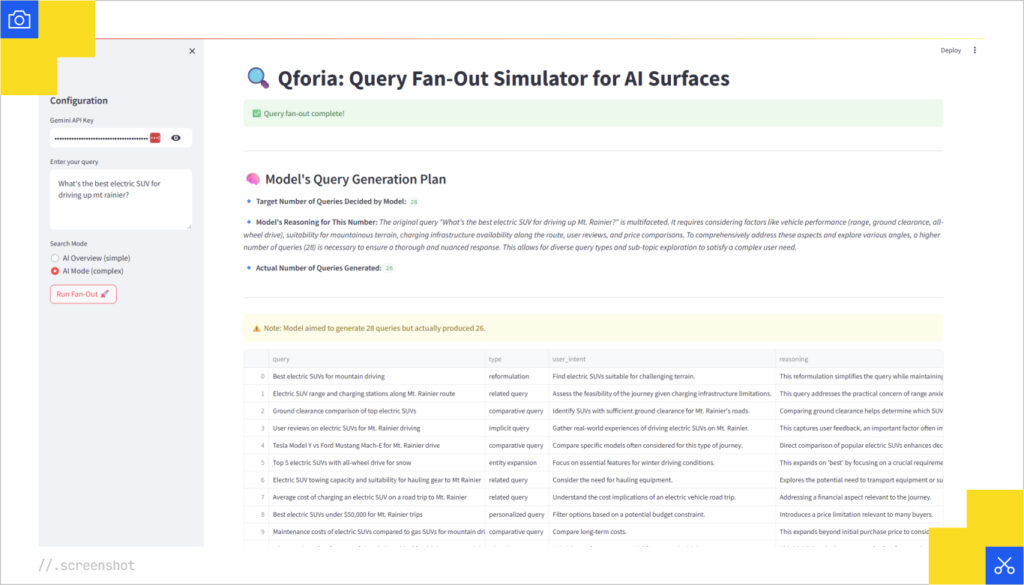
- Export your query data
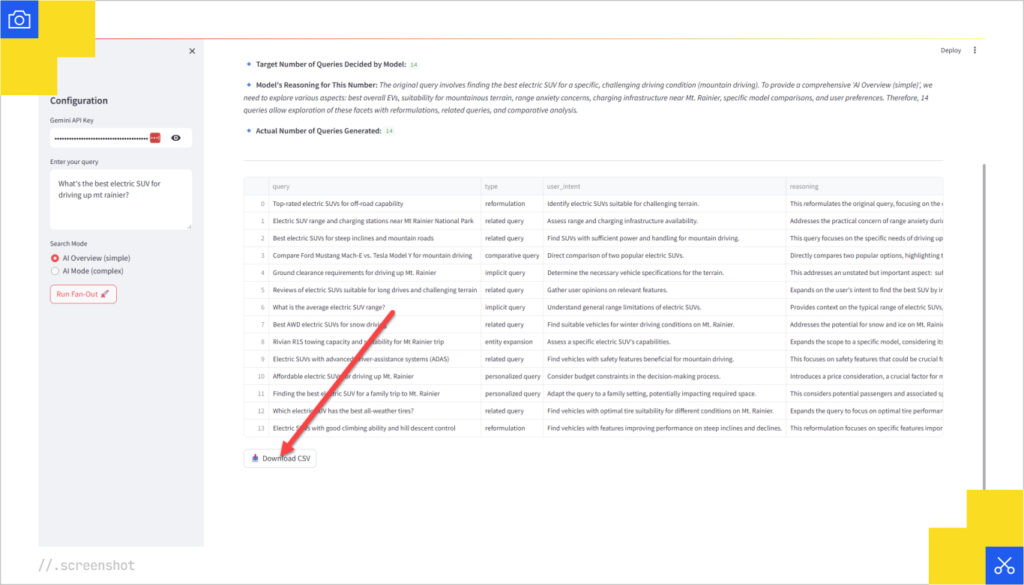
From here, you would need to pull the rankings for these keywords, vectorize all passages on yours and the competitor passages that made it through to the citations in AI Mode. Then you’d improve your passage copy for better performance in the pipeline. Unfortunately, there are no SEO tools to support this, because this isn’t SEO, it’s Relevance Engineering. Now with Qforia, you are a bit less in the dark about what queries Google might be looking for.
AI Mode Requires Matrixed Ranking Strategies
Based on what I’ve learned about how the technology works, the approach to ranking in the AI Mode surface needs to be matrixed. The goal is to have Gemini run into you for as many of the synthetic queries as possible and to make your message the most relevant at every reasoning turn.
Let’s think back to passage indexing. Google has a granular understanding of pages, so ideally, you’d have a single robust page that covers everything across all the subqueries. That way, you’ll only need to focus on a single page. However, in some cases, you may need multiple pages, and reviewing rankings data from the synthetic queries will reveal that.
One could argue that if you are doing topical clustering, you’re already doing this. However, in practice, the members of a topical cluster, as they are currently defined, are subjective, just like our historical understanding of relevance. Instead, these need to be data-driven based on actual user journeys and what Gemini derives during query fan-out.
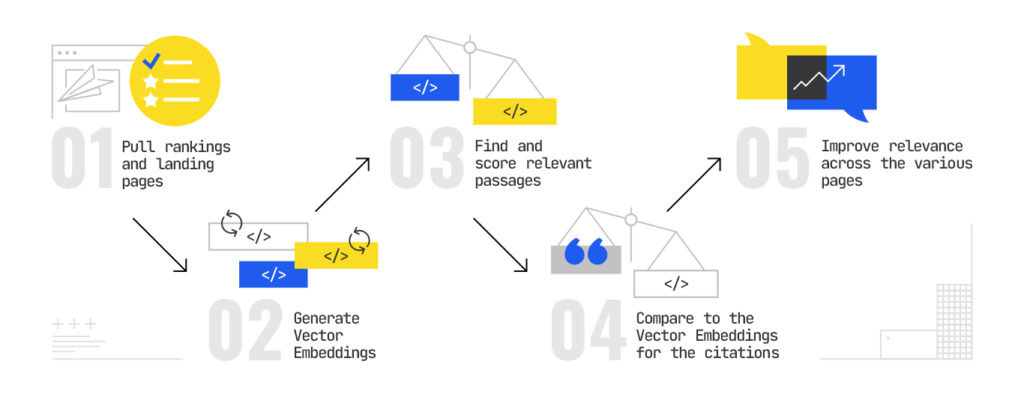
Now that we have a sense of the potential underlying queries, we need to build out a matrix of keywords and see how well we rank for the subqueries. Here’s how we’d do this:
- Pull rankings and landing pages for subqueries – This is straight forward, and really the only thing existing SEO technology can help us out with here.
- Generate Vector Embeddings for the queries and all passages in each of our documents – We need to generate embeddings for each passage in our document(s) so we can replicate the passage indexing. Google’s embeddings are currently at the top of the leaderboard and they are the only provider that makes a distinction between query and document embeddings. So, they are the best for this purpose.
- Find and score the most relevant passages from each document – With the mapping of keyword embeddings and passages, we need to find the most relevant passages in each of our documents by computing cosine similarity.
- Compare them to the Vector Embeddings for the citations – From there, we need to generate vector embeddings from the citations highlighted in the AI Mode response. Then we need to compare the score for our embeddings versus the score for the embeddings in the citations.
- Improve relevance across the various pages – Any of the instances where we got the lower scores, we need to go back and engineer the relevance of that content by improving the semantic chunking, statistics, readability, and usage of semantic triples as we discussed above.
This process is more than SEO because there is no SEO software that will get you passage-level embeddings. There’s no SEO software that will calculate the relevance score on a passage level. There’s no SEO software that will help you identify synthetic queries. There’s no SEO software that will help you optimize across multiple pages at once with the goal of improving your visibility. There’s no SEO software that exists to help you optimize for AI Mode. As of this writing, you’d have to write your own code to do what I just walked you through.
In other words, you need to engineer your relevance.
Does the Rank Tracking Paradigm Still Make Sense for AI Mode?
Rank Tracking has been on shaky ground for a long time. As a quick recap, despite personalization, rank tracking tries to replicate a user context that doesn’t exist to indicate visibility. Due to the highly dynamic nature, it has no place in AI Mode. Since personalization is so deeply baked into the experience, data from the logged out state is inaccurate. In fact, much of the data we make decisions from in SEO is inaccurate, but precise. That may have been good enough in a deterministic environment, but doesn’t work in a probabilistic one.
The Profound team has been defining what analytics looks like for conversational search surfaces like AIOs, ChatGPT, Perplexity, and CoPilot. So I reached out to them to see what they are thinking. Profound’s AI Strategist Josh Blyskal had this to say:
“We’re bullish on AI Mode. It’s already the most-used answer engine worldwide, and we don’t see that changing anytime soon. Generative answers in a conversational interface represent a fundamentally better method of information retrieval.
We expect the tracking of AI Mode to become more similar to what we’ve seen across ChatGPT and Perplexity. Brands will focus on visibility, sentiment and citations within AI Mode responses.
AI Mode will very likely heavily leverage Google’s Knowledge Graph and Shopping Graph. So, for straightforward searches like 'what’s the best corporate credit card,' answers could be more aligned to regular Google results.
Profound is already working on technology to help brands show up more frequently in AI Mode.”~ Josh Blyskal
This is an extension of the question I asked James onstage at SEO Week. I questioned what analytics even looks like in a highly personalized environment, and we agreed there is a need for persona-based tracking. That means the “ranking” for AI Mode will need to be tracked in a logged-in state for a user whose context in the Google environment matches your target audience.
Hear that? If you listen closely, you’ll catch the sound of ChatGPT Operator and Google Project Mariner rank intelligence apps starting up and inflating your search volume.
AI Mode Benefits from Multimodal Content Strategy
Last year’s leaked documents revealed that formats are taken into account when selecting what can rank. The implication is that there are a finite number of slots for a given content type for certain SERPs. AI Mode shifts the balance and changes what qualifies as content. This system synthesizes experiences by pulling from a range of formats including text, audio, video, images, and dynamic visualizations. In this environment, relying solely on text-based content is not just limiting. It risks being left out altogether.
Google’s AI pipeline can transcribe videos, extract claims from podcasts, interpret diagrams, and remix all of it into new outputs such as lists, summaries, or visual presentations. A product video might supply a quote. A podcast might provide a data point. An infographic could become a generated answer in text. The format matters as much as the content itself.
Early in the AI Mode process, Google’s system classifies not just the query type, but also the ideal output modality. If a visual or spoken explanation is considered more useful than a written one, AI Mode may prioritize those formats over traditional web pages. That means a more accurate article might be ignored in favor of a relevant clip or visual explanation.
Organizations must start thinking about content in terms of format-level coverage. Just as we now plan for clusters of related queries and user intents, we must also plan for clusters of related formats. Your goal is not just to be the most relevant article. It is to be the most relevant video, the most relevant chart, the most relevant soundbite.
If you are not producing those formats, Google may still reconstruct them from your content. But it may do so without citing you. Multimodal content creation is no longer just a visibility advantage. It is a strategy for controlling how your brand is represented.
In AI Mode, the winners are those who build content ecosystems, not just content pages. Visibility now depends on being present in all the places the system might look, across every format it can process.
The New SEO Software Requirements for AI Surfaces
It has become increasingly clear that most popular SEO software is not doing enough to support modern SEO. The reason why “Python SEO” exists is a function of SEO software not being state-of-the-art. Our collective lack of technical standards is why it’s so behind, but here are some key features and functionality that you, as a user, need to demand from your software providers to support your ability to engineer visibility moving forward. For the Relevance Engineers, this is what it will take for your personal toolkit to be feature complete for AI Overviews and AI Mode.
AI Search Measurement in Google Search Console
Let’s start with the main culprit, Google itself. Google Search Console is such a strange and hampered product. Like, what is the point of the Links Report? What does anyone use that for?
The whole platform is a data tease. Most reports limit you to 1000 results in the paginated series. Unless you’re clever with your filters or use the API, you can’t get much out of it efficiently. If you get too far into the year, you can’t do YoY comparisons without warehousing the data. Everything about it is inefficient. When you compare its crawl stats to your own verified Googlebot crawl data, the numbers are way off.
But I digress. Right now, we have no visibility into how AIOs or AI Mode perform. As of this writing, there is a noreferrer tag set in the experience (apparently this is a bug), so the little AI Mode traffic you’ll get will show up at Direct. All of this is laughable.
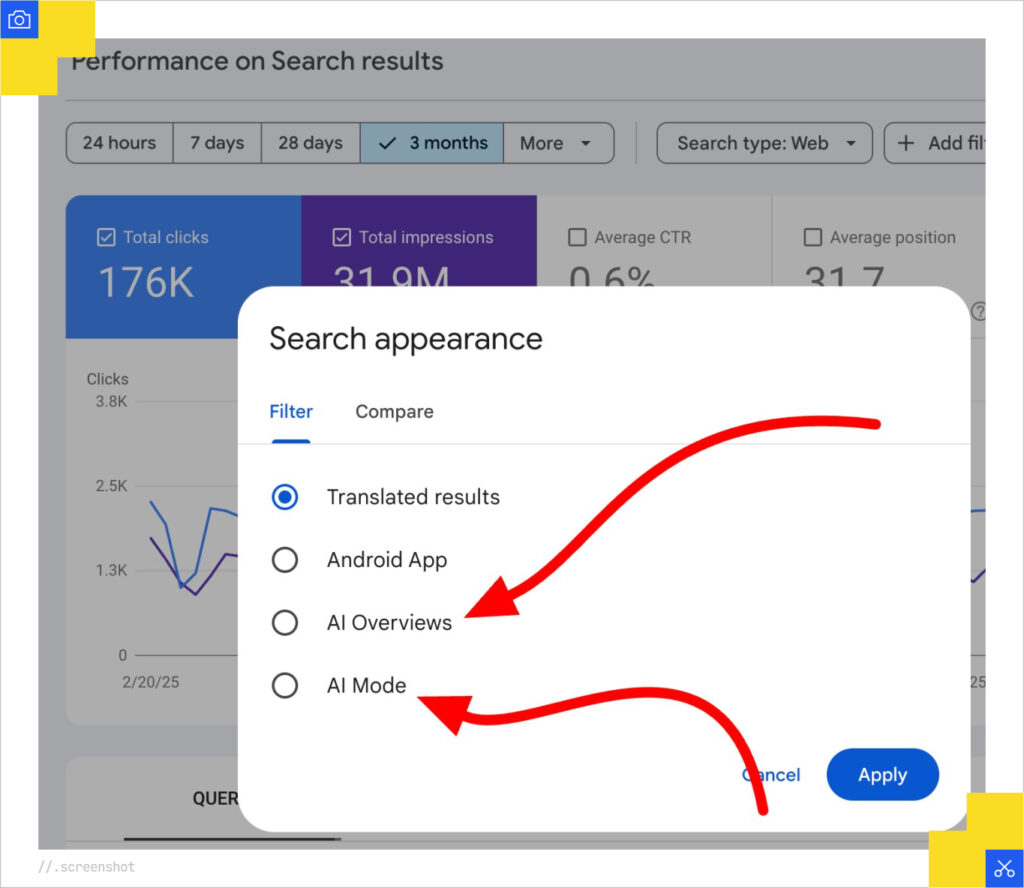
Image pulled from Barry Schwartz’s X feed
Here’s what we need specifically to make Google’s AI Search surfaces measurable:
- What is it: AI Specific reporting on visibility, citations, and frequency of appearance across generative surfaces.
- Why you need it: AI Mode is already reducing click-through rates, but we have no line of sight into whether we are still providing value or being fully bypassed. Google claims AI Overview data is in there, but there is no way to parse it out.
- How they should provide it: Segment-level reporting by AI surface (AI Overview, AI Mode, etc.) that includes citation heatmaps and passage-level usage data.
- How you can account for it now: You can’t. But you can scrape and monitor generative outputs at scale with tools like Profound or by building your own browser automation tools and string matching to track mentions.
How you can demand it: Hit the GSC feedback form.
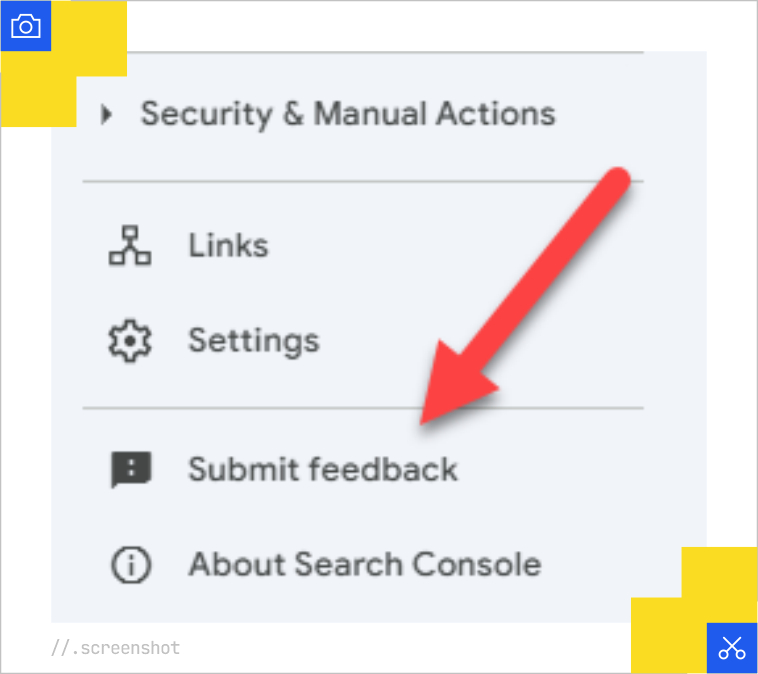
Logged-in Rank Tracking Based on Behavioral Personas
Rank tracking is still mostly rooted in a static understanding of universal rankings. But in AI Mode, rankings are dynamic, synthesized, and user-specific.
- What is it: Rank tracking for AI Mode based on synthetic queries and dynamic, user-personalized contexts (personas).
- Why you need it: AI Mode doesn’t show the same result to everyone. So, understanding ranking as a static position is functionally obsolete.
- How they should provide it: Rank modeling that includes intent class, user archetype, and classic organic position for core keyword and across fan-out queries.
- How you can account for it now: You can’t truly emulate it, but creating Google accounts and building up the context with Operator or a headless browser will allow you to create the persona. Then you’d run core queries across AI Mode and the synthetic query trees in classic organic and collect the data.
- How you can demand it: Profound is probably the closest to having something like this for AI Mode and AIOs. You should reach out to them to see what’s available.
Vector Embeddings for the Web
Vector Embeddings underpin everything in modern Google. Over the past few years, we’ve uncovered that the system creates vector representations of queries, pages, passages, authors, entities, websites, and now users themselves. At this point, this data is far more vital to our work than the link graph. Despite this, the SEO industry is still anchored in lexical scoring and keyword density, unable to access the semantic landscape that actually governs inclusion in AIOs and AI Mode. If we are to remain relevant, vector embeddings must become a foundational capability.
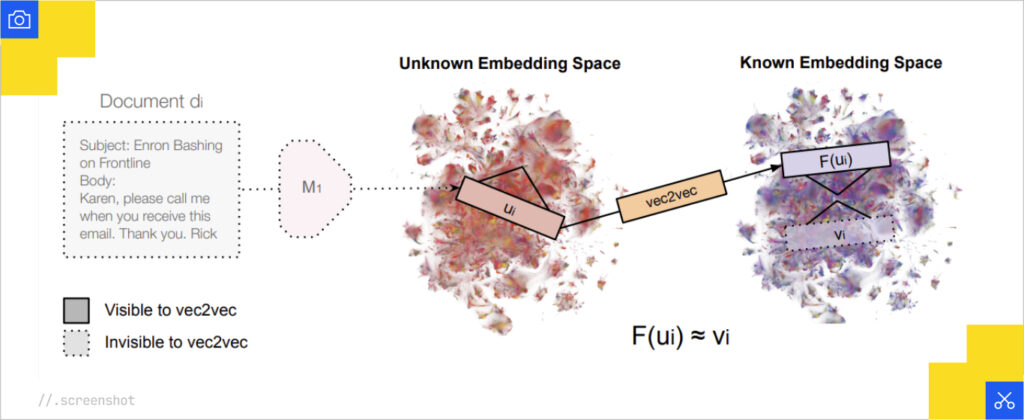
Just last week, a research paper entitled “Harnessing the Universal Geometry of Embeddings” was released, indicating that all vector embeddings ultimately converge on the same geometry. This suggests that at some point, we’ll be able to convert between embeddings, which means we will be able to generate open source embeddings and convert them into what Google is using.
- What is it: The mathematical representations in multidimensional space that are used in computations of meaning and relationships between entities, documents, websites, authors, and aspects of content.
- Why you need it: Google’s retrieval model is based on vector similarity. If you don’t understand how your content sits in vector space, you don’t understand how it will be retrieved or cited.
- How they should provide it: We need an embeddings explorer of the web that reveals site-level, author-level, page-level, and passage-level embeddings, for comparison across the web. We need tools that decompose your content into atomic assertions (triples) and score their retrievability and usefulness across fan-out queries. And finally, we need tools for content pruning based on site focus scoring in alignment with the data from the leak.
- How you can account for it now: Screaming Frog offers the ability to crawl and generate vector embeddings. However, to generate them on the passage level, you’ll need to write a custom JS function. Entities, authors, and websites require aggregation.
- How you can demand it: Contact support at your link data provider and ask why they don’t offer this.
Matrixed Semantic Content Editors
Content creation for AI Mode is often not a single-page task. You are now competing across a matrix of synthetic queries, reasoning steps, and passage-level comparisons. That means content needs to be engineered across clusters, not just optimized in isolation. Yet SEO content editor tools only let you edit content against a single keyword target based on the lexical model. The future demands an interface where content optimization happens across multiple surfaces and subqueries simultaneously, with dense retrieval in mind.
- What is it: A content editing tool that gives you the ability to analyze and engineer content across a query cluster in one interface.
- Why you need it: There are many SEO content editors. Most of them operate only on sparse retrieval techniques (TF-IDF/BM25). RAG pipelines operate in large part on dense retrieval techniques. Some use hybrid retrieval. None of the major ones are using sparse retrieval as their primary method
- How they should provide it: A content editing UI that surfaces passage-level matching against query clusters, with embeddings and ranking overlap visualized.
- How you can account for it now: You’d have to build your own, but it’s easier to just… hire us.
How you can demand it: Contact support at your content editor tool and ask when they expect to modernize their solution.
Query Journeys
Search is no longer a one-shot decision. It’s a session-driven sequence of related questions, many of which are generated by the system itself. Query fan-out, DeepSearch, and reasoning chains all reflect this evolution. But many keyword research tools still assume isolated queries, ignoring the order in which users interact with topics. Understanding how queries evolve over time is essential to engineering influence across a user’s decision journey.
- What is it: The ordered sequences of user queries from clickstream data.
- Why you need it: Understanding multi-query behavior is essential for engineering visibility across decision journeys.
- How they should provide it: Sequences of keyword data with user attributes.
- How you can account for it now: You’d have to get a subscription from a company like Datos and stitch the data together yourself.
- How you can demand it: Contact support at your keyword research tool and ask when they will incorporate clickstream data for query journeys.
Personalized Retrieval Simulations
With user embeddings becoming central to how Google personalizes results, relevance is no longer universal. Two people asking the same question may see entirely different answers. The current model of rank tracking assumes a static user profile, which fails in this context. What we need instead are tools that simulate how our content performs against different behavioral personas so we can engineer for visibility across varied user contexts, not just a hypothetical average.
- What it is: Modeling how user embeddings affect retrieval in AI Mode.
- Why you need it: Google is increasingly shaping answers based on who is asking, not just what they’re asking. Without understanding how your content performs across user types, you’re overfitting to a phantom “average” user.
- How they should provide it: A UI powered by embeddings that represent user personas, each with different memory profiles that allow you to test your content corpus versus competitors.
- How you can account for it now: Simulate retrieval against modified embeddings or prompt contexts using open-source LLMs and variable memory inserts.
- How you can demand it: MarketBrew probably has the closest solution for this; you should ask how they are approaching this.
Query Classification
Query Classification in major SEO tools is basic. Typically, they are giving you a modified but out-of-date version of Andrei Broder’s navigational/informational/transactional taxonomy. It’s out of date because Broder has since added “hedonic” to the list. However, Mark Williams-Cook revealed that Google’s internal classifications are much more actionable.
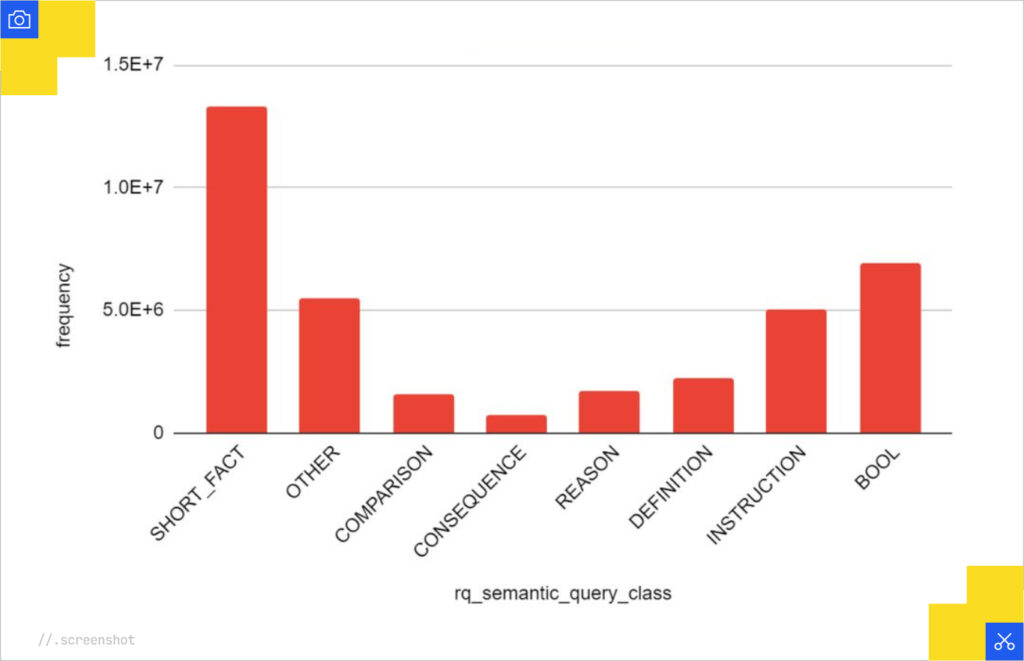
I suspect the internal classification helps with determining which features to include in AI Mode.
- What is it: Assigning Google’s internal query classifications user intent types (short_fact, reason, et al) to queries using ML and LLMs.
- Why you need it: AI Mode classifies queries to determine answer format, model selection, and trigger templates. Your content must match format and intent type.
- How they should provide it: Classification based on Google’s internal taxonomies, with support for multi-label intent.
- How you can account for it now: Use Mark’s classifier as part of your keyword research process.
- How you can demand it: Ask Mark to make an API so everyone can use this data at scale.
Query Expansion
Query fan-out rewrites the nature of visibility. Any modern SEO workflow must incorporate query expansion simulation as a baseline input to content planning and performance modeling.
- What is it: Generation of related, implicit, comparative, and recent subqueries by the system to power retrieval.
- Why you need it: There has always been some form of query expansion because a query like [gm car] is “General Motors car,” but you would likely not get the right results unless you included General Motors in the background. Hummingbird (e.g. Word2Vec) took care of this so we didn’t have to think about it. In the case of AI Mode, if you don’t rank for these, you don’t get considered, regardless of your performance for the head term.
- How they should provide it: A matrix of synthetic queries with visibility scoring, tied to primary head queries.
- How you can account for it now: Use tools like Qforia or Gemini prompt-chains to simulate fan-out, then embed and compare.
- How you can demand it: Contact support at your keyword research tool and send them this post.
Clickstream Data
AIOs and AI Mode data are not directly visible in GSC, and many generative results don’t drive clicks at all. This severs our ability to understand performance through traditional web analytics. Clickstream data becomes essential as a proxy for user behavior. It offers visibility into what users see, what they choose, and what they bypass, even in zero-click environments. SEO tools need to integrate this external signal to restore observational power in a space where direct attribution is disappearing. Re-ranking is also triggered by click behaviors; SEO software should provide a sense of the click models based on this data.
- What is it: Aggregated user behavior data capturing real-world traffic flows and click sequences.
- Why you need it: Without GSC data, clickstream may be your only view into traffic paths and post-click behavior.
- How they should provide it: Integration with clickstream providers like Similarweb or Datos, mapped to organic and AI surfaces.
- How you can account for it now: Stitch it manually with clickstream data and inference models of SERP type.
- How you can demand it: Contact support at your keyword research tool and send them this post.
Reasoning Chain Simulation
AI Mode’s logic isn’t linear; it’s inferred. Answers are built through chains of reasoning steps that span multiple passages and content types. Success means having your content selected to support one of those steps. But unless you simulate the reasoning chain, you don’t know if your content is useful to the machine’s thinking. Tools need to let us replicate this process, so we can test not just “does my content rank?” but “does my content help the model think?” and “where does my content fall out of the reasoning chain?”
- What it is: The ability to simulate how a system like Gemini builds a response via intermediate logical steps using Chain-of-Thought prompting and passage-level synthesis.
- Why you need it: Visibility in AI Mode depends not just on having good content, but on having passages that support steps in a machine’s reasoning. Without simulating that process, you have no idea if your content is usable.
- How they should provide it: Simulated reasoning flows per query cluster, with citation mapping to content and feedback for when you fall out of the pairwise reasoning
- How you can account for it now: Build your own using LlamaIndex, Chain-of-Thought prompts, and a vector store of your own site’s passages.
How you can demand it: MarketBrew probably has the closest solution for this; you should ask how they are approaching this.
Relevance-Based Link Graphs
Although the role of links is heavily deemphasized in these patents, I still believe in the importance of PageRank and its various forms. Despite obvious changes to how Google views the link graph, there hasn’t been any meaningful movement from link data providers in a very long time. At the very least, they should provide relevance scores between source and target documents. They should also be leveraging clickstream data and rankings to get a sense of where content lives in the index, since we now know that that impacts the value a link has to pass.
Dare I say that the link graph, as we have it, has become…not so interesting due to the gaps in the data. The link indices could be completely revitalized and significantly more valuable by becoming the providers of the embeddings data.
- What it is: A next-gen link analysis system that scores links not just by authority, but by semantic alignment, retrievability, co-citation behavior, and inferred index position.
- Why you need it: Google’s use of link data has evolved. Link equity is now entangled with retrieval patterns and passage relevance.
- How they should provide it: Document-level and passage-level relevance scores between source and target, using dense embeddings and clickstream modifiers.
- How you can account for it now: Build a system using Screaming Frog, Ollama embeddings, and Gephi to visualize and score your internal and external link graph, but good luck crawling the whole web this way.
- How you can demand it: Contact support at your link provider and send them this post.
Aside from what we need from Google, that is ten things that the SEO software industrial complex should be racing to incorporate into their solutions. These aspects are not just relevant to the inevitable future of AI Mode becoming the default, but are relevant to AI Overviews right now. Use your voice and push your software providers to alter their products in support of features that can actually help you get a result.
Rethinking Search Strategically for the AI Mode Environment
AI Mode represents a structural transformation in the search landscape. What began as enhancements to the SERP has now become a self-contained ecosystem of conversational, multimodal, and memory-informed retrieval. The conventional SEO paradigm, built on explicit queries, deterministic ranking, and click-based performance attribution, is no longer sufficient.
Just as AI Mode is an expansion of AI Overviews, we can expect user behavior to follow similar but even more compressed patterns. The best analog is probably ChatGPT or Perplexity: environments where users engage in low-friction, high-trust interactions and receive fully synthesized answers with little to no click behavior. That means organic search in AI Mode behaves more like a zero-click branding channel than a traditional performance one.
But unlike Overviews, AI Mode introduces multiple dimensions that fundamentally change what it means to “show up.” So the strategy must shift. This isn’t just about ranking anymore; it’s about earning inclusion in the candidate corpus and winning passage selection.
The first decision is simple: do you want to be there?
It might sound crazy, but this may be the moment that your organization or client abandons the channel as one that they proactively manipulate. A subset of users will continue to go back to classic search. If you’re doing well there, you may not care so much about AI Mode. Perhaps your overall channel mix is just fine, and/or you’re finding better incrementality elsewhere.
From a strategic standpoint, this shift necessitates a fundamental reframing. Organizations must stop optimizing solely for traffic and begin competing for machine-mediated relevance. Success in AI Mode is not a function of surface-level rankings but of embedding alignment, informational utility, and latent inclusion in systems of reasoning. The strategic implications fall across three domains: channel reclassification, capability transformation, and data infrastructure modernization.
Reclassify Search as an AI Visibility Channel
Historically, Organic Search has operated as a hybrid performance/brand channel. Roughly 70% attributable to performance-driven user actions and 30% to brand reinforcement. In the AI Mode paradigm, that balance will likely invert.
Search should now be reframed as a visibility and trust channel mediated through large language models. The organization’s goal shifts from driving traffic to being selected as a source. This demands a new KPI structure:
- Share of voice within AI surfaces
- Sentiment and citation prominence in generative responses
- Attribution influence modeling over deterministic last-click attribution
Leaders must realign budget allocations, stakeholder expectations, and measurement frameworks accordingly. It is no longer about appearing for a keyword; it is about being encoded into the model’s understanding of the information domain.
Build Relevance as an Organizational Capability
In a generative retrieval ecosystem, the source of competitive advantage is not content volume or link velocity; it is the systematic engineering of relevance across vector spaces.
This requires new capabilities:
- Semantic Architecture – Structuring knowledge assets to be machine-readable, recombinable, and contextually persistent.
- Content Portfolio Governance – Treating keyword portfolios and content assets like financial instruments: diversified, performance-monitored, and pruned for relevance decay.
- Model-Aware Editorial Strategy – Designing content not just for users, but for agents: optimizing for LLM interpretation, citation, and embedding distance from competitors.
Forward-leaning organizations will invest in teams that combine SEO, NLP, data science, UX, digital PR, and content strategy operations into an integrated Relevance Engineering function. This unit becomes the connective tissue between brand, product, and AI visibility.
Operationalize Intelligence in a Post-Click World
The collapse of the click as a primary performance signal leaves organizations flying blind unless they modernize their data strategy to include machine-consumable relevance metrics and generative surface analytics.
Strategic imperatives here include:
- Simulation Infrastructure – Stand up internal LLM evaluation pipelines (RAG, LlamaIndex, etc.) to simulate brand visibility in AI responses and train relevance metrics.
- Citation Intelligence Platforms – Track when, how, and why brand assets are cited in AI systems, even in zero-click environments.
- Content Intelligence – Invest in infrastructure that unifies passage-level embeddings, knowledge graph coverage, and content performance across classic and generative retrieval systems.
Executives must be open to dashboards beyond reflections of past user behavior and toward systems that surface where the organization exists in the model’s latent space, where it is understood, trusted, and re-used by AI agents on behalf of users. In other words, branding is in addition to performance.
Strategic Positioning: From Performance to Participation and Optimization to Orchestration
Ultimately, the AI Mode environment demands a shift from search as transaction to search as participation. The question is no longer “how do we rank?” but “how are we represented in AI cognition?”
This is the emergence of a new corporate function: Relevance Strategy. Aligning with Relevance Engineering through the deliberate, cross-functional coordination of a company’s presence in algorithmic decision-making systems. Organizations that succeed here will be those that treat visibility not as a campaign outcome, but as a strategic asset to be architected, measured, and governed.
Unfortunately, Not Everyone is Coming With Us
SEO in the AI Mode world is no longer about chasing blue links. It’s about building robust, retrievable, and reusable content artifacts that serve as input for machine synthesis. That requires a mindset shift from tactical optimization to strategic orchestration across queries, formats, and embeddings.
Relevance Engineers will lead this transition. They will be the ones who not only understand how the systems work, but who build workflows, training sets, and tools that keep brands visible even in a world without SERPs.
Like it or not, we are in a new era of Search. The relationship between user and search engine has changed just as the relationship between search engines and websites has changed. We can sit here and argue about what it is or isn’t. Or, we can redefine our capabilities and software based on what conversational search is actually headed.
So, who’s coming with me?