I didn’t know what was in store, but was ready to document everything. It was the first SEO Week. The iPullRank team was prepared. The event space was designed like no other SEO conference. To say the first day was technical would be an understatement. To the geeks!
We launched the week with The Science of SEO. Attendees immediately vibed as they took in the blue cobalt of the neon lights and the technology theme projection mapped across the venue’s walls. We were ready. Chef’s kiss.
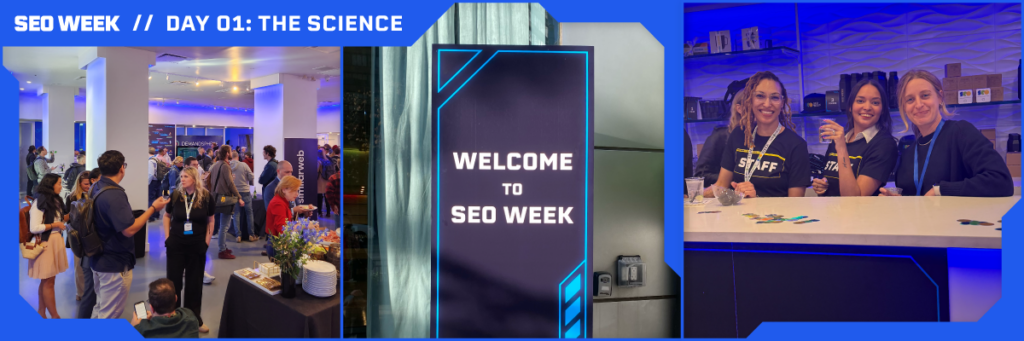
As someone who’s been in marketing for 20 years and in SEO for 13 (mostly on the content side of things), I’ll be real – some of the stuff shared this first day was way above my pay grade. But it was fascinating, inspiring, and a little humbling in the best way.
So if you’re like me, curious but maybe not fluent in vectors and embeddings just yet, don’t worry; I got you. I’ll walk you through each session, share the biggest takeaways, and drop a little glossary at the end of each section to help decode the techy bits. Let’s get into it.
Jump to the speakers
Download all of the decks from our SEO Week day one speakers
The Brave New World of SEO: Relevance Engineering
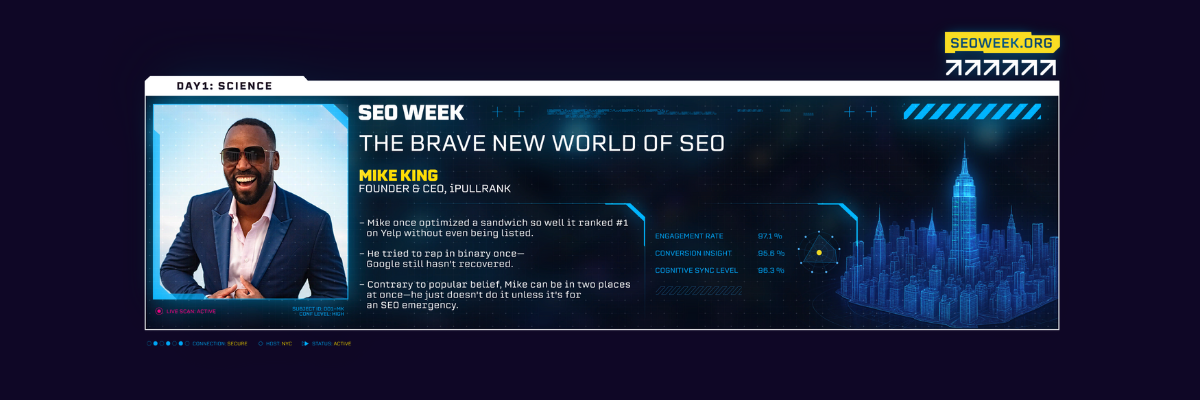
Mike King, Founder and CEO of iPullRank, launched the conference with his presentation, declaring that the entire search engine optimization industry is fundamentally changing. SEO practitioners need to accept that the traditional organic search results page of 10 blue links has become a relic; SEO is facing a fundamental shift with Google’s introduction of AI-driven features like AI Overviews and AI Mode.
Organic traffic is declining permanently, forcing marketers to reconsider how they measure success, moving beyond traffic to quality and relevance.
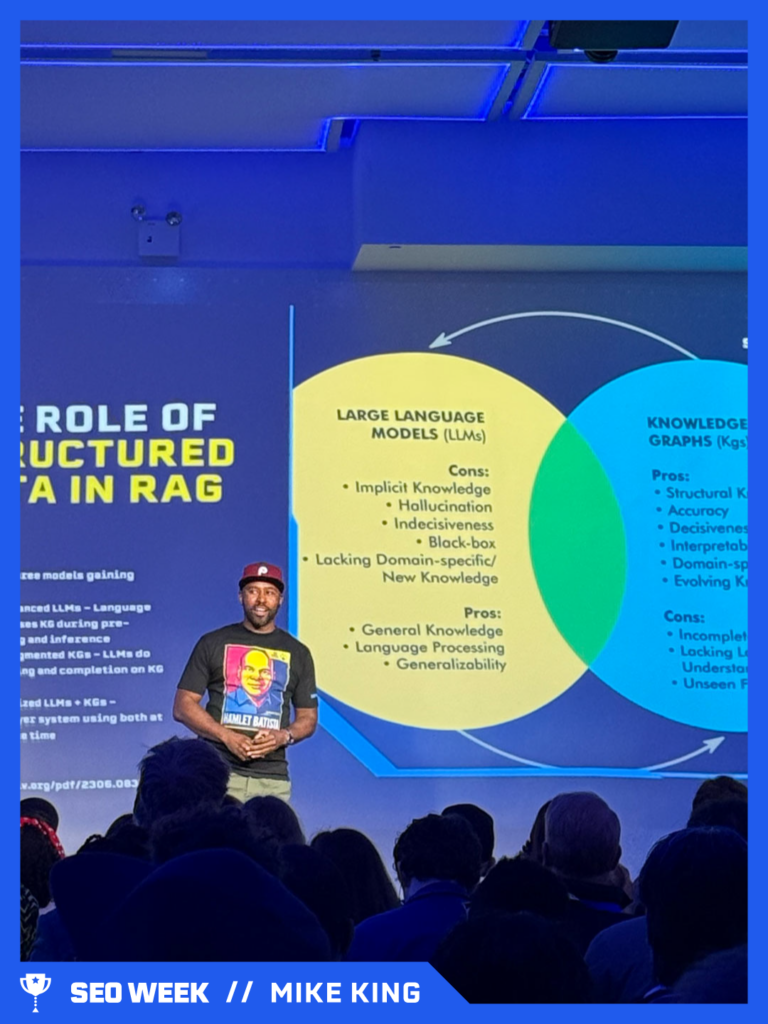
“SEOs are the janitors of the web.”
- Mike King
Key points
- AI Overviews and AI Mode: Google’s new search formats drastically reduce the need for users to click through to sites by summarizing answers directly in search results, significantly reducing traditional traffic. Unlike Featured Snippets, these tools profoundly impact user behavior and visibility.
- Delphic Costs: Google is intentionally reducing the cognitive effort of search by providing immediate, direct answers, thus training users to expect longer, conversational queries.
- Hyper-personalization: Google’s use of AI memory features personalizes search experiences to an unprecedented level, challenging traditional SEO practices.
- Vector Embeddings & Cosine Similarities: Content relevance is now mathematically determined using vector embeddings, which plot content and queries in semantic spaces. Websites must ensure their content is semantically clustered and closely related to targeted queries. Irrelevant “floating” content will fail to gain visibility.
- Relevance Engineering: The traditional role of SEO – optimizing for search – is outdated. Instead, Relevance Engineering proactively structures content and experiences around clear semantic and mathematical frameworks, aiming for high relevance and visibility across all search modalities.
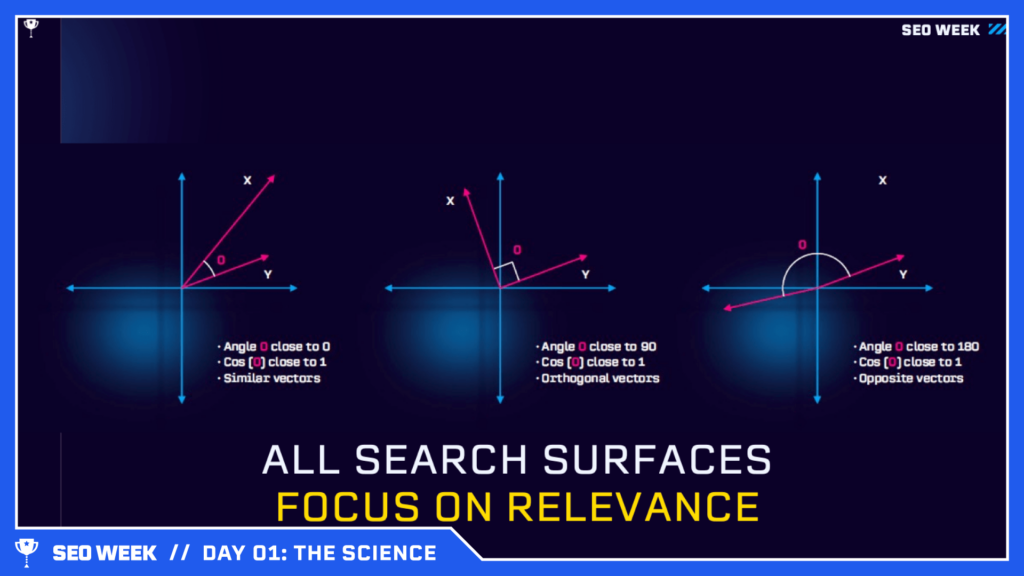
Practical steps for implementing Relevance Engineering
- Identify Relevant Audiences and Channels – Clearly define who your audience is and choose channels based on where your audience actively searches.
- Configure Platforms – Ensure technical setups align precisely with the specific search surfaces (Google AI Overviews, conversational searches, etc.).
- Engineer Relevant Experiences – Create content explicitly structured into semantic chunks, leveraging semantic triples (clear subject-predicate-object relationships) to boost search retrieval accuracy.
- Example (Ineffective): “SEO helps businesses get more traffic.”
- Example (Effective): “SEO (subject) increases (predicate) organic traffic (object) for businesses.”
- Develop Co-relevant Experiences – Foster meaningful connections across related web content to improve authority and relevance signals.
- Measure and Validate – Use scientific methods such as A/B testing and reproducible experimentation to measure success and refine tactics based on peer-reviewed, community-driven best practices.
Important Takeaway
Search Engine Optimization must evolve into Relevance Engineering, a scientifically rigorous, highly intentional discipline focusing on semantic precision, structured content, and proactive relevance across all search modalities. Traffic volume alone is no longer the goal; qualified engagement through precise, intentionally structured content is the future of search.
Mini Glossary
- AI Overviews: Google’s AI-generated search results provide concise, direct answers without traditional links.
- Vector Embeddings: Mathematical representations of content that measure semantic relationships numerically.
- Cosine Similarity: A measure of semantic similarity between two vectors; the closer the vectors, the higher the relevance.
- Semantic Triples: Structured expressions clearly stating a subject-predicate-object relationship, aiding search engines’ understanding of content.
- Relevance Engineering: A new SEO paradigm focusing on engineering mathematically precise content relevance rather than traditional optimization.
Relevance Engineering prioritizes understanding user intent, creating aligned experiences, and leveraging advanced information retrieval techniques. It’s a holistic approach to Organic Search.
With iPullRank spearheading initiatives like a Relevance Engineering website, the future of SEO depends on building intelligent, audience-first systems rooted in data, performance, and open-source innovation.
Read more:
Want to implement Relevance Engineering for your business?
From AI to Z: The Technical Evolution of Search Engines
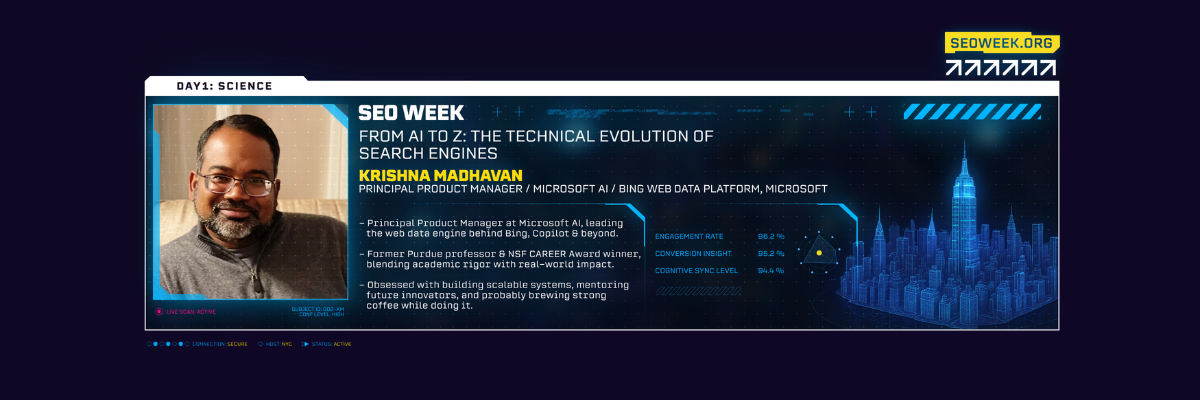
Principal Product Manager, Microsoft AI, Bing Web Data Platform at Microsoft Krishna Madhavan memed up his technical presentation with generative AI images of his cats. But don’t be fooled, it was a sophisticated topic.
Working on the front lines of Bing, he explained how search is no longer simple. It’s dynamic. It’s now shaped by AI, quantum computing, and user-centered relevance. AI-driven algorithms now understand user intent, predict needs, and initiate actions, transforming simple queries into personalized conversations.
Websites prioritizing user relevance, authenticity, and trustworthy content will outperform in this new landscape.
“All LLMs have a knowledge cutoff date. Fresh data and content is the fuel that powers AI.”
- Krishna Madhavan
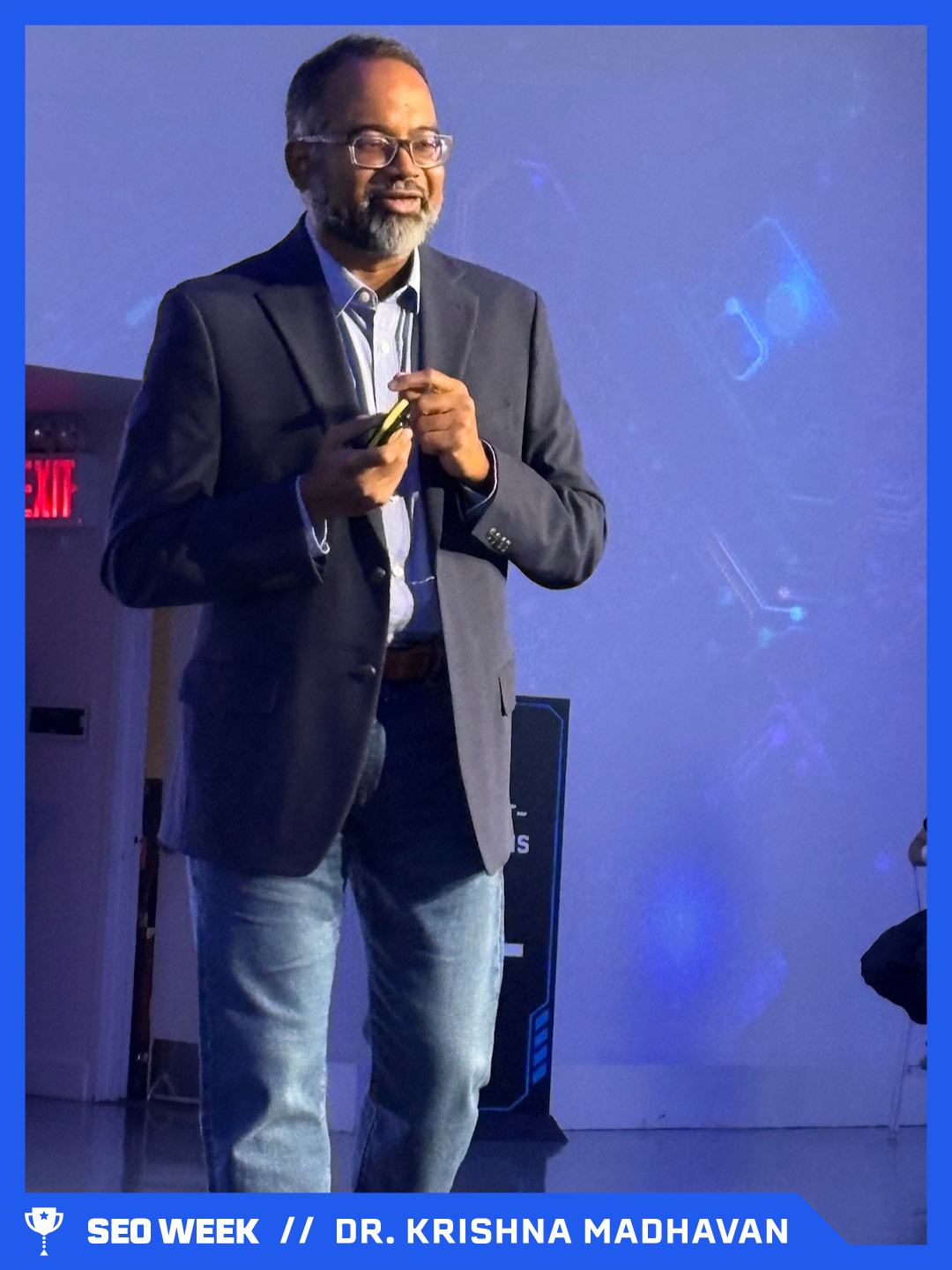
Key points
- Outcomes Over Clicks: Success in search is now measured by real user outcomes (e.g., transactions, subscriptions), not just visits.
- Importance of LLMs: LLMs enhance search by accurately interpreting queries and content through smarter indexing, query expansion, natural language processing (NLP), context-aware content, and improved content summaries – see Bing Chat’s recent improvement in relevance using LLM technology as an example.
- Generative AI’s Growth: Generative AI is rapidly improving search accuracy and personalization, speeding up tasks like summarizing complex data, recognizing trends, and automating routine actions.
- Fresh Data Is Crucial: Since LLMs have a fixed knowledge cutoff, continuously updated content is critical. Integrating fresh data through Retrieval Augmented Generation (RAG) ensures AI outputs are timely and relevant.
- Actionable tip: Use protocols like IndexNow to get your new content immediately indexed by search engines.
- Quantum Computing on the Horizon: Although still distant, quantum computing could dramatically enhance search capabilities, enabling simultaneous analysis of billions of possibilities, instantly updating data across multiple locations.
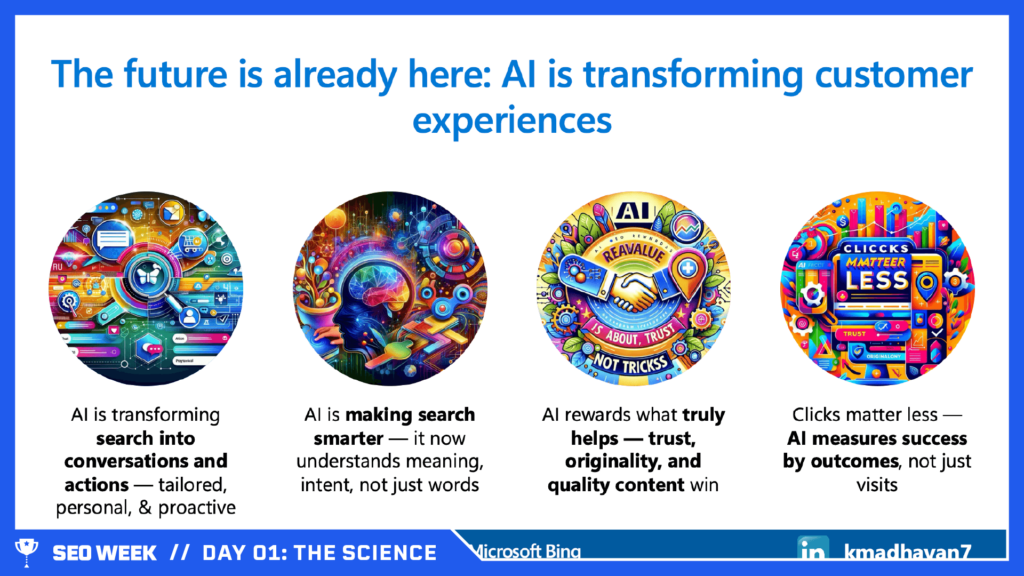
Important Takeaway
SEO is shifting from optimizing content purely for rankings to creating authentic, meaningful user interactions supported by advanced AI technologies. Ensuring your content remains fresh, relevant, and user-centric is essential for continued visibility and success.
Mini Glossary
- Large Language Models (LLMs): AI models trained on extensive datasets capable of understanding and generating human-like text.
- Natural Language Processing (NLP): Technology enabling computers to interpret and respond to human language naturally.
- Retrieval Augmented Generation (RAG): AI method combining live search results with pre-trained knowledge to generate accurate, current responses.
- IndexNow: Protocol allowing real-time notification of new or updated web content directly to search engines for immediate indexing.
- Quantum Computing: An Advanced computing method using quantum bits (qubits), enabling rapid parallel processing and enhanced computational power.
With technologies like Retrieval Augmented Generation, grounding LLM output in facts, IndexNow keeping Microsoft’s indexes more efficiently up to date, and neural networks mimicking the human brain, we’re entering a phase of SEO where content quality and data freshness drive success.
The future of search lies in real-time, multimodal, quantum-powered systems designed for intelligent, human-like engagement.
Authoritative Intelligence: Evolving IR, NLP, and Topical Evaluation in the Age of Infinite Content
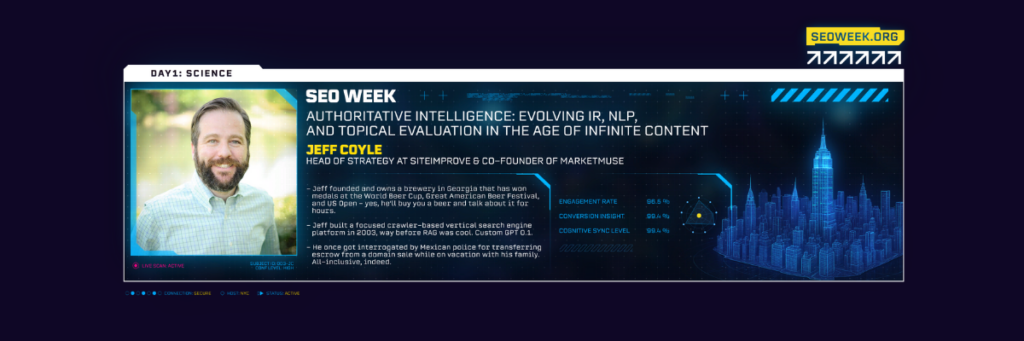
Head of Strategy at Siteimprove & Co-founder of MarketMuse Jeff Coyle framed the changing nature of search engines differently. He called out how keyword-based tactics are too basic.
We must consider sophisticated information retrieval rooted in natural language processing, vector search, and topic authority. That’s how the algorithms process content – there’s a shift from keyword-based content strategies to meaningful, authoritative content evaluation driven by advanced search technologies.
“The person who covers the entire JOURNEY is the real expert.”
- Jeff Coyle
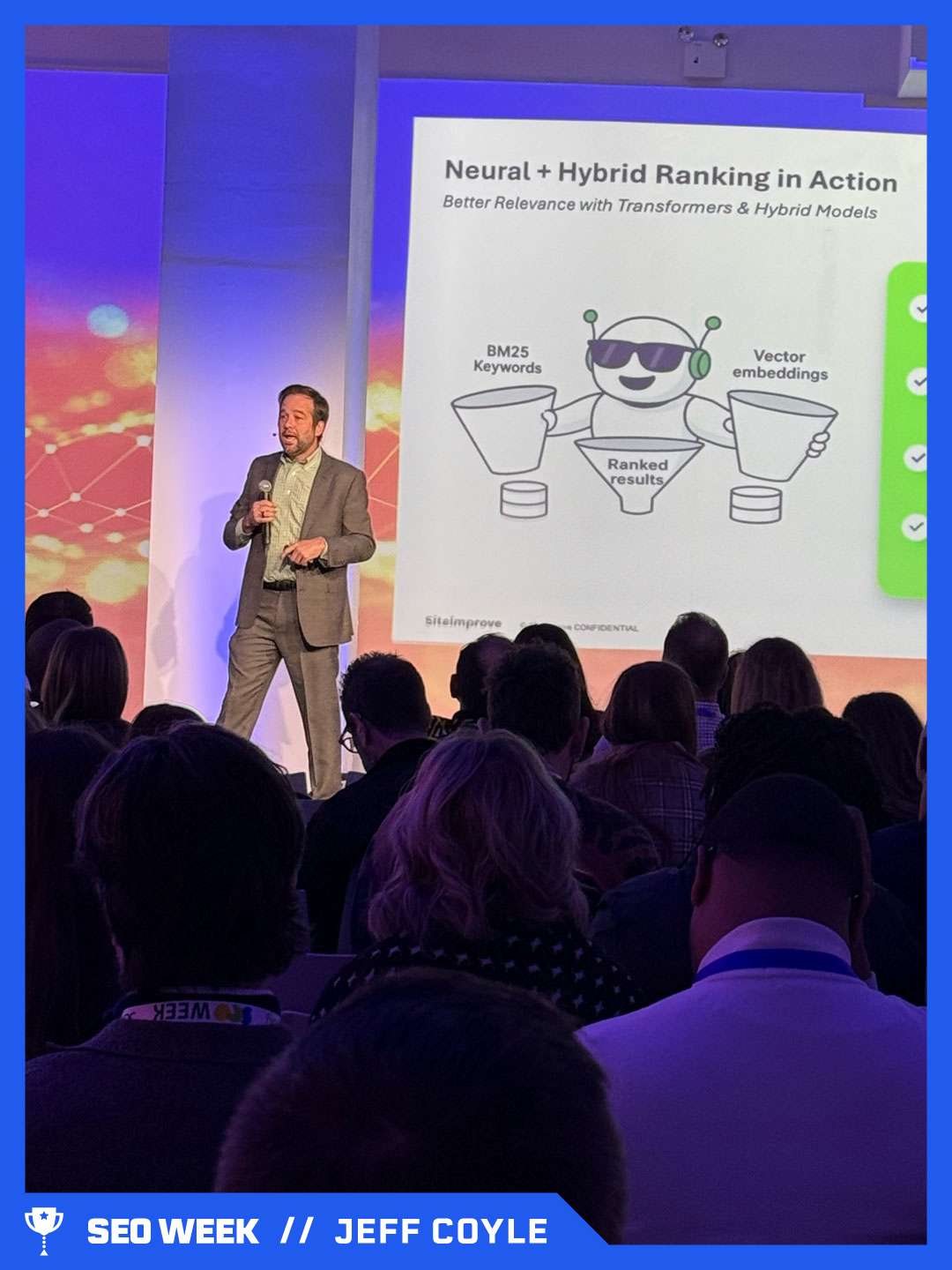
Key points
- Infinite Content Challenge: AI-driven workflows now produce vast amounts of mediocre content, raising the importance of distinguishing authoritative content.
- From Keywords to Context: Traditional keyword matching evolved into more sophisticated retrieval methods, including vector search and hybrid retrieval. For example, semantic vector search maps queries and content into numerical forms, improving relevance even when phrasing differs.
- Generative Information Retrieval: Modern search engines don’t merely return documents but synthesize information using Retrieval-Augmented Generation (RAG) systems. For instance, a query about a Mexican lager could return either a simple factual response or an extensive synthesis depending on the query’s complexity.
- Trust and Bias Management: Ensuring factual grounding, accurate citations, and managing biases (hallucinations and misinformation) through alignment and guardrails is essential.
- Editorial Excellence and Point of View: Coyle emphasizes the need for content with a distinct perspective. “You can’t edit your way to excellence,” he argued, advocating for proactive developmental editing to ensure clarity, nuance, and authoritative depth.
- Impact on SEO and Content Strategy: SEO strategies must now account for query-dependent nuances and user journeys, prioritizing content that provides comprehensive, trustworthy answers rather than just achieving high click rates.
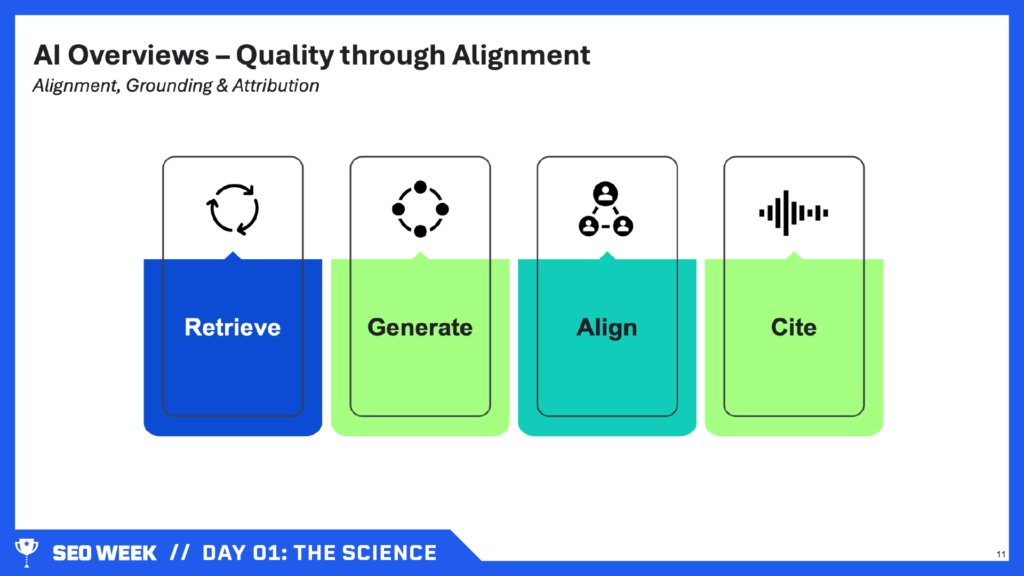
Important Takeaway
Authoritative content must go beyond mere keyword relevance; it must deeply understand and effectively address complex user queries through trustworthy, nuanced, and differentiated perspectives.
Mini Glossary
- Vector Search: A search method using numerical representations (vectors) of queries and documents to identify semantic relevance beyond exact keywords.
- Hybrid Retrieval: Combines keyword-based (lexical) search methods with semantic vector-based methods for improved relevance.
- Retrieval-Augmented Generation (RAG): AI systems retrieve relevant documents and then generate synthesized, contextually informed responses.
The challenge is no longer publishing content at scale, but earning trust through relevance, nuance, and reliability. With AI-driven, agentic workflows enabling massive content creation, site-level evaluations, and journey-based expertise now define quality.
NEGSEO - SEO for the Bad Kids
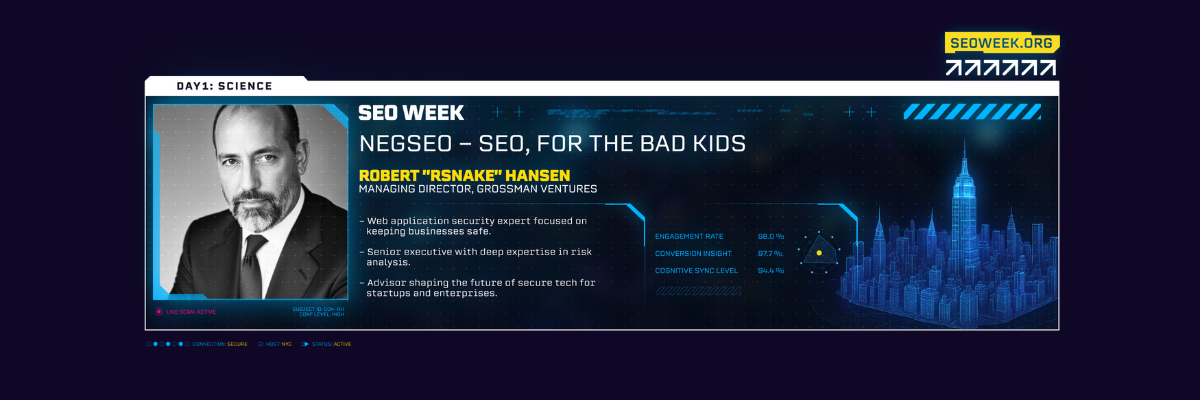
RSnake, a cybersecurity expert, focused his SEO Week presentation on Negative SEO (nSEO), a strategy of sabotaging competitors rather than improving your own rankings. He argued that Google suffers from confirmation bias, meaning they rarely investigate reports of nSEO attacks because they assume these issues are uncommon or false alarms.
The goal here isn’t to outrank competitors by improving your own site, but by deliberately sabotaging others. This “hierarchy of negative” approach exploits Google’s confirmation bias, which often overlooks such tactics.
Together, these talks captured the shift from traditional SEO metrics and tactics toward a more integrated, strategic approach—one that blends technical expertise, brand vision, and a deep understanding of evolving digital behaviors.
Across all sessions, one unifying theme emerged: The future of SEO is no longer about keywords; it’s about knowledge. It’s about showing up in the AI conversation, being understood by machines, and meeting users wherever their journey takes them – be it traditional search, voice, chat, or visual discovery.
"You can do really cool things, like old school cloaking techniques, to change the content in all kinds of interesting ways that might make the LLMs think things are true that aren’t true, or get them to think different things than what Google’s thinking.”
- RSnake
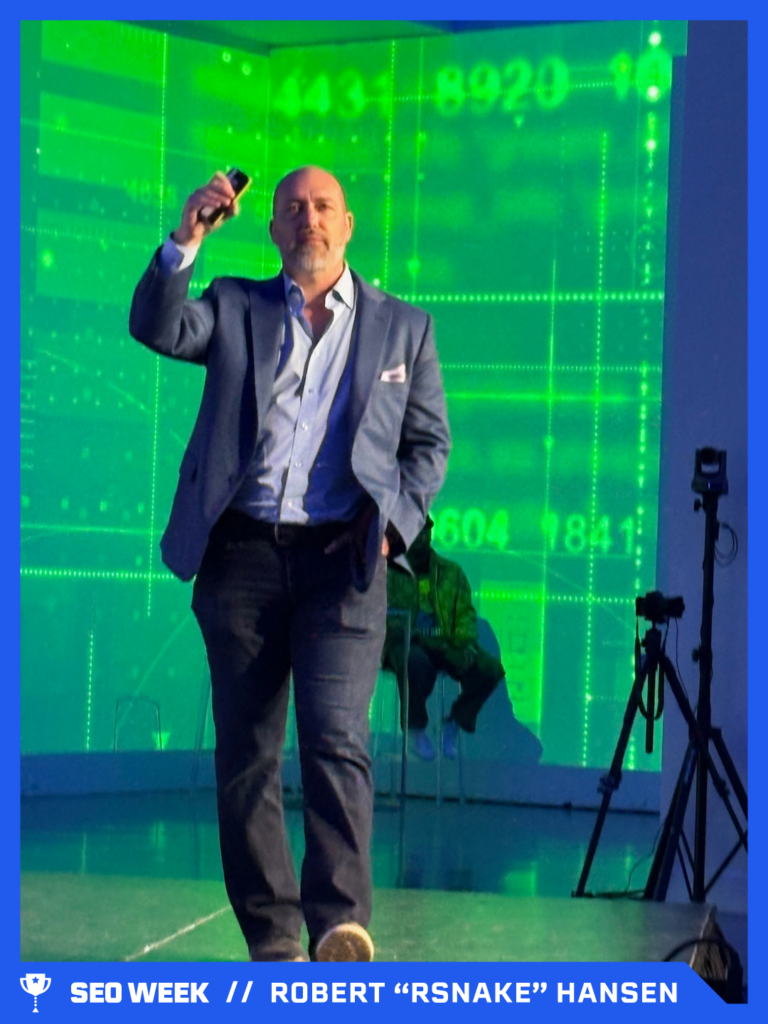
Practical nSEO tactics: Don't try this at home
- Fake DMCA notices: Getting pages removed unjustly.
- Spammy Autocomplete Suggestions: Associating competitor brands with negative keywords like “fraud” or “illegal.”
- Blacklist Sign-ups: Submitting competitor sites to RBL (Real-time Blackhole List) spam lists.
- Obscene Denial of Service (DoS): Overwhelming competitors with inappropriate communication requests.
- Fear, Uncertainty, Doubt (FUD) Articles: Publishing negative press to damage reputations.
- Spoofing Emails: Faking communication between a competitor’s SEO and IT teams to cause internal issues.
- Mass Negative Reviews: Coordinated review bombing.
- Clickjacking: Hijacking user clicks to trigger unwanted actions.
- Overloading AI Features: Flooding competitor websites with expensive chatbot or LLM requests.
- Parasitic Hosting: Exploiting security weaknesses like open file uploads to compromise sites.
- Injection Attacks (SQL and CMD): Gaining unauthorized database or server access.
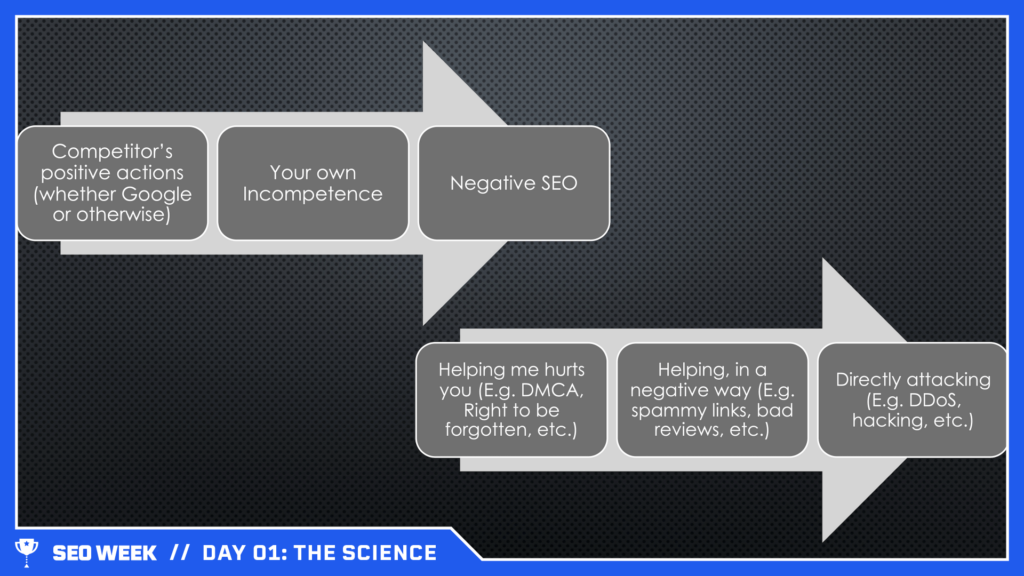
Important Takeaway
Google rarely acknowledges or investigates negative SEO effectively, leaving many sites vulnerable. Companies must proactively monitor and defend their digital presence to mitigate these risks.
Mini Glossary
- Confirmation Bias: The tendency to interpret new evidence as confirmation of one’s existing beliefs or theories.
- DMCA: Digital Millennium Copyright Act; legal measure used to remove allegedly infringing content from the web.
- RBL (Real-time Blackhole List): Lists used by email services to identify and block spam sources.
- DoS (Denial of Service): Attack aimed at making services unavailable by overwhelming them with traffic or requests.
- Clickjacking: Trick users into clicking something different from what they perceive, typically to steal information or direct them elsewhere.
- SQL Injection (SQLI): Exploiting vulnerabilities to manipulate databases.
- Command Injection (CMDI): Exploiting vulnerabilities to execute arbitrary commands on a server.
Negative SEO – instead of worrying about me going up, I just make everybody go down, so I’m the highest. Hierarchy of positive and hierarchy of negative.
The Rise of the SEO Data Scientist
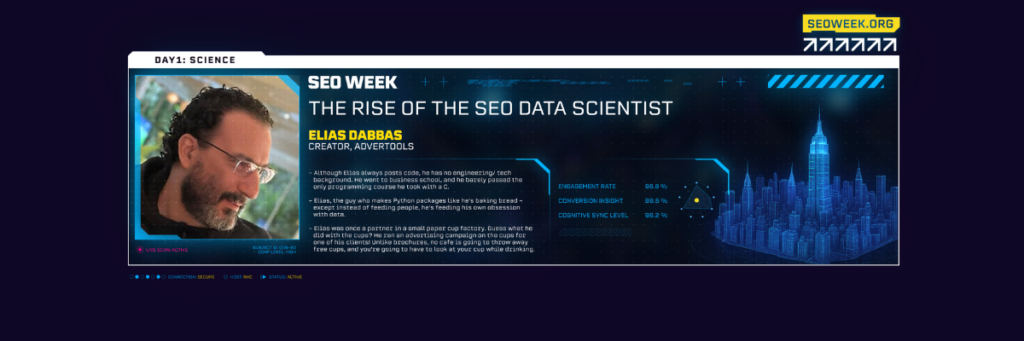
Elias Dabbas, author of Interactive Dashboards & Data Apps with Plotly & Dash showcased how SEO professionals can level up as data scientists by combining data engineering, automation, and prompt design to analyze and scale SEO efforts using his Python package, advertools.
Elias developed advertools to streamline SEO data science tasks by combining automation, analysis, and structured insight generation within a single, customizable toolkit. He emphasizes that true data science goes beyond just learning Python – it requires understanding core principles, from variable logic to visualization and contextual application.
“When some field is just getting started and you don't really understand it very well [Data Science], it's very easy to confuse the essence of what you're doing with the tools that you use [Python]."
- Elias Dabbas
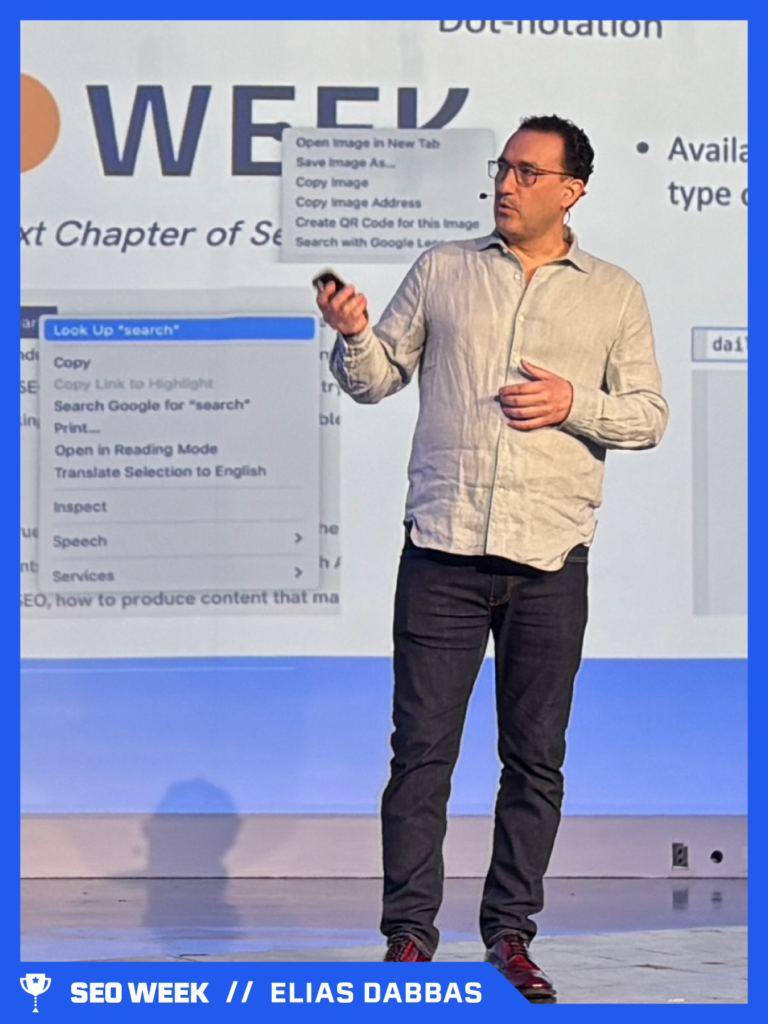
Key points and advertools capabilities
- Principle over Tools: Don’t confuse learning Python with learning data science. You need to understand how to define variables, build functions, and visualize data to extract insights, not just automate tasks.
- advertools as a Utility Belt:
- Converts robots.txt and XML sitemaps into filterable dataframes.
- Analyzes user-agent access, URL patterns, redirects, and blocks.
- Provides reproducible outputs, enabling scalable audits over time.
- Example: Crawl a domain and extract structured metadata (e.g. OpenGraph, JSON-LD) and all headers in one .jsonl file.
- URL Analysis with Python:
- adv.url_to_df() transforms a batch of URLs into a dataframe.
- Enables splitting, segmenting, and counting patterns in URL paths at scale.
- Prompt Engineering at Scale:
- Treats prompts like code: use templates with dynamic variables (e.g. {product_model}, {price}).
- Implements bulk prompting using for-loops across datasets.
- Example: Evaluate hundreds of articles against Google’s Helpful Content guidelines using a standard prompt template, then aggregate scores by article or question.
- SEO Crawling Reinvented:
- Uses spider or list mode to crawl domains.
- Captures structured data, links, images, headers, and redirects in a structured, auditable format.
- Offers tools for large-scale crawl comparison and content inventory analysis.
- Reproducibility is Key:
- Every workflow (crawl, URL analysis, prompt batch) can be repeated with consistency, enabling collaboration and longitudinal analysis.
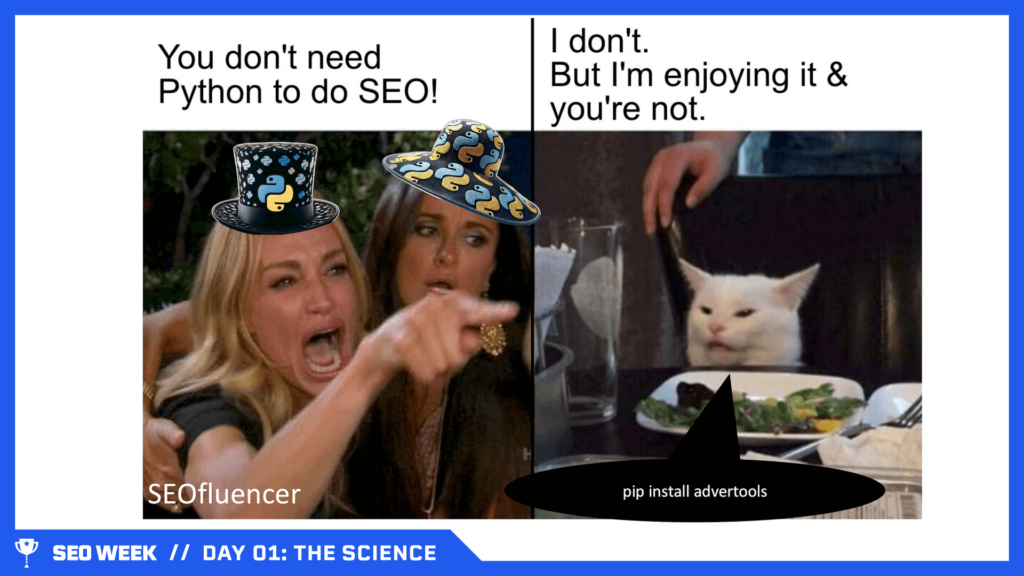
Important Takeaway
To scale modern SEO, you need to think like a data scientist: treat your content and technical elements as structured data, automate workflows, and audit using programmable frameworks—not intuition or guesswork.
Mini Glossary
- DataFrame: A table-like data structure used in Python (via pandas) to organize and manipulate data.
- robots.txt: A file on websites that tells search engines which pages they’re allowed to crawl.
- Prompt Template: A reusable LLM input format with placeholders that can be filled in with dynamic values.
- Bulk Prompting: Running a prompt template across multiple data rows, often using loops.
- .jsonl (JSON Lines): A file format where each line is a separate JSON object—ideal for storing crawl data.
- Structured Data: Metadata that helps search engines understand page content (e.g. schema.org, OpenGraph).
- Jupyter Notebook: An interactive Python environment used to write and run code, visualize data, and document workflows.
By offering both granular control and scalable workflows, advertools showcases the craftsmanship of engineering through a modular system that integrates structured data, generative AI, and methodical content evaluation—all in a transparent and auditable environment.
Hybrid Engine Optimization: A Crawler Driven Approach to Maximizing Search & AI Visibility
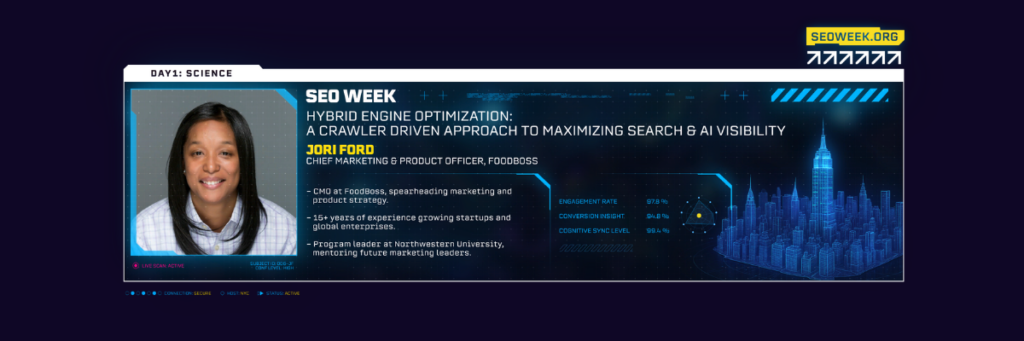
Hybrid Engine Optimization is the new mandate – Jori Ford, Chief Marketing & Product Officer at FoodBoss, dubbed the “Crawler Queen,” laid out how to audit crawl behavior, track AI agents, and structure content that gets surfaced by LLMs.
We used to think of the journey as very linear through a funnel. It’s changing – the journey is cyclical, never-ending. Search visibility now depends on being found by both traditional search engines and AI platforms.
“It’s about presence in the AI summary. If you’re not part of the journey, if you’re not part of the conversation, you’re not there.”
- Jori Ford
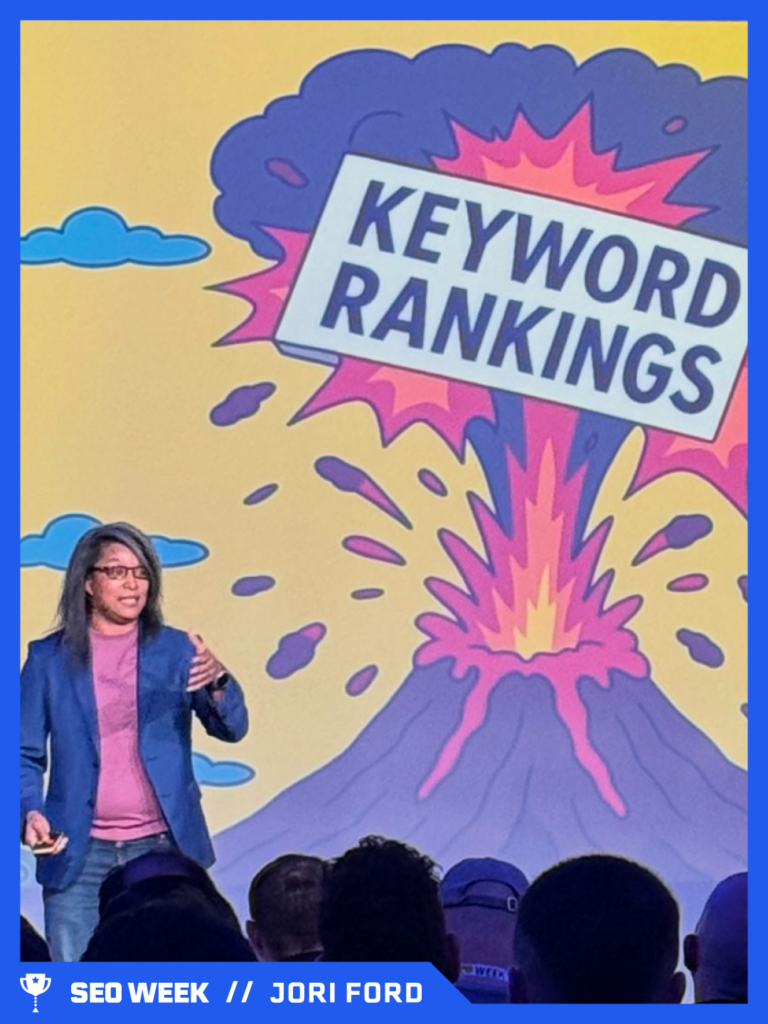
Key points
- Presence, Not Just Position: Ranking isn’t enough. With AI-generated answers replacing blue links, your content must be included in summaries from platforms like ChatGPT, Perplexity, and Google’s AI Overviews.
- Crawl Logs Are Your Source of Truth: Bot traffic shows what matters to machines, not just users. Parsing access logs can reveal:
- Which AI bots (like GPTBot, PerplexityBot) are crawling you
- Which pages they prioritize
- Where bots show up but clicks don’t follow
- Actionable tip: Request raw access logs from your dev team. Look for User-Agent strings to isolate AI bots, then prioritize high-crawl, low-click pages for optimization.
- Track AI Bots Using GTM and GA4: You can track AI crawlers like human visitors. Use Google Tag Manager to detect bot user agents and log them in GA4 as events. This helps identify overlooked content or missed opportunities.
- Example: If PerplexityBot hits your product comparison page frequently, but the page gets little organic traffic, revise it with clearer summaries or FAQs.
- Train the AI on Your Business: LLMs index based on what’s clear, structured, and learnable. You need to make your content extractable.
- How to do it:
- Place product definitions and brand messaging high on the page
- Add FAQ sections and glossary-style content
- Use internal links with brand terms
- Submit to Perplexity’s Pro knowledge base
- Ensure sitemap and robots.txt allow crawl access to key content
- Build “Answer Assets”: LLMs don’t index full pages. They extract fragments. Content should be built for retrieval.
- Structure for AI: Lead with the answer.
- Support with examples or data
- Use bullet points, headers, and anchors
- Optimize meta and OpenGraph for clarity
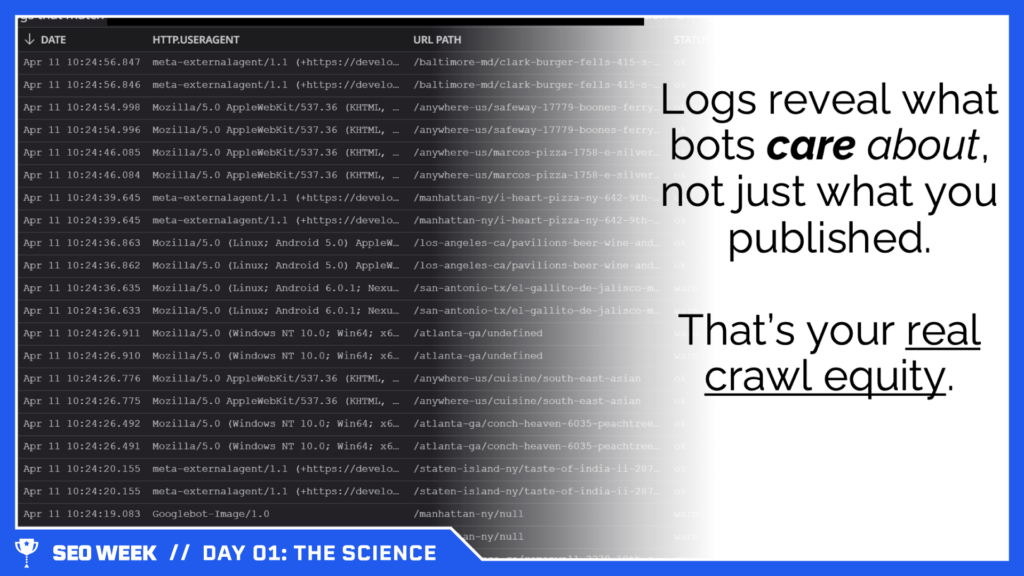
Important Takeaway
If your content isn’t structured for AI bots and summarized in ways they can retrieve, you’re invisible where decisions happen. Hybrid optimization means serving both humans and machines with equal precision.
Mini Glossary
- Access logs: A log file that records all events related to client applications and user access to a resource on a computer.
- User-Agent string: A characteristic string included in the HTTP request header that helps servers and network peers identify the application, operating system, vendor, and/or version of the requesting user agent.
- Google Tag Manager (GTM): A tag management system that allows you to set up and manage tags on your site without changing your website’s code.
- Google Analytics 4 (GA4): The newest version of Google’s web analytics service, designed to track user behavior and interactions across both websites and app.
- OpenGraph: An HTML markup used by social networks to display shared content.
The Hype, the Hubris, and the Hard Lessons of AI Development
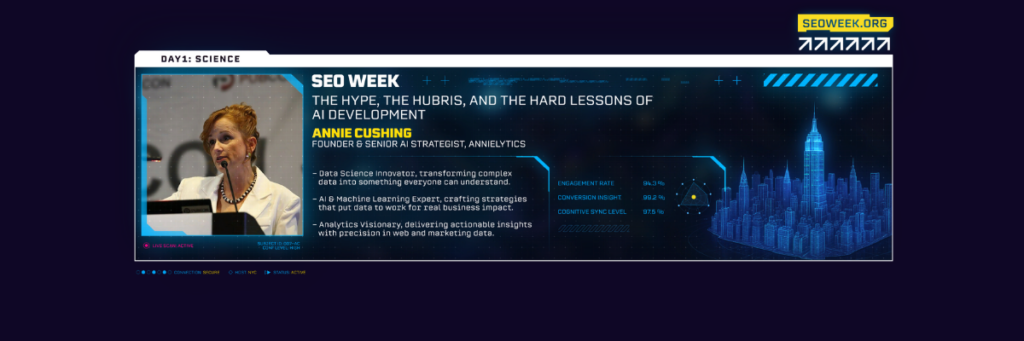
Founder & Senior AI Strategist at Annielytics Annie Cushing delivered a sharp critique of today’s AI ecosystem, calling out its overhyped narratives, the blind adoption of expensive foundation models, and a widespread lack of transparency in model evaluation.
Drawing from her hands-on experience building apps to help organizations choose the right AI tools, she exposed how misleading benchmarks, self-reported results, and misuse of generative AI can cost companies millions – and yield poor results.
“Imagine if we let students self-report SAT results instead of having a standardized process.”
- Annie Cushing
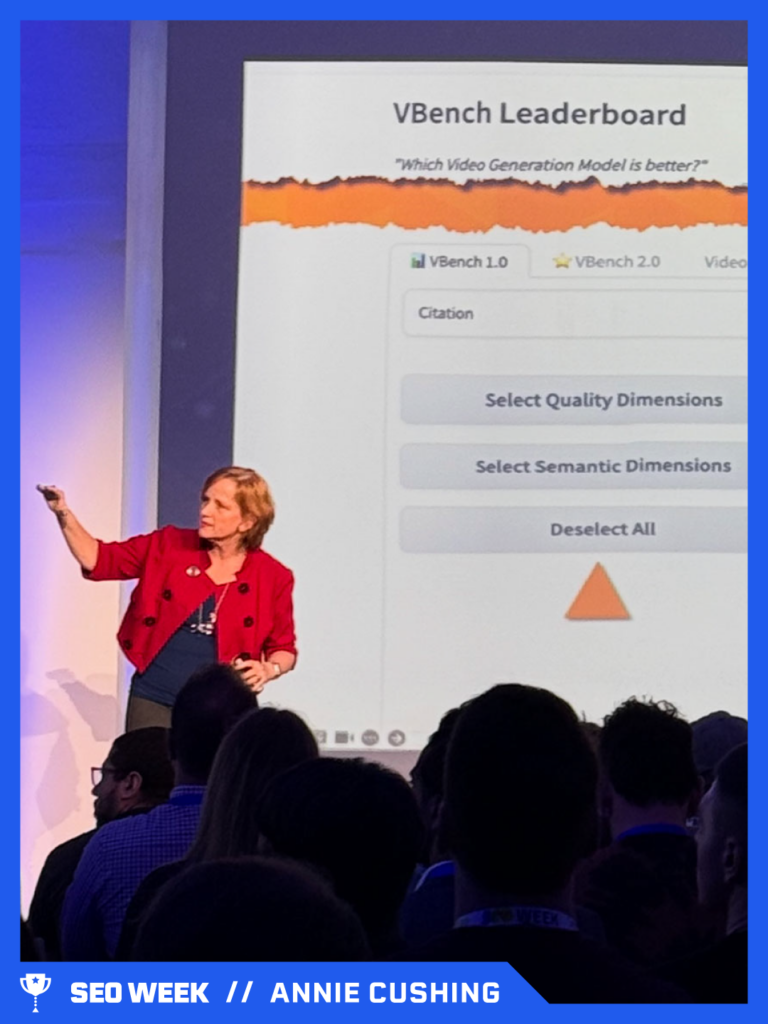
Key points
- AI Has Transformative Potential—but Strategy Is Lacking: Cushing looked at real-world use cases of AI in cancer detection, marine wildlife protection, and indigenous language preservation…but she stressed that many organizations adopt AI tools without a clear strategy or understanding of which models are suited for which tasks.
- Most AI Leaderboards Are Unreliable and Misleading: AI model performance dashboards (called leaderboards) often rely on vague filters, ambiguous scoring dimensions, or self-reported data from the model creators themselves. This lack of transparency leads to poor decision-making and inflated expectations.
- AI Strategy App: Annie built a free app to simplify leaderboard analysis by letting users select tasks and benchmarks, then visualize results in a graph with clear tooltips and filters.
- Foundation Models Are Often Overused and Overpriced: Many companies over-invest in expensive, general-purpose models like GPT-4 when simpler, more accurate, and cheaper models (or traditional statistical tools) would suffice.
- For example, she helped a dermatologist develop a classification model using a convolutional neural network (CNN) instead of a hallucination-prone foundation model.
- This resulted in better accuracy and lower cost.
- There Are Often Better Non-AI Alternatives: Use cases like tracking state education standards or analyzing customer churn are often better handled by traditional APIs or statistical models. Generative AI should not be a default solution for every task.
- The Industry Lacks Accountability for AI Performance Claims: Many AI companies, including major players like OpenAI and xAI, make unverified performance claims based on internal or public validation sets, bypassing rigorous, standardized evaluations.
- In one example, xAI claimed Grok 3 scored 93.3% on a math test a week after its answers were published.
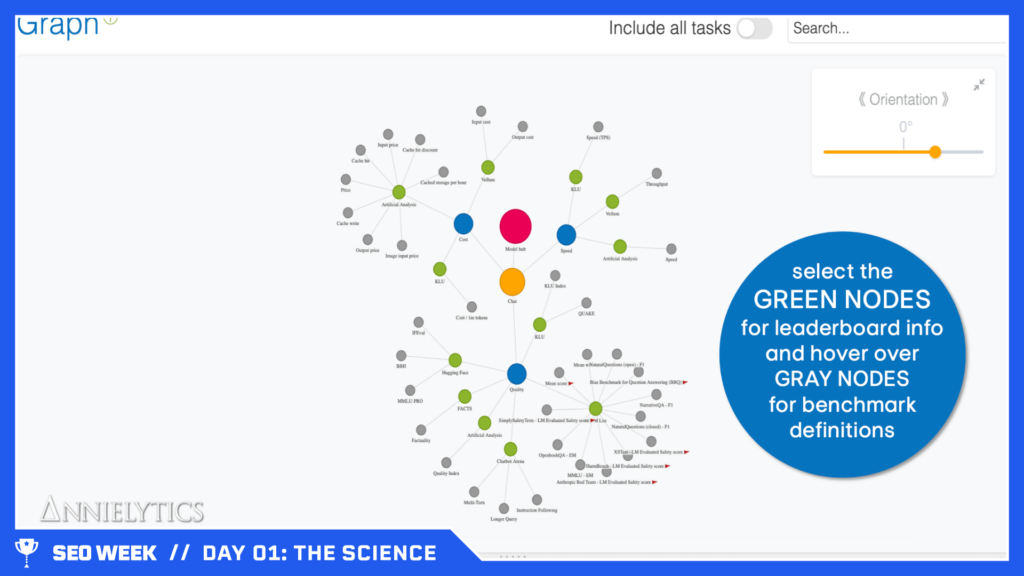
Important Takeaway
Most AI tools are not as reliable, cost-effective, or generalizable as vendors claim. Businesses must stop treating AI as a magic bullet and start treating model selection as a strategic, evidence-driven process.
Building internal tools that surface true performance data, like Annie’s benchmarking app, can reduce risk and cost dramatically.
Mini Glossary
- Foundation Model: A large-scale AI model trained on broad data and used for a wide range of tasks (e.g., GPT-4).
- Leaderboard: A public benchmark that ranks AI models based on standardized evaluation tasks.
- Model Contamination: When a model has seen the test data during training or validation, skewing results.
- CNN (Convolutional Neural Network): A type of deep learning model effective for image classification tasks.
- Validation Set: A portion of data used to tune AI models—often public and prone to misuse in self-reporting.
- Hallucination: When an AI model generates incorrect or fabricated information.
Annie cautions against overhyping unverified AI achievements, pointing to examples of dubious testing claims, and reminds us that we are still far from true AGI.
Benchmarking Brand Discoverability: LLMs vs. Traditional Search
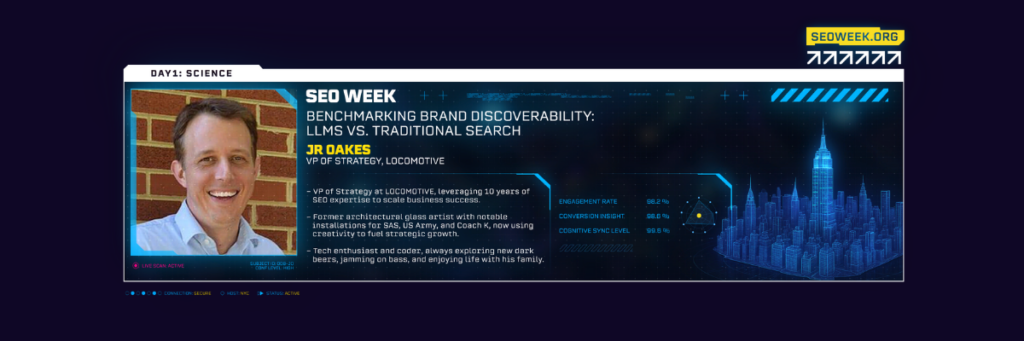
VP of Strategy at LOCOMOTIVE JR Oakes’ SEO Week talk presented a practical benchmarking experiment comparing how well traditional search and LLMs surface B2B SaaS brands in response to commercial queries.
Using a structured dataset from G2.com, Ahrefs, and a modifier library of 63 B2B SaaS qualifiers (e.g. “affordable”, “enterprise”), his team analyzed how different platforms – Google Search, Google AI Overviews, OpenAI, and Claude – recall and rank brands.
The core question: where are customers actually discovering brands now, and how does that vary by model?
“Agents don’t care about your affiliate commission.”
- JR Oakes
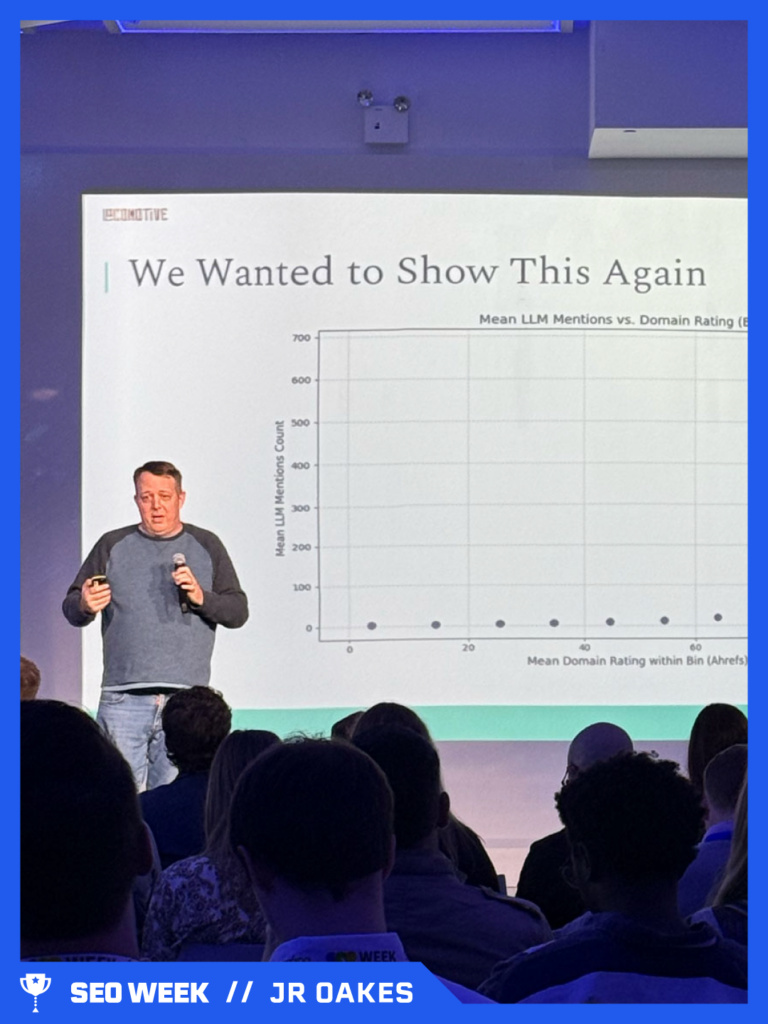
Key points
- Modifier-Based Discoverability: By pairing industry terms with specific search modifiers (e.g. “best CRM for startups”), they revealed how modifiers influence which brands appear. Google Organic returned the most unique brands per query, but LLMs like Claude and GPT-3.5 increasingly surface smaller and niche players.
- Model Behavior is Not Uniform: Each LLM had distinct recall tendencies. OpenAI and Anthropic differed significantly from Google AI Overviews. This inconsistency across models presents a clear opportunity to tailor content for each platform individually.
- Authority Still Matters: Brands with strong SEO fundamentals (e.g. high domain authority, strong content) also performed better in LLM outputs. Traditional SEO signals remain highly correlated with brand visibility across models.
- Geographic Biases Exist: LLMs are still largely US-centric, with a notable overrepresentation of California-based companies. Google performs better in surfacing newer or international brands due to fresher indexing and broader data coverage.
- Social Media ≠ Visibility Driver: Unlike Google, LLMs didn’t show meaningful preference for companies with large social media footprints, indicating a shift away from social signals in generative search visibility.
- LLM Lag on Freshness: Language models lag behind traditional search when it comes to surfacing newly launched companies or responding to fresh trends (known as QDF—Query Deserves Freshness).
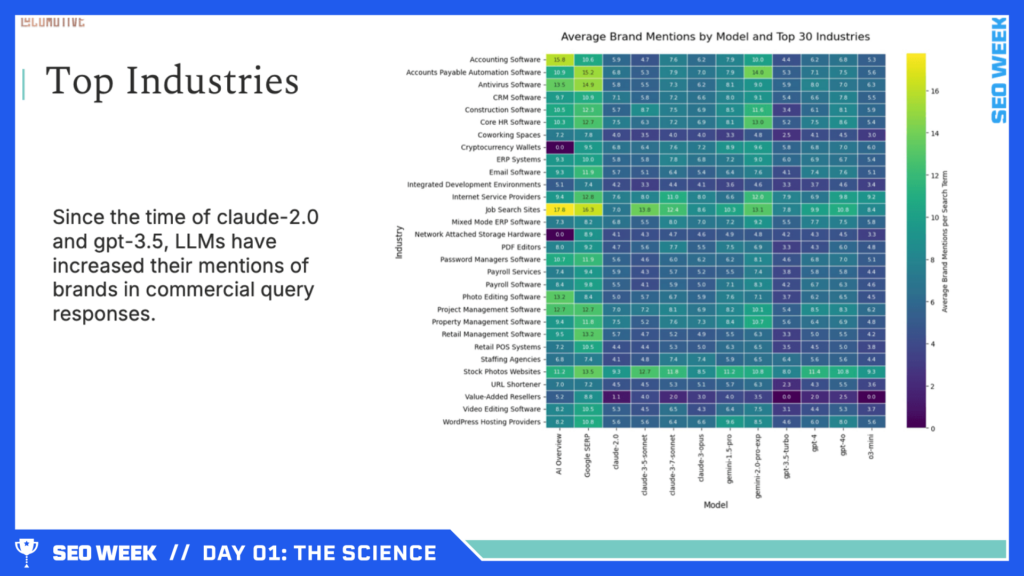
Important Takeaway
Success in LLM-driven discovery is not accidental. Brands need to apply SEO principles while adapting to platform-specific quirks. With modifier-rich queries becoming the norm, there’s strategic value in benchmarking brand presence across traditional and AI-powered search environments to uncover where your discoverability gaps lie.
Mini Glossary
- Modifier: A descriptive keyword added to a core term to refine intent (e.g. “cheap”, “secure”, “AI-powered”).
- QDF (Query Deserves Freshness): A search concept where newer content is prioritized when recent information is more relevant.
- Domain Authority: A metric indicating how likely a website is to rank in search engines based on backlink profile and trustworthiness.
As AI SEO grows, traditional SEO practices only partially transfer, and brands must adapt. The more authority you have in search engines, the more presence you have online, the more brand authority you have overall.
Frontier SEO Research and Data Science
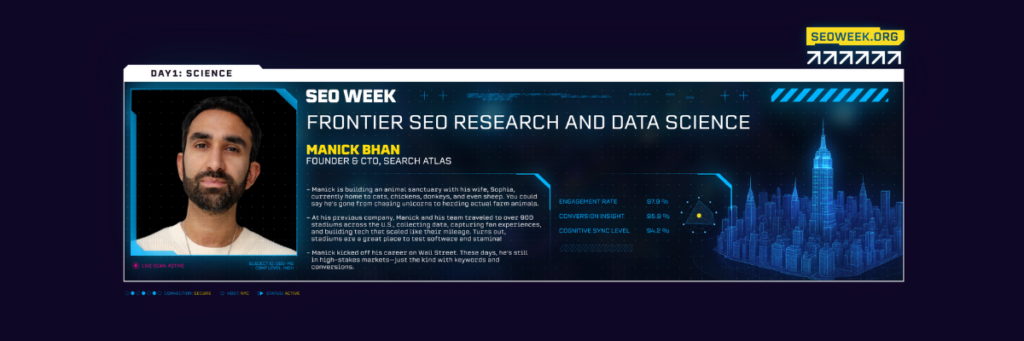
Manick Bhan, founder at Search Atlas, LinkGraph, and SEOTheory, emphasized the need for a scientific, collaborative approach to SEO, focusing heavily on data-driven techniques to decode Google’s algorithm and quantify website relevance.
He introduced practical tools and methodologies to measure and improve topical relevance and provided insights from correlation studies.
“We have to collaborate, we have to work together, we have to share our research and knowledge to advance the industry forward.”
- Manick Bhan
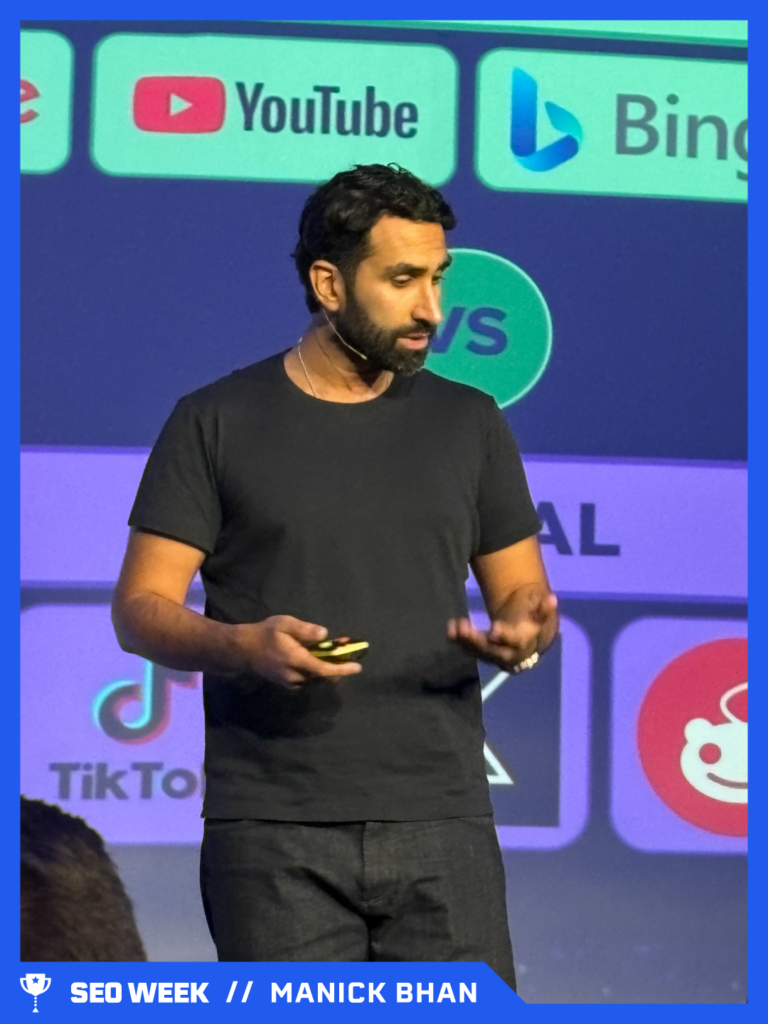
Key points
- Collaborative Competition: Instead of competing for individual keywords, SEO professionals should collaborate against Google’s subjective and rapidly updating algorithm, enhancing shared industry knowledge.
- Semantic Distance Analysis: Manick presented methods to visualize and quantify website content relevance through embeddings, enabling the identification and removal of irrelevant content.
- Topical Relevance Benchmarking: Using comparative coverage and correlation studies, Manick’s team determined that while domain strength remains highly influential, topical relevance significantly correlates with rankings, especially when dominating a specific niche.
- Local SEO Findings: Proximity, keyword-rich reviews, and business categories strongly influence local SEO rankings. Features like customer Q&A sections also notably affect local visibility.
- Crawl Behavior Insights: Site characteristics like daily crawl frequency, response time, and page size directly correlate with traffic and search visibility, highlighting the importance of technical site health.
- Content Semantics and Scholar Scores: Higher-quality, well-aligned content that scores well semantically tends to rank better, underscoring the continuing importance of deep, user-focused content.
- Authority Metrics and Intent Types: Correlations between authority signals and rankings remain consistent across different user intents, except navigational queries, highlighting nuances in SEO strategy.
- LLM Integration: Manick discussed the importance of optimizing content visibility within LLMs, suggesting methods like inclusion in datasets like Common Crawl to boost exposure in AI-generated responses.
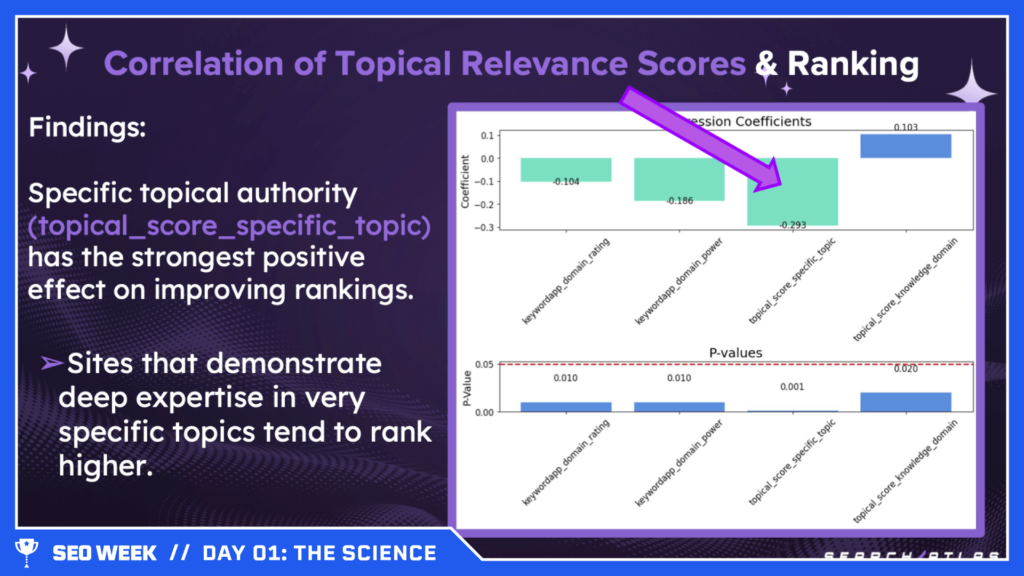
Important Takeaway
SEO professionals should prioritize deep, topic-specific content and technical site quality, collaborate more openly with industry peers, and strategically position their content to appear prominently in both traditional search results and emerging AI-driven platforms.
Mini Glossary
- Semantic Distance: Measuring how conceptually similar or different content is by mapping words or content into mathematical space.
- Embeddings: Representing words or content numerically, allowing comparison of their relationships or meanings.
- Topical Relevance: The depth and accuracy of website content coverage about a specific topic.
- Scholar Score: A metric assessing content quality, often tied to semantic richness and authority.
- Common Crawl: A large dataset containing snapshots of billions of web pages, widely used to train AI models like ChatGPT.
The field is shifting from a competitive to a collaborative mindset, and tools like Manick’s free GitHub project, Patent Brain, aim to visualize and quantify topical relevance.
Ultimately, advancing in SEO now means understanding search science, embracing automation, and crafting content that aligns tightly with both user intent and algorithmic logic.
Introducing: AX (Agent Experience)
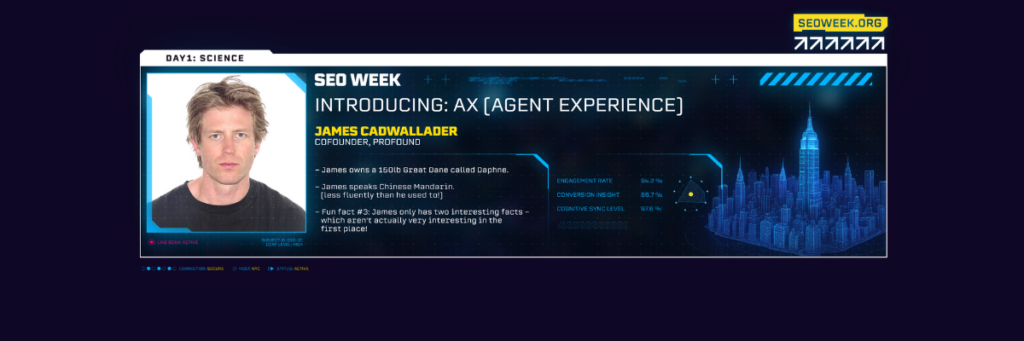
Profound Co-founder James Cadwallader introduced the concept of Agent Experience (AX), highlighting a shift towards an agent-driven internet, where AI agents interact with websites on behalf of users, rather than users directly visiting sites.
This marks a departure from traditional user experiences to a new model where AI agents interpret and present information autonomously – it’s the end of the traditional two-sided internet, where users manually browsed websites. We’re headed into a future where agents retrieve, interpret, and summarize information on behalf of users, often without them ever clicking through.
“We’re at the inflection point where humans no longer need to visit websites.”
- James Cadwallader
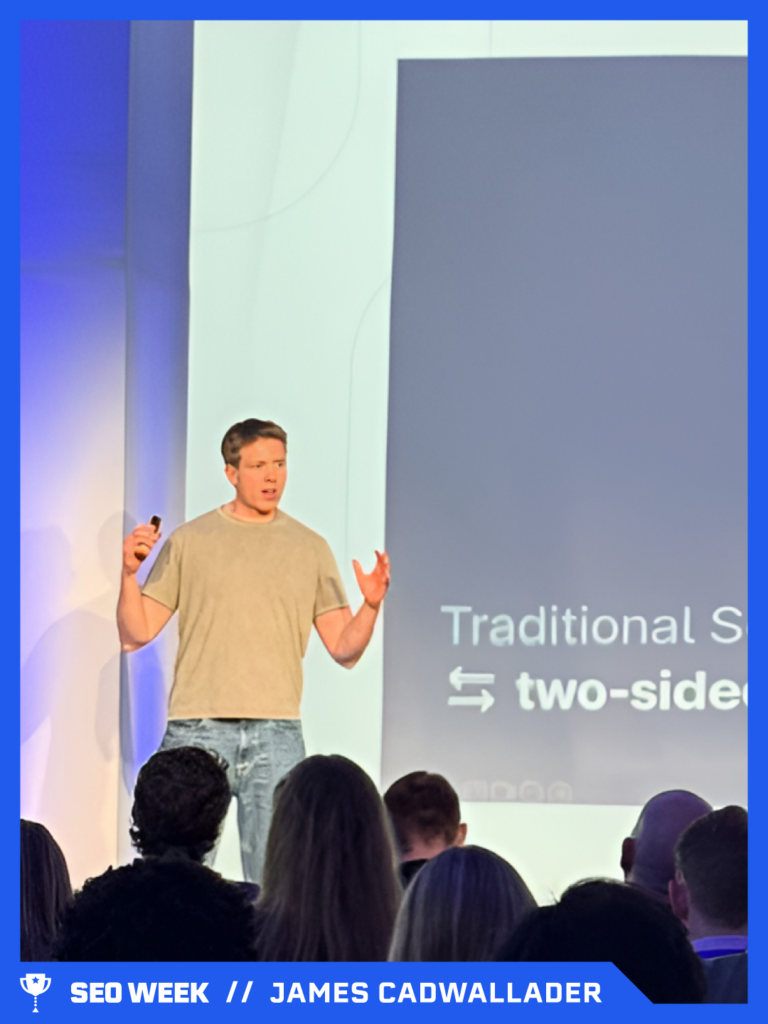
Key points
- Agent-Driven Internet: AI agents will become the primary consumers of online content, shifting the focus from direct user interactions to agent-mediated experiences.
- AX vs UX: AX involves designing digital environments specifically for AI agents, ensuring content is easily understood, actionable, and aligned with AI behavior.
- Commerce Evolution: Agents will autonomously make shopping decisions, potentially earning affiliate fees. An Adobe study found 92% of users felt AI improved their shopping, and 87% trusted AI for complex purchases.
- AI Personalization: Brands must prioritize understanding how agents personalize interactions, leveraging AI memory features such as OpenAI’s dynamically updating “Dreams,” which enhance interaction personalization over time.
- Advertising Shift: Advertising may evolve to directly target AI agents rather than consumers, potentially embedding ads in AI-generated responses.
- Optimizing for AI: AI agents prioritize structured data, semantic URLs, and metadata. Traffic, backlinks, or JavaScript interactions are less relevant to agents than clear, structured content.
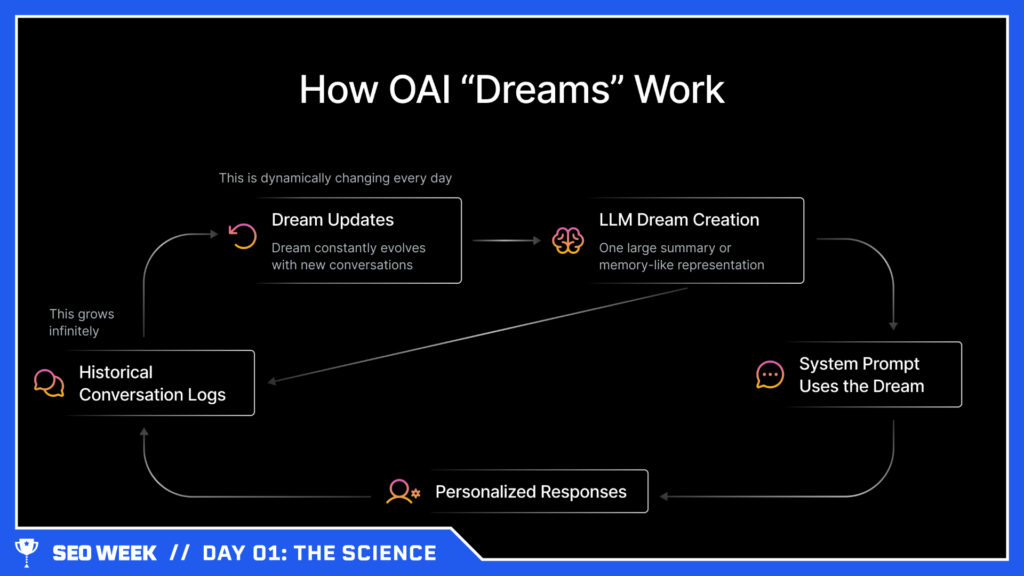
Important Takeaway
As AI agents become central to online interaction, marketers and SEO professionals must pivot to AX strategies, focusing on AI agent behaviors to maintain brand visibility and relevance.
Mini Glossary
- AX (Agent Experience): Design approach focused on optimizing digital environments specifically for AI agent interactions.
- Semantic URLs: URLs structured clearly with meaningful descriptions, enhancing AI agent confidence in content relevance.
- OpenAI Dreams: Dynamic, memory-based contexts within AI that evolve based on past interactions, improving personalization.
- LLMS.txt: Hypothetical structured data format optimized for AI agent consumption.
As platforms like ChatGPT evolve into answer engines that might even host ads, visibility will depend on how well a brand’s content is aligned with agent behavior.
This paradigm shift redefines SEO, transforming it from a traffic-driven function into a discipline centered on data precision, agent targeting, and machine-first strategy, presenting a once-in-a-century opportunity to shape the future of digital engagement.
Welcome Soiree Sponsored by Lastmile Retail
After a full day of brain-tingling presentations, Day 1 of SEO Week wrapped up with the perfect vibe-reset: the Opening Soirée.
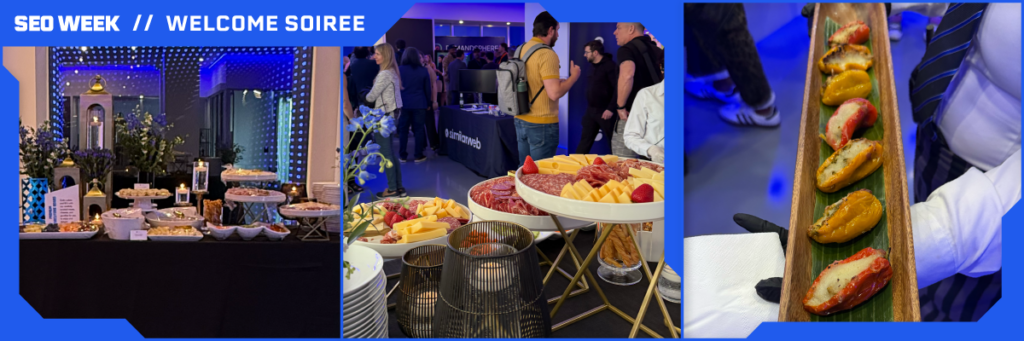
Held right in the chic, modern lobby of LAVAN, the event space transformed into a buzzing social scene complete with a bar, passed hors d’oeuvres, and a charcuterie-style buffet that had everyone hovering with plates in hand.
It was the perfect blend of smart and social – attendees, speakers, and the (pretty excited!) iPullRank crew mixed and mingled, trading takeaways from the sessions, sharing laughs, and making new connections. Whether you were deep in a convo about vector embeddings or just vibing over a glass of wine, it was the ideal way to cap off a powerful first day.
Stay Tuned - Next Week We’ll Review Day 2: The Psychology of SEO
And that was a wrap on Day 1 of SEO Week: The Science. What a ride – we laughed, we learned, we got our minds blown by vector math, and we crushed tiny fancy snacks like pros. From game-changing insights on the future of search to nerdery over cocktails, it was the kind of day that reminds you why SEO is anything but boring.
Huge shoutout to everyone who came through, shared ideas, asked questions, and made the Opening Soirée a vibe. This was just the beginning – stay tuned for next week’s post on Day 2: The Psychology. We’re keeping the momentum (and the vibe) going!